Dialog-based Language Learning
Note: Do not start editing until 8th Nov 2017
This page will be published for editing by EOD 7th Nov 2017 This page is a summary for NIPS 2016 paper - Dialog-based Language Learning by Jason Weston[1].
Introduction
One of the ways humans learn language, especially second language or language learning by students, is by communication and getting its feedback. However, most existing research in Natural Language Understanding has focused on supervised learning from fixed training sets of labeled data. This kind of supervision is not realistic of how humans learn, where language is both learned by, and used for, communication. When humans act in dialogs (i.e., make speech utterances) the feedback from other human’s responses contain very rich information. This is perhaps most pronounced in a student/teacher scenario where the teacher provides positive feedback for successful communication and corrections for unsuccessful ones.
This paper is about dialog-based language learning, where supervision is given naturally and implicitly in the response of the dialog partner during the conversation. This paper is a step towards the ultimate goal of being able to develop an intelligent dialog agent that can learn while conducting conversations. Specifically this paper explores whether we can train machine learning models to learn from dialog.
Contributions of this paper
- Introduce a set of tasks that model natural feedback from a teacher and hence assess the feasibility of dialog-based language learning.
- Evaluated some baseline models on this data and compared them to standard supervised learning.
- Introduced a novel forward prediction model, whereby the learner tries to predict the teacher’s replies to its actions, which yields promising results, even with no reward signal at all
Background on Memory Networks
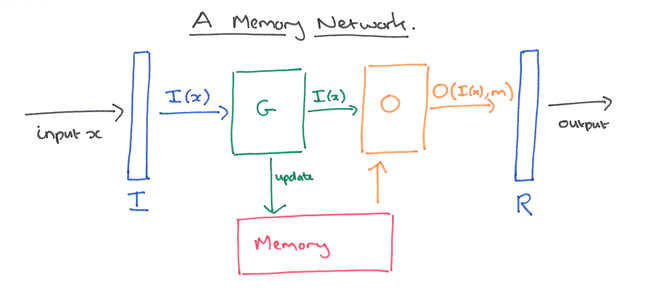
A memory network combines learning strategies from the machine learning literature with a memory component that can be read and written to.
The high-level view of a memory network is as follows:
- There is a memory, m, an indexed array of objects (e.g. vectors or arrays of strings).
- An input feature map I, which converts the incoming input to the internal feature representation
- A generalization component G which updates old memories given the new input.
- An output feature map O, which produces a new output in the feature representation space given the new input and the current memory state.
- A response component R which converts the output into the response format desired – for example, a textual response or an action.
I,G,O and R can all potentially be learned components and make use of any ideas from the existing machine learning literature.
In question answering systems for example, the components may be instantiated as follows:
- I can make use of standard pre-processing such as parsing, coreference, and entity resolution. It could also encode the input into an internal feature representation by converting from text to a sparse or dense feature vector.
- The simplest form of G is to introduce a function H which maps the internal feature representation produced by I to an individual memory slot, and just updates the memory at H(I(x)).
- O Reads from memory and performs inference to deduce the set of relevant memories needed to perform a good response.
- R would produce the actual wording of the question answer based on the memories found by O. For example, R could be an RNN conditioned on the output of O
When the components I,G,O, & R are neural networks, the authors describe the resulting system as a Memory Neural Network (MemNN). They build a MemNN for QA (question answering) problems and compare it to RNNs (Recurrent Neural Network) and LSTMs (Long Short Term Memory RNNs) and find that it gives superior performance.