Model-Agnostic Meta-Learning for Fast Adaptation of Deep Networks
Introduction & Background
Learning Modular Policies from Sketches
Experiments
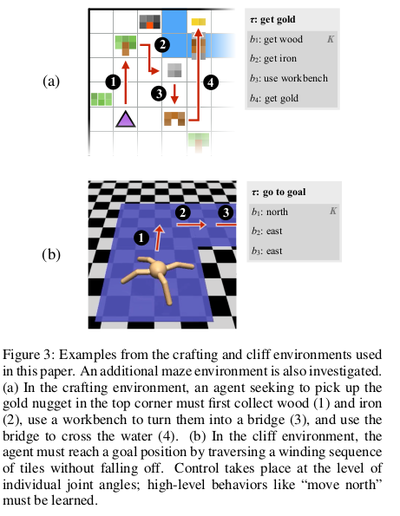
This paper considers three families of tasks: a 2-D Minecraft-inspired crafting game (Figure 3a), in which the agent must acquire particular resources by finding raw ingredients, combining them together in the correct order, and in some cases building intermediate tools that enable the agent to alter the environment itself; a 2-D maze navigation task that requires the agent to collect keys and open doors, and a 3-D locomotion task (Figure 3b) in which a quadrupedal robot must actuate its joints to traverse a narrow winding cliff.
In all tasks, the agent receives a reward only after the final goal is accomplished. For the most challenging tasks, involving sequences of four or five high-level actions, a task-specific agent initially following a random policy essentially never discovers the reward signal, so these tasks cannot be solved without considering their hierarchical structure. These environments involve various kinds of challenging low-level control: agents must learn to avoid obstacles, interact with various kinds of objects, and relate fine-grained joint activation to high-level locomotion goals.
Implementation
In all of the experiments, each subpolicy is implemented as a neural network with ReLU nonlinearities and a hidden layer with 128 hidden units. Each critic is a linear function of the current state. Each subpolicy network receives as input a set of features describing the current state of the environment, and outputs a distribution over actions. The agent acts at every timestep by sampling from this distribution. The gradient steps given in lines 8 and 9 of Algorithm 1 are implemented using RMSPROP with a step size of 0.001 and gradient clipping to a unit norm. They take the batch size D in Algorithm 1 to be 2000, and set $\gamma$= 0.9 in both environments. For curriculum learning, the improvement threshold $r_{good}$ is 0.8.
Environments
The environment in Figure 3a is inspired by the popular game Minecraft, but is implemented in a discrete 2-D world. The agent interacts with objects in the environment by executing a special USE action when it faces them. Picking up raw materials initially scattered randomly around the environment adds to an inventory. Interacting with different crafting stations causes objects in the agent’s inventory to be combined or transformed. Each task in this game corresponds to some crafted object the agent must produce; the most complicated goals require the agent to also craft intermediate ingredients, and in some cases build tools (like a pickaxe and a bridge) to reach ingredients located in initially inaccessible regions of the world.
The maze environment is very similar to “light world” described by [4]. The agent is placed in a discrete world consisting of a series of rooms, some of which are connected by doors. The agent needs to first pick up a key to open them. For our experiments, each task corresponds to a goal room that the agent must reach through a sequence of intermediate rooms. The agent senses the distance to keys, closed doors, and open doors in each direction. Sketches specify a particular sequence of directions for the agent to traverse between rooms to reach the goal. The sketch always corresponds to a viable traversal from the start to the goal position, but other (possibly shorter) traversals may also exist.
The cliff environment (Figure 3b) proves the effectiveness of the approach in a high-dimensional continuous control environment where a quadrupedal robot [5] is placed on a variable-length winding path, and must navigate to the end without falling off. This is a challenging RL problem since the walker must learn the low-level walking skill before it can make any progress. The agent receives a small reward for making progress toward the goal, and a large positive reward for reaching the goal square, with a negative reward for falling off the path.
Multitask Learning
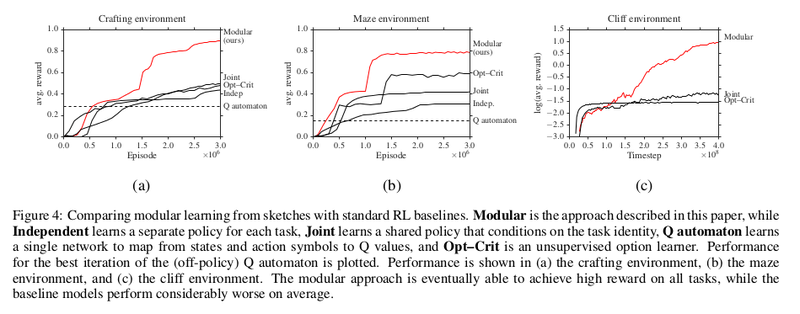
The primary experimental question in this paper is whether the extra structure provided by policy sketches alone is enough to enable fast learning of coupled policies across tasks. The aim is to explore the differences between the approach described and relevant prior work that performs either unsupervised or weakly supervised multitask learning of hierarchical policy structure. Specifically,they compare their modular approach to:
- Structured hierarchical reinforcement learners:
- the fully unsupervised option–critic algorithm of Bacon & Precup[1]
- a Q automaton that attempts to explicitly represent the Q function for each task / subtask combination (essentially a HAM [8] with a deep state abstraction function)
- Alternative ways of incorporating sketch data into standard policy gradient methods:
- learning an independent policy for each task
- learning a joint policy across all tasks, conditioning directly on both environment features and a representation of the complete sketch
The joint and independent models performed best when trained with the same curriculum described in Section 3.3, while the option–critic model performed best with a length–weighted curriculum that has access to all tasks from the beginning of training.
