STAT946F17/Conditional Image Generation with PixelCNN Decoders: Difference between revisions
No edit summary |
No edit summary |
||
Line 1: | Line 1: | ||
=NOT DONE YET!= | =NOT DONE YET!= | ||
=Introduction= | =Introduction= | ||
This works is based of the widely used PixelCNN and PixelRNN, introduced by Oord et al. in [[#Reference|[1]]]. From the previous work, the authors observed that PixelRNN performed better than PixelCNN, however, PixelCNN was faster to compute as you can parallize the training process. In this work, Oord et al. [[#Reference|[2]]] introduced a Gated PixelCNN, which is a convolutional variant of the PixelRNN model, based on PixelCNN. In particular, the Gated PixelCNN uses explicit probability densities to generate new images using autoregressive connections to model images through pixel-by-pixel computation by decomposing the joint image distribution as a product of conditionals. The Gated PixelCNN is an improvement over the PixelCNN by removing the "blindspot" problem, and to yield a better performance, the authors replaced the ReLU units with sigmoid and tanh activation function. The proposed Gated PixelCNN combines the strength of both PixelRNN and PixelCNN - that is by matching the log-likelihood of PixelRNN on both CIFAR and ImageNet along with the quicker computational time presented by the PixelCNN. Moreover, the authors also introduced a conditional Gated PixelCNN variant (called Conditional PixelCNN) which has the ability to generate images based on class labels, tags, as well as latent embeddings to create new image density models. These embeddings capture high level information of an image to generate a large variety of images with similar features; for instance, the authors can generate different poses of a person based on a single image by conditioning on a one-hot encoding of the class. This approach provided insight into the invariances of the embeddings which enabled the authors to generate different poses of the same person based on a single image. Finally, the authors also presented a PixelCNN Auto-encoder variant which essentially replaces the | This works is based of the widely used PixelCNN and PixelRNN, introduced by Oord et al. in [[#Reference|[1]]]. From the previous work, the authors observed that PixelRNN performed better than PixelCNN, however, PixelCNN was faster to compute as you can parallize the training process. In this work, Oord et al. [[#Reference|[2]]] introduced a Gated PixelCNN, which is a convolutional variant of the PixelRNN model, based on PixelCNN. In particular, the Gated PixelCNN uses explicit probability densities to generate new images using autoregressive connections to model images through pixel-by-pixel computation by decomposing the joint image distribution as a product of conditionals. The Gated PixelCNN is an improvement over the PixelCNN by removing the "blindspot" problem, and to yield a better performance, the authors replaced the ReLU units with sigmoid and tanh activation function. The proposed Gated PixelCNN combines the strength of both PixelRNN and PixelCNN - that is by matching the log-likelihood of PixelRNN on both CIFAR and ImageNet along with the quicker computational time presented by the PixelCNN. Moreover, the authors also introduced a conditional Gated PixelCNN variant (called Conditional PixelCNN) which has the ability to generate images based on class labels, tags, as well as latent embeddings to create new image density models. These embeddings capture high level information of an image to generate a large variety of images with similar features; for instance, the authors can generate different poses of a person based on a single image by conditioning on a one-hot encoding of the class. This approach provided insight into the invariances of the embeddings which enabled the authors to generate different poses of the same person based on a single image. Finally, the authors also presented a PixelCNN Auto-encoder variant which essentially replaces the deconvolutional decoder with the PixelCNN. | ||
=Gated PixelCNN= | =Gated PixelCNN= | ||
Pixel-by-pixel is a simple generative method wherein given an image of dimension of dimension $x_{n^2}$, we iterate, employ feedback and capture pixel densities from every pixel to predict our "unknown" pixel density $x_i$. To do this, the traditional PixelCNNs and PixelRNNs adopted the joint | Pixel-by-pixel is a simple generative method wherein given an image of dimension of dimension $x_{n^2}$, we iterate, employ feedback and capture pixel densities from every pixel to predict our "unknown" pixel density $x_i$. To do this, the traditional PixelCNNs and PixelRNNs adopted the joint distribution p(x), wherein the pixels of a given image is the product of the conditional distributions. Hence, the authors employ autoregressive models which means they just use plain chain rule for joint distribution, depicted in Equation 1. So the very first pixel is independent, second depend on first, third depends on first and second and so on. Basically you just model your image as sequence of points where each pixel depends linearly on previous ones. Equation 1 depicts the joint distribution where x_i is a single pixel: | ||
$$p(x) = \prod\limits_{i=1}^{n^2} p(x_i | x_1, ..., x_{i-1})$$ | $$p(x) = \prod\limits_{i=1}^{n^2} p(x_i | x_1, ..., x_{i-1})$$ | ||
where $p(x)$ is the generated image, $n^2$ is the number of pixels, and $p(x_i | x_1, ..., x_{i-1})$ is the probability of the $i$th pixel which depends on the values of all previous pixels. It is important to note that $p(x_0, x_1, ..., x_{n^2})$ is the joint probability based on the chain rule - which is a product of all conditional distributions $p(x_0) \times p(x_1|x_0) \times p(x_2|x_1, x_0)$ and so on. Figure 1 provides a pictoral understanding of the joint distribution which displays that the pixels are computed pixel-by-pixel for every row, and the forthcoming pixel depends on the pixels values above and to the left of the pixel in concern. | |||
[[File:xi_img.png|500px|center|thumb]] | |||
Revision as of 00:10, 18 November 2017
NOT DONE YET!
