STAT946F17/Decoding with Value Networks for Neural Machine Translation
Introduction
Background Knowledge
- NTM
Neural Machine Translation (NMT), which is based on deep neural networks and provides an end- to-end solution to machine translation, uses an RNN-based encoder-decoder architecture to model the entire translation process. Specifically, an NMT system first reads the source sentence using an encoder to build a "thought" vector, a sequence of numbers that represents the sentence meaning; a decoder, then, processes the "meaning" vector to emit a translation. (Figure 1)[1]

- Sequence-to-Sequence(Seq2Seq) Model
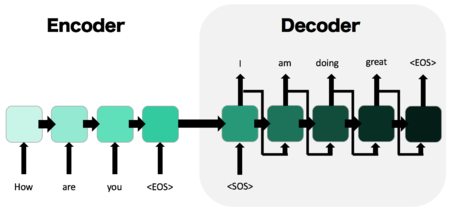
- Two RNNs: an encoder RNN, and a decoder RNN
1) The input is passed though the encoder and it’s final hidden state, the “thought vector” is passed to the decoder as it’s initial hidden state.
2)Decoder given the start of sequence token, <SOS>, and iteratively produces output until it outputs the end of sequence token, <EOS>
- Commonly used in text generation, machine translation, and related problems
- Beam Search
Decoding process:
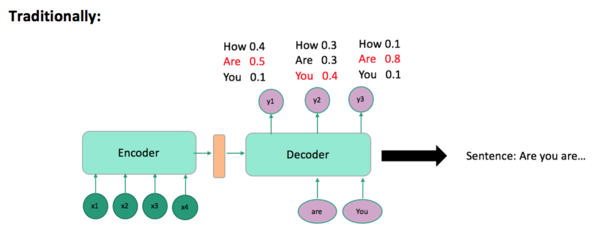
Problem: Choosing the word with highest score at each time step t is not necessarily going to give you the sentence with the highest probability(Figure 3). Beam search solves this problem (Figure 4). Beam search is a heuristic search algorithm such that at each time step t, it takes the top m proposal and continues decoding with each one of them. In the end, you will get a sentence with the highest probability not in the word level. The algorithm terminates if all sentences are completely generated, i.e., all sentences are ended with the <EOS> token.
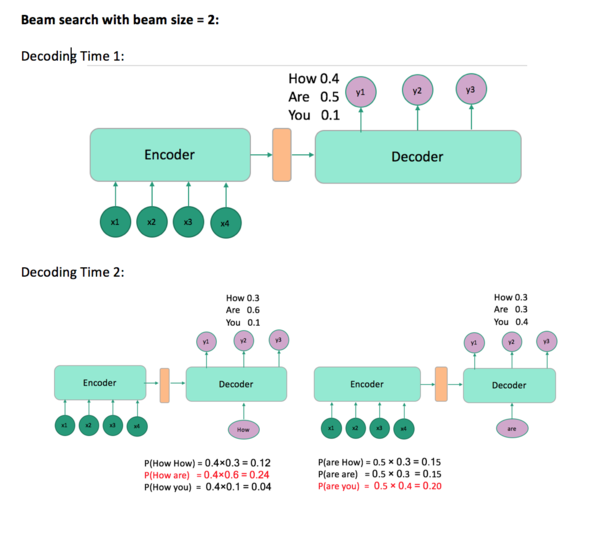
value network
Upon the success of NMT with beam search, beam search tends to focus more on short-term reward, which is called myopic bias. At time t, a word $w$ may be appended to the candidates $y_{<t-1} = y_1,...,y_{t}$ if $P(y_{<t}+w|x) > P(y_{<t}+w'|x)$ even if the word $w'$ is the ground truth translation at step t or can offer a better score in future decodings. By applying the concept of value function in Reinforcement Learning (RL), the authors develop a neural network-based prediction model, \textit{the value network for NMT}, to estimate the long-term reward when appending $w$ to $y_{<t}$ to address the myopic bias.