stat940F24: Difference between revisions
(82 intermediate revisions by 5 users not shown) | |||
Line 293: | Line 293: | ||
* <math>y</math> is the original label, | * <math>y</math> is the original label, | ||
* <math>k</math> is the number of classes. | * <math>k</math> is the number of classes. | ||
The reason for using label smoothing: | |||
* '''Prevents overfitting''': By preventing the model from becoming too confident in its predictions, label smoothing reduces the likelihood of overfitting. | |||
* '''Improves generalization''': Label smoothing can help the model generalize better to unseen data, as it discourages overconfidence in training. | |||
== Bagging/Ensemble == | == Bagging/Ensemble == | ||
Bagging (short for bootstrap | Bagging (short for bootstrap aggregating) is a machine learning ensemble technique for reducing generalization error by combining several models (Breiman, 1994) | ||
Explanation: Aggregating is to ombine the predictions of each model, often using voting (for classification) or averaging (for regression). | |||
It works by training several different models separately, and then have all of the models vote on the output for test examples. For example, random forest, which is a popular bagging algorithm that builds multiple decision trees on different bootstrap samples of the data and aggregates their predictions. | |||
The reason why bagging works: | |||
* '''Variance reduction''': By training multiple models on different data subsets, bagging reduces the variance of the predictions. This means that it helps models generalize better to new, unseen data. | |||
* '''Handling overfitting''': Bagging is particularly useful for high-variance models (e.g., decision trees) as it helps reduce overfitting. | |||
While bagging is highly useful for traditional machine learning models, it is less commonly used in deep learning because modern neural networks are already highly expressive. Instead, other ensemble methods, such as model ensembling or dropout, are used. | |||
Code Sample: | |||
from sklearn.ensemble import RandomForestClassifier | |||
from sklearn.datasets import load_iris | |||
from sklearn.model_selection import train_test_split | |||
- Load a dataset (Iris dataset for example) | |||
data = load_iris() | |||
X_train, X_test, y_train, y_test = train_test_split(data.data, data.target, test_size=0.3, random_state=42) | |||
- Train a random forest classifier (bagging technique) | |||
rf_model = RandomForestClassifier(n_estimators=100, random_state=42) | |||
rf_model.fit(X_train, y_train) | |||
- Make predictions | |||
y_pred = rf_model.predict(X_test) | |||
- Evaluate the model | |||
accuracy = rf_model.score(X_test, y_test) | |||
== Dropout == | == Dropout == | ||
Line 344: | Line 382: | ||
Use a single neural net without dropout | Use a single neural net without dropout | ||
If a unit is retained with probability p during training, the outgoing weights of that unit are multiplied by p at test time. <math>p \cdot w</math> | If a unit is retained with probability p during training, the outgoing weights of that unit are multiplied by p at test time. <math>p \cdot w</math> | ||
== Additional Regularization == | |||
=== L1 norm === | |||
L1 norm regularization, also known as Lasso, is a technique that adds a penalty equal to the absolute value of the magnitude of coefficients to the loss function. This encourages sparsity in the learned weights, meaning it forces some of the weights to become exactly zero, effectively selecting important features and reducing model complexity. | |||
=== Mixup === | |||
Mixup is a data augmentation technique that creates new training examples by taking convex combinations of pairs of input data and their labels. By blending images and labels together, the model learns smoother decision boundaries and becomes more robust to adversarial examples and noise. | |||
=== Cutout === | |||
Cutout is a form of data augmentation where random square regions are masked out (set to zero) in input images during training. This forces the model to focus on a broader range of features across the image rather than relying on any single part, leading to better generalization and robustness. | |||
=== Gradient Clipping === | |||
Gradient clipping is a technique used to prevent the gradients from becoming too large during training, which can cause the model to diverge. This is done by capping the gradients at a predefined threshold, ensuring that updates remain stable, especially in models like recurrent neural networks (RNNs) where exploding gradients are a common issue. | |||
=== DropConnect === | |||
DropConnect is a variation of Dropout, but instead of dropping neurons, it randomly drops connections (weights) between neurons during training. This prevents the co-adaptation of neurons while allowing individual neurons to contribute to learning. | |||
=== Data Augmentation (beyond Mixup and Cutout) === | |||
