Unsupervised Neural Machine Translation: Difference between revisions
Line 32: | Line 32: | ||
There are also proposals that use techniques other than direct parallel corpora to do machine translation. Some use a third intermediate language that is well connected to 2 other languages that otherwise have little direct resources. Other works use monolingual data in combination with scarce parallel corpora. | There are also proposals that use techniques other than direct parallel corpora to do machine translation. Some use a third intermediate language that is well connected to 2 other languages that otherwise have little direct resources. Other works use monolingual data in combination with scarce parallel corpora. | ||
The most important contribution | The most important contribution to the problem of training a NMT model with monolingual data was from [He, 2016], which trains two agents to translate in opposite directions (e.g. French → English and English → French) and teach each other through reinforcement learning. However this approach still required a large parallel corpus for a warm start, while our paper does not use parallel data. | ||
= Methodology = | = Methodology = |
Revision as of 12:15, 21 November 2018
This paper was published in ICLR 2018, authored by Mikel Artetxe, Gorka Labaka, Eneko Agirre, and Kyunghyun Cho.
Introduction
The paper presents an unsupervised Neural Machine Translation(NMT) method to machine translation using only monoligual corpora without any alignment between sentences or documents. Monoligual corpora are text corpora that is made up of one language only. This contrasts with the usual Supvervised NMT approach that uses parallel corpora, where two corpora are the direct translation of each other and the translations are aligned by words or sentences. This problem is important as NMT often requires large parallel corpora to achieve good results, however in reality there are a number of languages that lack parallel pairing, e.g. for German-Russian.
Other authors have recently tried to address this problem as well as semi-supervised approaches but these methods still require a strong cross-lingual signal. The proposed method eliminates the need for a cross-lingual information, relying solely on monolingual data.
The general approach of the methodology is to:
- Use monolingual corpora in the source and target languages to learn source and target word embeddings.
- Align the 2 sets of word embeddings in the same latent space.
Then iteratively perform:
- Train an encoder-decoder to reconstruct noisy versions of sentence embeddings for both source and target language, where the encoder is shared and the decoder is different in each language.
- Tune the decoder in each language by back-translating between the source and target language.
Background
Word Embedding Alignment
The paper uses word2vec [Mikolov, 2013] to convert each monoligual corpora to vector enbeddings. These embeddings have been shown to contain the contextual and syntactic features independent of language, and so in theory there could exist a linear map that maps the embeddings from language L1 to language L2.
Figure 1 shows an example of aligning the word embeddings in English and French.
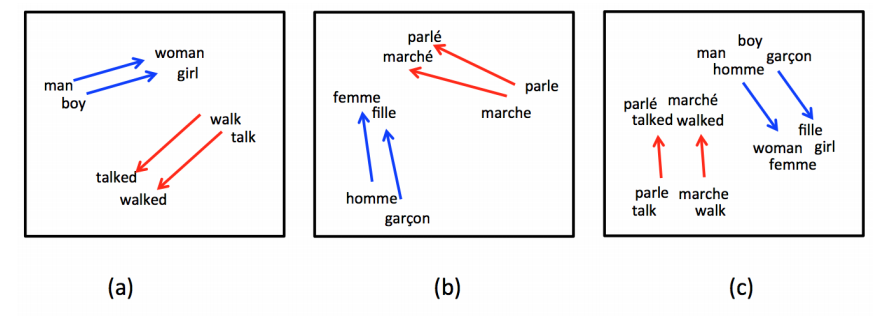
The paper uses the methodology proposed by [Artetxe, 2017] to do cross-lingual embedding aligning in an unsupervised manner and without parallel data. Without going into the details, the general approach of this paper is starting from a seed dictionary of numeral pairings (e.g. 1-1, 2-2, etc.), to iteratively learn the mapping between 2 language embeddings, while concurrently improving the dictionary with the learned mapping at each iteration.
There have been significant work in statistical deciphering technique to induce a machine translation model from monolingual data. These techniques treat the source language as ciphertext and models the distribution of the ciphertext.