Learning curves for baselines and the modular model are shown in Figure 4. It can be seen that in all environments, our approach substantially outperforms the baselines: it induces policies with substantially higher average reward and converges more quickly than the policy gradient baselines. It can further be seen in Figure 4c that after policies have been learned on simple tasks, the model is able to rapidly adapt to more complex ones, even when the longer tasks involve high-level actions not required for any of the short tasks.
Ablations
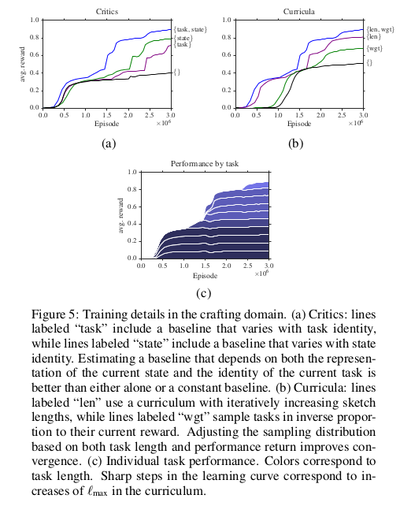
In addition to the overall modular parameter tying structure induced by sketches, the other critical component of the training procedure is the decoupled critic and the curriculum. The next experiments investigate the extent to which these are necessary for good performance.
To evaluate the the critic, consider three ablations:
- Removing the dependence of the model on the environment state, in which case the baseline is a single scalar per task
- Removing the dependence of the model on the task, in which case the baseline is a conventional generalised advantage estimator
- Removing both, in which case the baseline is a single scalar, as in a vanilla policy gradient approach.
Results are shown in Figure 5a. Introducing both state and task dependence into the baseline leads to faster convergence of the model: the approach with a constant baseline achieves less than half the overall performance of the full critic after 3 million episodes. Introducing task and state dependence independently improve this performance; combining them gives the best result.
Zero-shot and Adaptation Learning
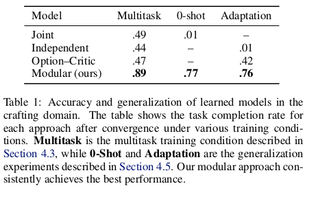
In the final experiments, the authors test the model’s ability to generalize beyond the standard training condition. Consider two tests of generalization: a zero-shot setting, in which the model is provided a sketch for the new task and must immediately achieve good performance, and a adaptation setting, in which no sketch is provided leaving the model to learn the form of a suitable sketch via interaction in the new task.They hold out two length-four tasks from the full inventory used in Section 4.3, and train on the remaining tasks. For zero-shot experiments, the concatenated policy is formed to describe the sketches of the held-out tasks, and repeatedly executing this policy (without learning) in order to obtain an estimate of its effectiveness. For adaptation experiments, consider ordinary RL over high-level actions B rather than low-level actions A, implementing the high-level learner with the same agent architecture as described in Section 3.1. Results are shown in Table 1. The held-out tasks are sufficiently challenging that the baselines are unable to obtain more than negligible reward: in particular, the joint model overfits to the training tasks and cannot generalize to new sketches, while the independent model cannot discover enough of a reward signal to learn in the adaptation setting. The modular model does comparatively well: individual subpolicies succeed in novel zero-shot configurations (suggesting that they have in fact discovered the behavior suggested by the semantics of the sketch) and provide a suitable basis for adaptive discovery of new high-level policies.
Conclusion & Critique
The paper's contributions are:
- A general paradigm for multitask, hierarchical, deep reinforcement learning guided by abstract sketches of task-specific policies.
- A concrete recipe for learning from these sketches, built on a general family of modular deep policy representations and a multitask actor–critic training objective.
They have described an approach for multitask learning of deep multitask policies guided by symbolic policy sketches. By associating each symbol appearing in a sketch with a modular neural subpolicy, they have shown that it is possible to build agents that share behavior across tasks in order to achieve success in tasks with sparse and delayed rewards. This process induces an inventory of reusable and interpretable subpolicies which can be employed for zero-shot generalisation when further sketches are available, and hierarchical reinforcement learning when they are not.
References
[1] Bacon, Pierre-Luc and Precup, Doina. The option-critic architecture. In NIPS Deep Reinforcement Learning Work-shop, 2015.
[2] Sutton, Richard S, Precup, Doina, and Singh, Satinder. Be-tween MDPs and semi-MDPs: A framework for tempo-ral abstraction in reinforcement learning. Artificial intel-ligence, 112(1):181–211, 1999.
[3] Stolle, Martin and Precup, Doina. Learning options in reinforcement learning. In International Symposium on Abstraction, Reformulation, and Approximation, pp. 212– 223. Springer, 2002.
[4] Konidaris, George and Barto, Andrew G. Building portable options: Skill transfer in reinforcement learning. In IJ-CAI, volume 7, pp. 895–900, 2007.
[5] Schulman, John, Moritz, Philipp, Levine, Sergey, Jordan, Michael, and Abbeel, Pieter. Trust region policy optimization. In International Conference on Machine Learning, 2015b.
[6] Greensmith, Evan, Bartlett, Peter L, and Baxter, Jonathan. Variance reduction techniques for gradient estimates in reinforcement learning. Journal of Machine Learning Research, 5(Nov):1471–1530, 2004.
[7] Andre, David and Russell, Stuart. Programmable reinforce-ment learning agents. In Advances in Neural Information Processing Systems, 2001.
[8] Andre, David and Russell, Stuart. State abstraction for pro-grammable reinforcement learning agents. In Proceedings of the Meeting of the Association for the Advance-ment of Artificial Intelligence, 2002.