* '''Random Flips and Rotations''': Randomly flipping (either vertical or horizontal) or rotating images during training to make the model invariant to certain transformations. | |||
* '''Color Jittering''': Modifying the brightness, contrast, saturation, and hue of images to make the model more robust to variations in color. | |||
* '''Random Cropping and Scaling''': Randomly cropping and scaling images to force the model to learn from different perspectives and contexts within the data. | |||
For PyTorch augmentation, one can refer https://pytorch.org/vision/stable/transforms.html | |||
== Generalization Paradox == | |||
* Models with many parameters tend to overfit | |||
* However, deep neural network, despite using many parameters, works well with unseen data (look up the Double Descent Curve), the reason remains unknown | |||
This phenomenon is illustrated by the '''Double Descent Curve''', where after reaching a peak in test error (due to overfitting), the error decreases again with further model complexity. The precise reasons remain uncertain, but hypotheses include implicit regularization from optimization methods like SGD, hierarchical feature learning, and the redundancy offered by overparameterization. | |||
== Batch Normalization == | |||
=== Overview === | |||
Batch normalization is a technique used to improve the training process of deep neural networks by normalizing the inputs of each layer. Despite the initial intuition for the method being somewhat incorrect, it has proven to be highly effective in practice. Batch normalization speeds up convergence, allows for larger learning rates, and makes the model less sensitive to initialization, resulting in more stable and efficient training. | |||
Batch normalization motivated by internal covariate shift (2015 lofee & Szegedy) | |||
=== Internal Covariance Shift === | |||
Batch normalization was originally proposed as a solution to the internal covariance shift problem, where the distribution of inputs to each layer changes during training. This shift complicates training because the model must constantly adapt to new input distributions. | |||
The transformation of layers can be described as: | |||
<math> l = F_2(F_1(u, \theta_1), \theta_2) </math> | |||
For a mini-batch of activations <math>X = \{x_1, x_2, \dots, x_m\}</math> from a specific layer, batch normalization proceeds as follows: | |||
1. '''Compute the mean''': <math> \mu_B = \frac{1}{m} \sum_{i=1}^{m} x_i </math> | |||
2. '''Compute the variance''': <math> \sigma_B^2 = \frac{1}{m} \sum_{i=1}^{m} (x_i - \mu_B)^2 </math> | |||
3. '''Normalize the activations''': <math> \hat{x}_i = \frac{x_i - \mu_B}{\sqrt{\sigma_B^2 + \epsilon}} </math> | |||
4. '''Scale and shift''' the normalized activations using learned parameters <math>\gamma</math> (scale) and <math>\beta</math> (shift): <math> y_i = \gamma \hat{x}_i + \beta </math> | |||
where: | |||
* <math>x_i</math> represents the activations in the mini-batch, | |||
* <math>\mu_B</math> is the mean of the mini-batch, | |||
* <math>\sigma_B^2</math> is the variance of the mini-batch, | |||
* <math>\epsilon</math> is a small constant added for numerical stability, | |||
* <math>\hat{x}_i</math> is the normalized activation, | |||
* <math>\gamma</math> and <math>\beta</math> are learned parameters for scaling and shifting. | |||
The batch normalization improves validation accuracy by removing the dropout and enables higher learning rate | |||
=== Batch Normalization === | |||
As mentioned earlier, the original intuition behind batch normalization was found to be incorrect after further research. A paper by Santurkar, S., Tsipras, D., Ilyas, A., & Madry, A. (NeurIPS 2019) contradicted the original 2015 paper on BatchNorm by highlighting the following points: | |||
* Batch normalization does not fix covariate shift. | |||
* If we fix covariate shift, it doesn't help. | |||
* lf we intentionally increase lCS, it doesn't harm. | |||
* Batch Norm is not the only possible normalization. There are alternatives. | |||
Instead, they argue that Batch normalization works better due to other factors, particularly related to its effect on the optimization process: | |||
1. '''Reparameterization of the loss function:''' | |||
* Improved Lipschitzness: Batch normalization improves the Lipschitz continuity of the loss function, meaning that the loss changes at a smaller rate, and the magnitudes of the gradients are smaller. This makes the gradients of the loss more "Lipschitz." | |||
* Better β-smoothness: The loss exhibits significantly better smoothness, which aids in optimization by preventing large, erratic changes in the gradient. | |||
2. '''Variation of the loss function''': BatchNorm reduces the variability of the value of the loss. Consider the variation of the loss function: | |||
<math> \mathcal{L}(x + \eta \nabla \mathcal{L}(x)) </math> where <math> \eta \in [0.05, 0.4] </math>. | |||