There are also proposals that use techniques other than direct parallel corpora to do machine translation. Some use a third intermediate language that is well connected to 2 other languages that otherwise have little direct resources. Other works use monolingual data in combination with scarce parallel corpora.
The most important contribution to the problem of training a NMT model with monolingual data was from [He, 2016], which trains two agents to translate in opposite directions (e.g. French → English and English → French) and teach each other through reinforcement learning. However this approach still required a large parallel corpus for a warm start, while our paper does not use parallel data.
Methodology
The corpora data is first processed in a standard way to tokenize and case the words. The authors also experiment with an additional way of translation using Byte-Pair Encoding(BPE) [Sennrich, 2016], where the translation is done by sub-words instead of words. BPE is often used to improve rare-word translations. To test the effectiveness of BPE, they limited the vocabulary to the most frequent 50,000 BPE tokens.
The words or BPEs are then converted to word embeddings using word2vec with 300 dimensions, and then aligned between languages using the method proposed by [Artetxe, 2017]. The alignment method proposed by [Artetxe, 2017] is also used as a baseline to evaluate this model as discussed later in Results.
The translation model uses a standard encoder-decoder model with attention. The encoder is a 2-layer bidirectional RNN, and the decoder is a 2 layer RNN. All RNNs use GRU cells with 600 hidden units. The encoder is shared by the source and target language, while the decoder is different by language.
Although the architecture uses standard models, the proposed system differs from the standard NMT through 3 aspects:
- Dual structure: NMT usually are built for one direction translations English[math]\displaystyle{ \rightarrow }[/math]French or French[math]\displaystyle{ \rightarrow }[/math]English, whereas the proposed model trains both directions at the same time translating English[math]\displaystyle{ \leftrightarrow }[/math]French.
- Shared encoder: one encoder is shared for both source and target languages in order to produce a representation in the latent space independent of language, and each decoder learns to transform the representation back to its corresponding language.
- Fixed embeddings in the encoder: Most NMT systems initialize the embeddings and update them during training, whereas the proposed system trains the embeddings in the beginning and keeps these fixed throughout training, so the encoder receives language-independent representations of the words. This requires existing unsupervised methods to create embeddings using monolingual corpora as discussed in background.
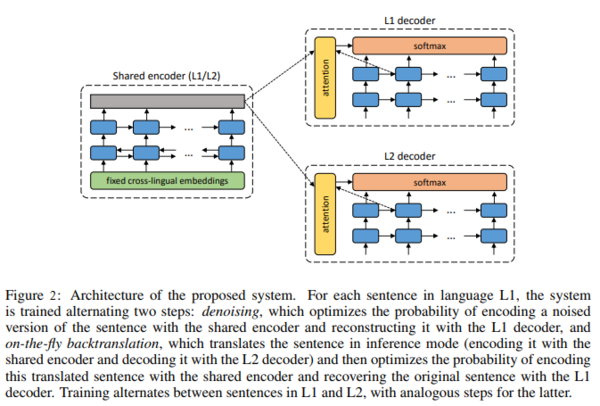
The translation model iteratively improves the encoder and decoder by performing 2 tasks: Denoising, and Back-translation.
Denoising
Random noise is added to the input sentences in order to allow the model to learn some structure of languages. Without noise, the model would simply learn to copy the input word by word. Noise also allows the shared encoder to compose the embeddings of both languages in a language-independent fashion, and then be decoded by the language dependent decoder.
Denoising works to reconstruct a noisy version of the same language back to the original sentence. In mathematical form, if [math]\displaystyle{ x }[/math] is a sentence in language L1:
- Construct [math]\displaystyle{ C(x) }[/math], noisy version of [math]\displaystyle{ x }[/math],
- Input [math]\displaystyle{ C(x) }[/math] into the current iteration of the shared encoder and use decoder for L1 to get reconstructed [math]\displaystyle{ \hat{x} }[/math].
The training objective is to minimize the cross entropy loss between [math]\displaystyle{ {x} }[/math] and [math]\displaystyle{ \hat{x} }[/math].
In other words, the whole system is optimized to take an input sentence in a given language, encode it using the shared encoder, and reconstruct the original sentence using the decoder of that language.
