Describtion of Text Mining
Presented by
Yawen Wang, Danmeng Cui, Zijie Jiang, Mingkang Jiang, Haotian Ren, Haris Bin Zahid
Introduction
This paper focuses on the different text mining techniques and the applications of text mining in the healthcare and biomedical domain. The text mining field has been popular as a result of the amount of text data that is available in different forms. The text data is bound to grow even more in 2020, indicating a 50 times growth since 2010. Text is unstructured information, which is easy for humans to construct and understand but difficult for machines. Hence, there is a need to design algorithms to effectively process this avalanche of text. To further explore the text mining field, the related text mining approaches can be considered. The different text mining approaches relate to two main methods: knowledge delivery and traditional data mining methods.
The authors note that knowledge delivery methods involve the application of different steps to a specific data set to create specific patterns. Research in knowledge delivery methods has evolved over the years due to advances in hardware and software technology. On the other hand, data mining has experienced substantial development through the intersection of three fields: databases, machine learning, and statistics. As brought out by the authors, text mining approaches focus on the exploration of information from a specific text. The information explored is in the form of structured, semi-structured, and unstructured text. It is important to note that text mining covers different sets of algorithms and topics that include information retrieval. The topics and algorithms are used for analyzing different text forms.
Preprocessing
The authors introduce multiple methods of preprocessing text.
1. Tokenization
This process splits text into units of words of phrases known as tokens while removing unnecessary characters.
2. Filtering
Filtering is a process by which unnecessary words or characters are removed. Often these include punctuation, prepositions, and conjugations. The resulting corpus then contains words with maximal importance in distinguishing between classes.
3. Stemming
Stemming extracts the roots of words. It is a language dependent process. The goal of both stemming is to reduce inflectional and related (definition wise) forms of a word to a common base form. An example of this would be changing "am", "are", or "is" to "be".
Text Representation and Encoding
In this section of the paper, the authors explore the different ways in which the text can be represented on a large collection of documents. One common way of representing the documents is in the form of a bag of words. The bag of words considers the occurrences of different terms. In different text mining applications, documents are ranked and represented as vectors so as to display the significance of any word. The authors note that the three basic models used are vector space, inference network, and the probabilistic models. The vector space model is used to represent documents by converting them into vectors. In the model, a variable is used to represent each model to indicate the importance of the word in the document. The words are weighted using the TF-IDF scheme computed as
$$ q(w)=f_d(w)*log{\frac{|D|}{f_D(w)}} $$
In many text mining algorithms, one of the key components is preprocessing. Preprocessing consists of different tasks that include filtering, tokenization, stemming, and lemmatization. The first step is tokenization, where a character sequence is broken down into different words or phrases. After the breakdown, filtering is carried out to remove some words. The various word inflected forms are grouped together through lemmatization, and later, the derived roots of the derived words are obtained through stemming.
Classification
Classification in Text Mining aims to assigned predefined classes to text documents. For a set [math]\displaystyle{ \mathcal{D} = {d_1, d_2, ... d_n} }[/math] of documents, such that each [math]\displaystyle{ d_i }[/math] is mapped to a label [math]\displaystyle{ l_i }[/math] from the set [math]\displaystyle{ \mathcal{L} = {l_1, l_2, ... l_k} }[/math]. The goal is to find a classification model [math]\displaystyle{ f }[/math] such that: [math]\displaystyle{ \\ }[/math] $$ f: \mathcal{D} \rightarrow \mathcal{L} \quad \quad \quad f(\mathcal{d}) = \mathcal{l} $$ The author illustrates 4 different classifiers that are commonly used in text mining.
1. Naive Bayes Classifier
Bayes rule is used to classify new examples and select the class that has the generated result that occurs most often. Naive Bayes Classifier models the distribution of documents in each class using a probabilistic model assuming that the distribution of different terms is independent of each other. The models commonly used in this classifier tried to find the posterior probability of a class based on the distribution and assumes that the documents generated are based on a mixture model parameterized by [math]\displaystyle{ \theta }[/math] and compute the likelihood of a document using the sum of probabilities over all mixture component. In addition, the Naive Bayes Classifier can help get around the curse of dimensionality, which may arise with high-dimensional data, such as text.
2. Nearest Neighbour Classifier
Nearest Neighbour Classifier uses distance-based measures to perform the classification. The documents which belong to the same class are more likely "similar" or close to each other based on the similarity measure. The classification of the test documents is inferred from the class labels of similar documents in the training set.
3. Decision Tree Classifier
A hierarchical tree of the training instances, in which a condition on the attribute value is used to divide the data hierarchically. The decision tree recursively partitions the training data set into smaller subdivisions based on a set of tests defined at each node or branch. Each node of the tree is a test of some attribute of the training instance, and each branch descending from the node corresponds to one of the values of this attribute. The conditions on the nodes are commonly defined by the terms in the text documents.