A smaller variability of the loss indicates that the steps taken during training are less likely to cause the loss to increase uncontrollably. | |||
3. '''Gradient predictiveness''': BatchNorm enhances the predictiveness of the gradients, meaning the changes in the loss gradient are more stable and predictable. This can be expressed as: | |||
<math> || \nabla \mathcal{L}(x) - \nabla \mathcal{L}(x + \eta \nabla \mathcal{L}(x)) || </math>, where <math> \eta \in [0.05, 0.4] </math>. | |||
A good gradient predictiveness implies that the gradient evaluated at a given point remains relevant over longer distances, which allows for larger step sizes during training. | |||
=== Alternatives to Batch Norm === | |||
'''Weight Normalization''': Weight normalization is a technique where the weights, instead of the activations, are normalized. This method reparameterizes the weight vectors to accelerate the training of deep neural networks. | |||
Tim Salimans and Diederik P. Kingma, "Weight Normalization: A Simple Reparameterization to Accelerate Training of Deep Neural Networks," 2016. | |||
'''ELU (Exponential Linear Unit) and SELU (Scaled Exponential Linear Unit)''': ELU and SELU are two proposed activation functions that have a decaying slope instead of a sharp saturation. They can be used as alternatives to BatchNorm by providing smoother, non-linear activation without requiring explicit normalization. | |||
Djork-Arné Clevert, Thomas Unterthiner, and Sepp Hochreiter, "Fast and Accurate Deep Network Learning by Exponential Linear Units (ELUs)," In International Conference on Learning Representations (ICLR), 2016. | |||
Günter Klambauer, Thomas Unterthiner, Andreas Mayr, and Sepp Hochreiter, "Self-Normalizing Neural Networks," ICLR, 2017. | |||
== Convolutional Neural Network (CNN) == | |||
=== Introduction === | |||
Convolutional networks are simply neural networks that use convolution instead of general matrix multiplication in at least one of their layers. CNN is mainly used for image processing. | |||
=== Convolution === | |||
In ML, convolution means dot product | |||
<math> h = \sigma(\langle x, w\rangle+b)</math> | |||
* Same x, different w -- multi-layer perception (MLP) | |||
* Different x, same w -- CNN (weight sharing) | |||
From class, the following operation is called convolution | |||
<math> s(t) = \int x(a)w(t-a)ds </math> | |||
The convolution operation is typically denoted with an asterisk: | |||
<math> s(t) = (x \ast w)(t) </math> | |||
=== Discrete Convolution === | |||
If we now assume that x and w are defined only on integer t, we can define the discrete convolution: | |||
<math> | |||
s[t] = (x \ast w)(t) = \sum_{a=-\infty}^{\infty} x[a] \, w[t-a] | |||
</math> | |||
<math>w[t-a]</math> represents the sequence <math>w[t]</math> shifted by <math>a</math> units. | |||
=== In practice === | |||
We often use convolutions over more than one axis at a time. | |||
<math> | |||
s[i,j] = (I * K)[i,j] = \sum_m \sum_n I[m,n] K[i-m, j-n] | |||
</math> | |||
* '''Input''': usually a multidimensional array of data. | |||
* '''Kernel''': usually a multidimensional array of parameters that should be learned. | |||
We assume that these functions are zero everywhere but the finite set of points for which we store the values. | |||
We can implement the infinite summation as a summation over a finite number of array elements. | |||
=== Convolution and Cross-Correlation === | |||
Convolution is commutative: | |||
<math> | |||
s[i,j] = (I * K)[i,j] = \sum_m \sum_n I[i-m, j-n] K[m, n] | |||
</math> | |||
Cross-correlation: | |||
<math> | |||
s[i,j] = (I * K)[i,j] = \sum_m \sum_n I[i+m, j+n] K[m, n] | |||
</math> | |||
Many machine learning libraries implement cross-correlation but call it convolution. In the context of backpropagation in neural networks, cross-correlation simplifies the computation of gradients with respect to the input and kernel. | |||
Visualization of Cross-Correlation and Convolution with Matlab (https://www.youtube.com/v/Ma0YONjMZLI) | |||
=== Image to Convolved Feature === | |||
* '''Kernel\filter size''': weight <math>\times</math> height, e.g. 3 <math>\times</math> 3 in below example | |||
* '''Stride''': how many pixels to move the filter each time | |||
* '''Padding''': add zeros (or any other value) around the boundary of the input | |||
=== Example === | |||
The following image illustrates a 2D convolution operation between an input image and a filter to produce a convolved feature map. | |||
'''Image (Input)''': | |||
The grid on the left represents a 5<math>\times</math>5 matrix of pixel values. The orange-highlighted 3<math>\times</math>3 region is part of the image currently being convolved with the filter. | |||
'''Convolution Operation''': | |||
The filter values are applied to the selected region in an element-wise multiplication followed by a summation. The operation is as follows: | |||