The proposed noise function is to perform [math]\displaystyle{ N/2 }[/math] random swaps of words that are near each other, where [math]\displaystyle{ N }[/math] is the number of words in the sentence.
Back-Translation
With only denoising, the system doesn't have a goal to improve the actual translation. Back-translation works by using the decoder of the target language to create a translation, then encoding this translation and decoding again using the source decoder to reconstruct a the original sentence. In mathematical form, if [math]\displaystyle{ C(x) }[/math] is a noisy version of sentence [math]\displaystyle{ x }[/math] in language L1:
- Input [math]\displaystyle{ C(x) }[/math] into the current iteration of shared encoder and the decoder in L2 to construct translation [math]\displaystyle{ y }[/math] in L1,
- Construct [math]\displaystyle{ C(y) }[/math], noisy version of translation [math]\displaystyle{ y }[/math],
- Input [math]\displaystyle{ C(y) }[/math] into the current iteration of shared encoder and the decoder in L1 to reconstruct [math]\displaystyle{ \hat{x} }[/math] in L1.
The training objective is to minimize the cross entropy loss between [math]\displaystyle{ {x} }[/math] and [math]\displaystyle{ \hat{x} }[/math].
Contrary to standard back-translation that uses an independent model to back translate the entire corpus at one time, the system uses mini-batches and the dual architecture to generate pseudo-translations and then train the model with the translation, improving the model iteratively as the training progresses.
Training
Training is done by alternating these 2 objectives from mini-batch to mini-batch. Each iteration would perform one mini-batch of denoising for L1, another one for L2, one mini-batch of back-translation from L1 to L2, and another one from L2 to L1. The procedure is repeated until convergence. During decoding, greedy decoding was used at training time for back-translation, but actual inference at test time was done using beam-search with a beam size of 12.
Optimizer choice and other hyperparameters can be found in the paper.
Experiments and Results
The model is evaluated using the Bilingual Evaluation Understudy(BLEU) Score, which is typically used to evaluate the quality of the translation, using a reference (groud-truth) translation.
The paper trains translation model under 3 different settings to compare the performance (Table 1). All training and testing data used was from a standard NMT dataset, WMT'14.
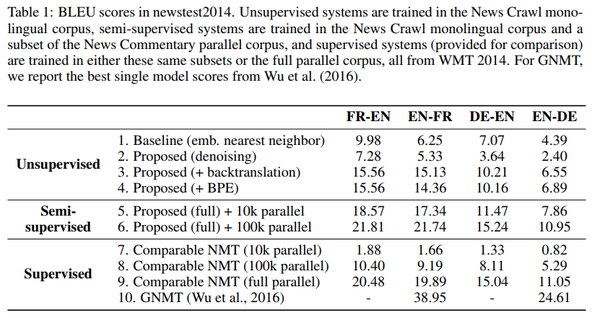
Unsupervised
The model only has access to monolingual corpora, using the News Crawl corpus with articles from 2007 to 2013. The baseline for unsupervised is the method proposed by [Artetxe, 2017], which was the unsupervised word vector alignment method discussed in the Background section.
The paper adds each component piece-wise when doing evaluation to test the impact each piece has on the final score. As shown in Table1, Unsupervised results compared to the baseline of word-by-word results are strong, with improvement between 40% to 140%. Results also show that back-translation is essential. Denoising doesn't show a big improvement however it is required for back-translation, because otherwise back-translation would translate nonsensical sentences.
For the BPE experiment, results show it helps in some language pairs but detracts in some other language pairs. This is because while BPE helped to translate some rare words, it increased the error rates in other words.
Semi-supervised
Since there is often some small parallel data but not enough to train a Neural Machine Translation system, the authors test a semi-supervised setting with the same monolingual data from the unsupervised settings together with either 10,000 or 100,000 random sentence pairs from the News Commentary parallel corpus. The supervision is included to improve the model during the back-translation stage to directly predict sentences that are in the parallel corpus.
Table1 shows that the model can greatly benefit from addition of a small parallel corpus to the monolingual corpora. It is surprising that semi-supervised in row 6 outperforms supervised in row 7, one possible explanation is that both semi-supervised training set and the test set belong to the news domain, whereas the supervised training set is all domains of corpora.
