Zero-Shot Visual Imitation: Difference between revisions
Line 28: | Line 28: | ||
In the above equation, <math display="inline">π</math> takes as input a pair of observations <math display="inline">(x_i, x_g)</math> and outputs the sequence of required actions <math display="inline">(\overrightarrow{a}_τ : a_1, a_2, ..., a_K)</math> to reach the goal observation <math display="inline"> | In the above equation, <math display="inline">π</math> takes as input a pair of observations <math display="inline">(x_i, x_g)</math> and outputs the sequence of required actions <math display="inline">(\overrightarrow{a}_τ : a_1, a_2, ..., a_K)</math> to reach the goal observation <math display="inline">x_g</math> from the current observation <math display="inline">x_i</math>. The states <math display="inline">x_i</math> and <math display="inline">x_g</math> are sampled from <math display="inline">S</math> | ||
Revision as of 12:31, 5 November 2018
This page contains a summary of the paper "Zero-Shot Visual Imitation" by Pathak, D., Mahmoudieh, P., Luo, G., Agrawal, P. et al. It was published at the International Conference on Learning Representations (ICLR) in 2018.
Introduction and Paper Overview
The dominant paradigm for imitation learning relies on strong supervision of expert actions to learn both what and how to imitate for a certain task. For example, in the robotics field, Learning from Demonstration (LfD) (Argall et al., 2009; Ng & Russell, 2000; Pomerleau, 1989; Schaal, 1999) requires an expert to manually move robot joints (kinesthetic teaching) or teleoperate the robot to teach a desired task. The expert will, in general, provide multiple demonstrations of a specific task at training time which the agent will form into observation-action pairs to then distill into a policy for performing the task. In the case of demonstrations for a robot, this heavily supervised process is tedious and unsustainable especially looking at the fact that new tasks need a set of new demonstrations for the robot to learn from.
Observational Learning (Bandura & Walters, 1977), a term from the field of psychology, suggests a more general formulation where the expert communicates what needs to be done (as opposed to how something is to be done) by providing observations of the desired world states via video or sequential images. This is the proposition of the paper and while this is a harder learning problem, it is possibly more useful because the expert can now distill a large number of tasks easily (and quickly) to the agent.
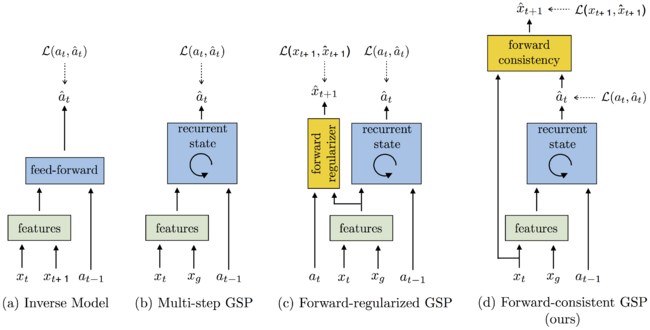
This paper follows (Agrawal et al., 2016; Levine et al., 2016; Pinto & Gupta, 2016) where an agent first explores the environment independently and then distills its observations into goal-directed skills. The word 'skill' is used to denote a function that predicts the sequence of actions to take the agent from the current observation to the goal. This function is what is known as a goal-conditioned skill policy (GSP) and it is learned by re-labeling states that the agent has visited as goals and the actions taken as prediction targets. During inference/prediction, the GSP recreates the task step-by-step given the goal observations from the demonstration.
A challenge of learning the GSP is that the distribution of trajectories from one state to another is multi-modal; that is, there are many possible ways of traversing from one state to another. This issue is addressed with the main contribution of this paper, the forward-consistent loss which essentially says that reaching the goal is more important than how it is reached. First, a forward model is learned that predicts the next observation from the given action and current observation. The difference in the output of the forward model for the GSP-selected action and the ground-truth next state is used to train the model. This forward-consistent loss has the effect of not inadvertently penalizing actions that are consistent with the ground-truth action but not exactly the same.
As a simple example to explain the forward-consistent loss, imagine a scenario where a robot must grab an object some distance ahead with an obstacle along the pathway. Now suppose that during demonstration the obstacle is avoided by going to the right and then grabbing the object while the agent during training decides to go left and then grab the object. The forward-consistent loss would characterize the action of the robot as consistent with the ground-truth action of the demonstrator and not penalize the robot for going left instead of right.
Of course, when introducing something like this forward-consistent loss, issues related to the number of steps needed to reach a certain goal become prevalent. To address this, the paper pairs the GSP with a goal recognizer that determines if the goal has been satisfied with respect to some metrics. Figure 1 shows various GSPs along with diagram d) showing the forward-consistent loss proposed in this paper.
The zero-shot imitator is tested on a Baxter robot performing tasks involving rope manipulation, a TurtleBot performing office navigation and navigation experiments in VizDoom. Positive results are shown for all three experiments leading to the conclusion that the forward-consistent GSP can be used to imitate a variety of tasks without making environmental or task-specific assumptions.
Learning to Imitate Without Expert Supervision
In this section (and the included subsections) the methods for learning the GSP, forward consistency loss and goal recognizer network are described.
Let [math]\displaystyle{ S : \{x_1, a_1, x_2, a_2, ..., x_T\} }[/math] be the sequence of observation-action pairs generated by the agent as it explores the environment using the policy [math]\displaystyle{ a = π_E(s) }[/math]. This exploration data is used to learn the GSP.
In the above equation, [math]\displaystyle{ π }[/math] takes as input a pair of observations [math]\displaystyle{ (x_i, x_g) }[/math] and outputs the sequence of required actions [math]\displaystyle{ (\overrightarrow{a}_τ : a_1, a_2, ..., a_K) }[/math] to reach the goal observation [math]\displaystyle{ x_g }[/math] from the current observation [math]\displaystyle{ x_i }[/math]. The states [math]\displaystyle{ x_i }[/math] and [math]\displaystyle{ x_g }[/math] are sampled from [math]\displaystyle{ S }[/math]