4. Support Vector Machines
SVM is a form of Linear Classifiers which are models that makes a classification decision based on the value of the linear combinations of the documents features. The output of a linear predictor is defined to the [math]\displaystyle{ y=\vec{a} \cdot \vec{x} + b }[/math] where [math]\displaystyle{ \vec{x} }[/math] is the normalized document word frequency vector, [math]\displaystyle{ \vec{a} }[/math] is a vector of coefficient and [math]\displaystyle{ b }[/math] is a scalar. Support Vector Machines attempts to find a linear separators between various classes. An advantage of the SVM method is it is robust to high dimensionality.
Clustering
Clustering has been extensively studied in the context of the text as it has a wide range of applications such as visualization and document organization.
Clustering algorithms are used to group similar documents and thus aids in information retrieval. Text clustering can be in different levels of granularities, where clusters can be documents, paragraphs, sentences, or terms. Since text data has numerous distance characteristics that demand the design of text-specific algorithms for the task, using a binary vector to represent the text document is simply not enough. Here are some unique properties of text representation:
1. Text representation has a large dimensionality, in which the size of the vocabulary from which the documents are drawn is massive, but a document might only contain a small number of words.
2. The words in the documents are usually correlated with each other. Need to take the correlation into consideration when designing algorithms.
3. The number of words differs from one another of the document. Thus the document needs to be normalized first before the clustering process.
There are 3 most commonly used text clustering algorithms presented.
1. Hierarchical Clustering algorithms
Hierarchical Clustering algorithms builds a group of clusters that can be depicted as a hierarchy of clusters. The hierarchy can be constructed in top-down (divisive) or bottom-up (agglomeration). Hierarchical clustering algorithms are one of the Distanced-based clustering algorithms, i.e., using a similarity function to measure the closeness between text documents.
In the top-down approach, the algorithm begins with one cluster which includes all the documents. we recursively split this cluster into sub-clusters. Here is an example of a Hierarchical Clustering algorithm, the data is to be clustered by the euclidean distance. This method builds the hierarchy from the individual elements by progressively merging clusters. In our example, we have six elements {a} {b} {c} {d} {e} and {f}. The first step determines which elements to merge in a cluster by taking the two closest elements, according to the chosen distance.
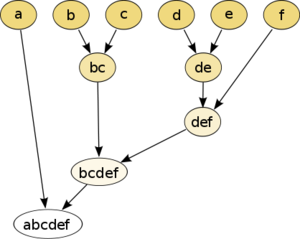
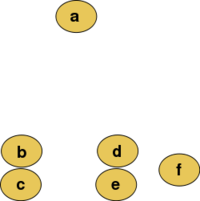
A main advantage of hierarchical clustering is that the algorithm only needs to be done once for any number of clusters (ie. if an individual wishes to use a different number of clusters than originally intended, they do not need to repeat the algorithm)
2. k-means Clustering
k-means clustering is a partitioning algorithm that partitions n documents in the context of text data into k clusters.
Input: Document D, similarity measure S, number k of cluster Output: Set of k clusters Select randomly k datapoints as starting centroids While not converged do Assign documents to the centroids based on the closest similarity Calculate the cluster centroids for all clusters return k clusters
The main disadvantage of k-means clustering is that it is indeed very sensitive to the initial choice of the number of k. Also, since the function is run until clusters converges, k-means clustering tends to take longer to perform than hierarchical clustering. On the other hand, advantages of k-means clustering are that it is simple to implement, the algorithm scales well to large datasets, and the results are easily interpretable.
3. Probabilistic Clustering and Topic Models
Topic modeling is one of the most popular probabilistic clustering algorithms in recent studies. The main idea is to create a *probabilistic generative model* for the corpus of text documents. In topic models, documents are a mixture of topics, where each topic represents a probability distribution over words.
There are two main topic models:
- Probabilistic Latent Semantic Analysis (pLSA)
- Latent Dirichlet Allocation (LDA)
The paper covers LDA in more detail. LDA is a state-of-the-art unsupervised algorithm for extracting topics from a collection of documents.
Given [math]\displaystyle{ \mathcal{D} = \{d_1, d_2, \cdots, d_{|\mathcal{D}|}\} }[/math] is the corpus and [math]\displaystyle{ \mathcal{V} = \{w_1, w_2, \cdots, w_{|\mathcal{V}|}\} }[/math] is the vocabulary of the corpus.
A topic is [math]\displaystyle{ z_j, 1 \leq j \leq K }[/math] is a multinomial probability distribution over [math]\displaystyle{ |\mathcal{V}| }[/math] words.