Supervised
This setting provides an upper bound to the unsupervised proposed system. The data used was the combination of all parallel corpora provided at WMT 2014.
The Comparable NMT was trained using the same proposed model except it does not use monolingual corpora, and consequently it was trained without denoising and back-translation. The proposed model under supervised setting does much worse than the state of the NMT in row 10, which suggests that adding the additional constraints to enable unsupervised learning also limits the potential performance.
Qualitative Analysis
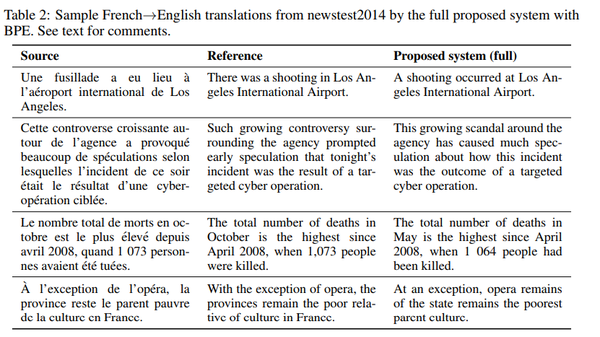
Table 2 shows 4 examples of French to English translations. Example 1 and 2 show that the model is able to model structural differences in the languages (ex.e, it correctly translates "l’aeroport international de Los Angeles" as "Los Angeles International Airport", and it is capable of producing high quality translations of long and more complex sentences. However in Example 3 and 4, the system failed to translate the months and numbers correctly and having difficulty with comprehending odd sentence structures.
Conclusions and Future Work
The paper presented an unsupervised model to perform translations with monolingual corpora by using an attention based encoder-decoder system and training using denoise and back-translation.
Although experimental results show that the proposed model is effective as an unsupervised approach, there is significant room for improvement when using the model in a supervised way, suggesting the model is limited by the architectural modifications. Some ideas for future improvement include:
- Instead of using fixed cross-lingual word embeddings in the beginning which forces the encoder to learn a common representation for both languages, progressively update the weight of the embeddings as training progresses.
- Decouple the shared encoder into 2 independent encoders at some point during training
- Progressively reduce the noise level
- Incorporate character level information into the model, which might help address some of the adequacy issues observed in our manual analysis
- Use other noise/denoising techniques, and analyze their effect in relation to the typological divergences of different language pairs.
Critique
While the idea is interesting and results are impressive for an unsupervised approach, much of the model had actually already been proposed by other papers that are referenced. The paper doesn't add a lot of new ideas but only builds on existing techniques and combines them in a different way to achieve good experimental results. However it is a great step in this direction.
The results showed that the proposed system performed far worse than state of the art when used in a supervised setting, which is concerning and shows that the techniques used creates a limitation and a ceiling for performance.
The best results shown are between two very closely related languages(English and French), and does much worse for English - German, even though English and German are also closely related (but less so than English and French) which suggests that the model may not be successful at translating between distant language pairs. More testing would be interesting to see.
The results comparison could have shown how the semi-supervised version of the model scores compared to other semi-supervised approaches as touched on in the other works section.
References
- [Mikolov, 2013] Tomas Mikolov, Ilya Sutskever, Kai Chen, Greg S Corrado, and Jeff Dean. "Distributed representations of words and phrases and their compositionality."
- [Artetxe, 2017] Mikel Artetxe, Gorka Labaka, Eneko Agirre, "Learning bilingual word embeddings with (almost) no bilingual data".
- [Gouws,2016] Stephan Gouws, Yoshua Bengio, Greg Corrado, "BilBOWA: Fast Bilingual Distributed Representations without Word Alignments."
- [He, 2016] Di He, Yingce Xia, Tao Qin, Liwei Wang, Nenghai Yu, Tieyan Liu, and Wei-Ying Ma. "Dual learning for machine translation."
- [Sennrich,2016] Rico Sennrich and Barry Haddow and Alexandra Birch, "Neural Machine Translation of Rare Words with Subword Units."