This Looks Like That: Deep Learning for Interpretable Image Recognition
Presented by
Nouha Chatti
Introduction
The motivation behind this paper is to introduce a new deep learning network architecture capable of reasoning in a humanly understandable way dealing with classification tasks. The idea is to perform these tasks by defining a form of interpretability when processing the images. The method suggested in this paper consists in dissecting parts of the input images and comparing them to prototypical parts of training images of a given class: Thus the expression this looks like that. In fact, this solution adds a transparency advantage to deep neural networks and allows the user to understand the actual process of decision making. It can intervene in many crucial problems that require understanding the actions that led to a particular output of the model. There are many fields that already rely on this case-based reasoning especially in the medical domain where diagnosis using X-ray scans is based on comparing these latter to other prototypical scans.
Previous Work
Network Architecture
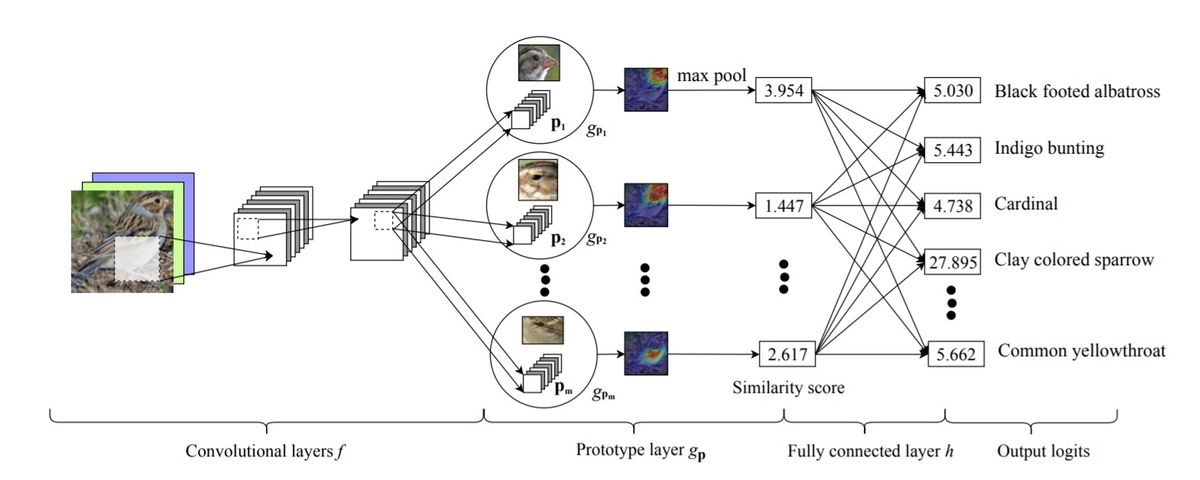