The distribution of word given document is:
[math]\displaystyle{ p(w_i|d) = \Sigma_{j=1}^K p(w_i|z_j)p(z_j|d) }[/math]
The LDA assumes the following generative process for the corpus of [math]\displaystyle{ \mathcal{D} }[/math]
- For each topic [math]\displaystyle{ k\in \{1,2,\cdots, K\} }[/math], sample a word distribution [math]\displaystyle{ \phi_k \sim Dir(\beta) }[/math]
- For each document [math]\displaystyle{ d \in \{1,2,\cdots,D\} }[/math]
- Sample a topic distribution [math]\displaystyle{ \theta_d \sim Dir(\alpha) }[/math]
- For each word [math]\displaystyle{ w_n, n \in \{1,2,\cdots,N\} }[/math] in document [math]\displaystyle{ d }[/math]
- Sample a topic [math]\displaystyle{ z_i \sim Mult(\theta_d) }[/math]
- Sample a word [math]\displaystyle{ w_n \sim Mult(\phi_{z_i}) }[/math]
In practice, LDA is often used as a module in more complicated models and has already been applied to a wide variety of domains. In addition, many variations of LDA has been created, including supervised LDA (sLDA) and hierarchical LDA (hLDA)
Information Extraction
Information Extraction (IE) is the process of extracting useful, structured information from unstructured or semi-structured text. It automatically extracts based on our command.
For example, from the sentence “XYZ company was founded by Peter in the year of 1950”, we can identify the following information:
Founderof(Peter, XYZ) Foundedin(1950, XYZ)
The author mentioned 4 parts that are important for Information Extraction
1. Namely Entity Recognition(NER)
This is the process of identifying real-world entity from free text, such as "Apple Inc.", "Donald Trump", "PlayStation 5" etc. Moreover, the task is to identify the category of these entities, such as "Apple Inc." is in the category of the company, "Donald Trump" is in the category of the USA president, and "PlayStation 5" is in the category of the entertainment system.
2. Hidden Markov Model
Since traditional probabilistic classification does not consider the predicted labels of neighbor words, we use the Hidden Markov Model when doing Information Extraction. This model is different because it considers the label of one word depends on the previous words that appeared.
3. Conditional Random Fields
This is a technique that is widely used in Information Extraction. The definition of it is related to graph theory. let G = (V, E) be a graph and Yv stands for the index of the vertices in G. Then (X, Y) is a conditional random field, when the random variables Yv, conditioned on X, obey Markov property with respect to the graph, and: p(Yv |X, Yw ,w , v) = p(Yv |X, Yw ,w ∼ v), where w ∼ v means w and v are neighbors in G.
4. Relation Extraction
This is a task of finding semantic relationships between word entities in text documents. Such as "Seth Curry" is the brother of "Stephen Curry", if there is a document including these two names, the task is to identify the relationship of these two entities.
Biomedical Application
Text mining has several applications in the domain of biomedical sciences. The explosion of academic literature in the field has made it quite hard for scientists to keep up with novel research. This is why text mining techniques are ever so important in making the knowledge digestible.
The text mining techniques are able to extract meaningful information from large data by making use of biomedical ontology, which is a compilation of a common set of terms used in an area of knowledge. The Unified Medical Language System (UMLS) is the most comprehensive such resource, consisting of definitions of biomedical jargon. Several information extraction algorithms rely on the ontology to perform tasks such as Named Entity Recognition (NER) and Relation Extraction.
NER involves locating and classifying biomedical entities into meaningful categories and assigning semantic representation to those entities. The NER methods can be broadly grouped into Dictionary-based, Rule-based and Statistical approaches. Relation extraction, on the other hand, is the process of determining relationships between the entities. This is accomplished mainly by identifying the correlation between entities through analyzing the frequency of terms, as well as rules defined by domain experts. Moreover, modern algorithms are also able to summarize large documents and answer natural language questions posed by humans.
Conclusion
This paper gave a holistic overview of the methods and applications of text mining, particularly its relevance in the biomedical domain. It highlights several popular algorithms and summarizes them along with their advantages, limitations and some potential situations where they could be used. Because of ever-growing data, for example, the very high volume of scientific literature being produced every year, the interest in this field is massive and is bound to grow in the future.
Critiques
This is a very detailed approach to introduce some different algorithms on text mining. Since many algorithms are given, it might be a good idea to compare their performances on text mining by training them on some text data and compare them to the former baselines, to see if there exists any improvement.
it is a detailed summary of the techniques used in text mining. It would be more helpful if some dataset can be included for training and testing. The algorithms were grouped by different topics so that different datasets and measurements are required.
It would be better for the paper to include test accuracy for testing and training sets to support text mining is a more efficient and effective algorithm compared to other techniques. Moreover, this paper mentioned Text Mining approach can be used to extract high-quality information from videos. It is to believe that extracting from videos is much more difficult than images and texts. How is it possible to retain its test accuracy at a good level for videos?
Preprocessing an important step to analyze text, so it might be better to have the more details about that. For example, what types of words are usually removed and show we record the relative position of each word in the sentence. If one close related sentences were split into two sentences, how can we capture their relations?
The authors could give more details on the applications of text mining in the healthcare and biomedical domain. For example, how could preprocessing, classification, clustering, and information extraction process be applied to this domain. Other than introduction of existing algorithms (e.g. NER), authors can provide more information about how they performs (with a sample dataset), what are their limitations, and comparisons among different algorithms.
References
Allahyari, M., Pouriyeh, S., Assefi, M., Safaei, S., Trippe, E. D., Gutierrez, J. B., & Kochut, K. (2017). A brief survey of text mining: Classification, clustering, and extraction techniques. arXiv preprint arXiv:1707.02919.