<math> (1 \times 1) + (1 \times 0) + (1 \times 1) + (0 \times 0) + (1 \times 1) + (1 \times 0) + (0 \times 1) + (0 \times 0) + (1 \times 1) = 4 </math> | |||
'''Convolved Feature Map''': | |||
The result value <math>4</math>, is placed in the corresponding position (top-left) of the convolved feature map on the right. | |||
[[Image:conv_example.png|thumb|400px|center|One Convolution Example]] | |||
This process is repeated as the filter slides across the entire image. The final feature map is shown below. | |||
[[Image:conv_example_final.png|thumb|400px|center|Final Feature Map]] | |||
=== Sparse Interactions=== | |||
In feed forward neural network '''every''' output unit interacts with every input unit. | |||
* When we have <math>m</math> inputs and <math>n</math> outputs, then matrix multiplication requires <math> (m \times n) </math> parameters. and the algorithms used in practice have <math> O(m \times n) </math> runtime (per example) | |||
Convolutional networks, typically have sparse connectivity (sparse weights). This is accomplished by making the kernel smaller than the input. | |||
* Limit the number of connections each output may have to <math>k</math>, then requires only <math> (k \times n) </math> parameters and <math> O(k \times n) </math> runtime | |||
=== Parameter Sharing === | |||
* In a traditional neural net, each element of the weight matrix is multiplied by one element of the input. i.e. It is used once when computing the output of a layer. | |||
* In CNNs, each member of the kernel is used at every position of the input | |||
* Instead of learning a separate set of parameters for every location, we learn only one set | |||
=== Equivariance === | |||
A function <math>f(x)</math> is '''equivaraint''' to a function <math>g</math> is the following holds: | |||
<math>f(g(x)) = g(f(x))</math> | |||
'''In simple terms''': Applying the function <math>f</math> after <math>g</math> is equivalent to applying <math>g</math> after <math>f</math> | |||
[[Image:equivariance.png|thumb|300px|center|Equivariance]] | |||
==== Equivariance in CNNs ==== | |||
* CNNs are naturally equivariant to translation (Covlution = Shift) | |||
* If an input image is shifted, the output feature map shifts correspondingly, preserving spatial structure | |||
* Importance: This property ensures that CNNs can detect features like edges or corners, no matter where they appear in the image | |||
A convolutional layer has equivariance to translation. For example, | |||
<math>g(x)[i] = x[i-1]</math> | |||
If we apply this transformation to x, then apply convolution, the result will be the same as if we applied convolution to x, then applied the transformation to the output. | |||
For images, convolution creates a 2-D map of where certain features appear in the input. Note that convolution is not equivariant to some other transformations, such as changes in the scale (rescaling) or rotation of an image. | |||
==== Importance of Data Augmentation ==== | |||
Data augmentation is commonly used to make CNNs robust against variations in scale, rotation, or other transformations. This involves artificially modifying the training data by applying transformations like flipping, scaling, and rotating to expose the network to different variations of the same object. | |||
=== Convolutional Networks === | |||
'''The first stage (Convolution):''' The layer performs several convolutions in parallel to produce a set of preactivations. The convolution stage is designed to detect local features in the input image, such as edges and patterns. It does this by applying filters/kernels across the input image. | |||
'''The second stage (Detector): ''' Each preactivation is run through a nonlinear activation function (e.g. rectified linear). This stage introduces nonlinearity into the model, enabling it to learn complex patterns. Without this nonlinearity, the model would be limited to learning only linear relationships. | |||
'''The third stage (Pooling)''' Pooling reduces the spatial dimensions of the feature maps (height and width), helping to make the model more invariant to small translations and distortions in the input image. It also reduces computational load and helps prevent overfitting. | |||
[[Image:cnn.png|thumb|400px|center|CNN Structure]] | |||
=== Pooling=== | |||
Down-sample input size to reduce computation and memory | |||
==== Popular Pooling functions ==== | |||
* The maximum of a rectangular neighborhood (Max pooling operation) | |||
* The average of a rectangular neighborhood | |||
* The L2 norm of a rectangular neighborhood | |||
* A weighted average based on the distance from the central pixel | |||
==== Pooling with Downsampling ==== | |||
Max-pooling with a pool width of 3 and a stride between pools of 2. This reduces the representation size by a factor of 2, which reduces the the computational and statistical burden on the next layer. | |||