Introduction
This works is based of the widely used PixelCNN and PixelRNN, introduced by Oord et al. in [1]. From the previous work, the authors observed that PixelRNN performed better than PixelCNN, however, PixelCNN was faster to compute as you can parallize the training process. In this work, Oord et al. [2] introduced a Gated PixelCNN, which is a convolutional variant of the PixelRNN model, based on PixelCNN. In particular, the Gated PixelCNN uses explicit probability densities to generate new images using autoregressive connections to model images through pixel-by-pixel computation by decomposing the joint image distribution as a product of conditionals. The Gated PixelCNN is an improvement over the PixelCNN by removing the "blindspot" problem, and to yield a better performance, the authors replaced the ReLU units with sigmoid and tanh activation function. The proposed Gated PixelCNN combines the strength of both PixelRNN and PixelCNN - that is by matching the log-likelihood of PixelRNN on both CIFAR and ImageNet along with the quicker computational time presented by the PixelCNN. Moreover, the authors also introduced a conditional Gated PixelCNN variant (called Conditional PixelCNN) which has the ability to generate images based on class labels, tags, as well as latent embeddings to create new image density models. These embeddings capture high level information of an image to generate a large variety of images with similar features; for instance, the authors can generate different poses of a person based on a single image by conditioning on a one-hot encoding of the class. This approach provided insight into the invariances of the embeddings which enabled the authors to generate different poses of the same person based on a single image. Finally, the authors also presented a PixelCNN Auto-encoder variant which essentially replaces the deconvolutional decoder with the PixelCNN.
Gated PixelCNN
Pixel-by-pixel is a simple generative method wherein given an image of dimension of dimension $x_{n^2}$, we iterate, employ feedback and capture pixel densities from every pixel to predict our "unknown" pixel density $x_i$. To do this, the traditional PixelCNNs and PixelRNNs adopted the joint distribution p(x), wherein the pixels of a given image is the product of the conditional distributions. Hence, the authors employ autoregressive models which means they just use plain chain rule for joint distribution, depicted in Equation 1. So the very first pixel is independent, second depend on first, third depends on first and second and so on. Basically you just model your image as sequence of points where each pixel depends linearly on previous ones. Equation 1 depicts the joint distribution where x_i is a single pixel:
$$p(x) = \prod\limits_{i=1}^{n^2} p(x_i | x_1, ..., x_{i-1})$$
where $p(x)$ is the generated image, $n^2$ is the number of pixels, and $p(x_i | x_1, ..., x_{i-1})$ is the probability of the $i$th pixel which depends on the values of all previous pixels. It is important to note that $p(x_0, x_1, ..., x_{n^2})$ is the joint probability based on the chain rule - which is a product of all conditional distributions $p(x_0) \times p(x_1|x_0) \times p(x_2|x_1, x_0)$ and so on. Figure 1 provides a pictoral understanding of the joint distribution which displays that the pixels are computed pixel-by-pixel for every row, and the forthcoming pixel depends on the pixels values above and to the left of the pixel in concern.
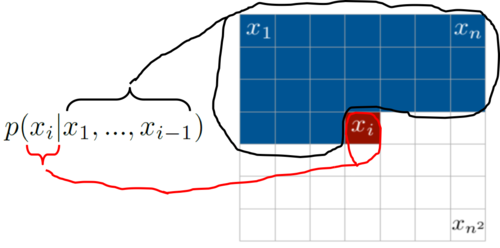
Summary
$\bullet$ Improved PixelCNN
- Similar performance as PixelRNN, and quick to compute like PixelCNN (since it is easier to parallelize)
- Fixed the "blind spot" problem by introducing 2 stacks (horizontal and vertical)
- Gated activation units which now use sigmoid and tanh instead of ReLU units
$\bullet$ Conditioned Image Generation
- One-shot conditioned on class-label
- Conditioned on portrait embedding
- Pixel AutoEncoders
Reference
- Aaron van den Oord et al., "Pixel Recurrent Neural Network", ICML 2016
- Aaron van den Oord et al., "Conditional Image Generation with PixelCNN Decoders", NIPS 2016