==== Pooling and Translations ==== | |||
Pooling helps to make the representation become invariant to small translations of the input. Invariance to local translation can be a very useful property if we care more about whether some feature is present than exactly where it is. For example: In a face, we need not know the exact location of the eyes. | |||
==== Input of Varying Size ==== | |||
Example: we want to classify images of variable size. | |||
The input to the classification layer must have a fixed size. In the final pooling output (for example) four sets of summary statistics, one for each quadrant of an image, regardless of the image size. Feature after pooling is <math>2 \times 2</math>. | |||
It is also possible to dynamically pool features together, for example, by running a clustering algorithm on the locations of interesting features (Boureau et al., 2011). | |||
i.e. a different set of pooling regions for each image. | |||
Learn a single pooling structure that is then applied to all images (Jia et al., 2012) | |||
=== Convolution and Pooling as an Infinitely Strong Prior === | |||
* '''Weak Prior''': a prior distribution that has high entropy, which means there is a high level of uncertainty or spread in the distribution. An example of this would be a Gaussian distribution with high variance | |||
* '''Strong Prior''': has very low entropy, which implies a high level of certainty or concentration in the distribution. An example of this would be a Gaussian distribution with low variance | |||
* '''Infinitely Strong Prior''': places zero probability on some parameters and says a convolutional net is similar to a fully connected net with an infinitely strong prior over its weights | |||
The weights for one hidden unit must be identical to the weights of its neighbor, but shifted in space. The weights must be zero, except for in the small, spatially contiguous receptive field assigned to that hidden unit. | |||
Use of convolution as infinitely strong prior probability distribution over the parameters of a layer. This prior says that the function the layer should learn contains only local interactions and is equivariantto translation. | |||
The use of pooling is infinitely strong prior that each unit should be invariant to small translations. Convolution and pooling can cause underfitting. | |||
=== Practical Issues === | |||
The input is usually not just a grid of real values. It is a grid of vector-valued observations. For example, a color image has a red, green, and blue intensity at each pixel. | |||
When working with images, we usually think of the input and output of the convolution as 3-D tensors. One index into the different channels and two indices into the coordinates of each channel. | |||
Software implementations usually work in batch mode, so they will actually use 4-D tensors, with the fourth axis indexing different examples in the batch. | |||
=== Training === | |||
Suppose we want to train a convolutional network that incorporates convolution of kernel stack <math>K</math> applied to multi-channel image <math>V</math> with stride <math>s</math>:<math>c(K; V; s)</math> | |||
Suppose we want to minimize some loss function <math>J(V; K)</math>. During forward propagation, we will need to use <math>c</math> itself to output <math>Z</math>. | |||
<math>Z</math> is propagated through the rest of the network and used to compute <math>J</math>. | |||
* During backpropagation, we receive a tensor <math>\mathbf{G}</math> such that: | |||
<math> G_{i,j,k} = \frac{\partial}{\partial Z_{i,j,k}} J(V, K) </math> | |||
*To train the network, we compute the derivatives with respect to the weights in the kernel: | |||
<math> g(\mathbf{G}, \mathbf{V}, s)_{i,j,k,l} = \frac{\partial}{\partial Z_{i,j,k}} J(V, K) = \sum_{m,n} G_{i,m,n} V_{j,ms+k,ns+l} </math> | |||
*If this layer is not the bottom layer of the network, we compute the gradient with respect to <math>\mathbf{V}</math> to backpropagate the error further: | |||
<math> h(\mathbf{K}, \mathbf{G}, s)_{i,j,k} = \frac{\partial}{\partial V_{i,j,k}} J(V, K) = \sum_{l,m \lvert s l + m = j} \sum_{n,p \lvert s n + p = k} \sum_{q} K_{q,i,m,p} G_{i,l,n} </math> | |||
=== Random or Unsupervised Features === | |||
The most computationally expensive part of training a convolutional network is learning the features. | |||
* Supervised training with gradient descent requires full forward and backward propagation through the entire network for every gradient update. | |||
* One approach to reduce this cost is to use features that are not learned in a supervised manner, such as random or unsupervised features. | |||
==== Random Initilizations ==== | |||
Simply initialize the convolution kernels randomly. In high-dimension space, random vectors are almost orthogonal to each other (correlated). Features captured by different kernels are independent. | |||
==== Unsupervised Learning ==== | |||
Learn the convolution kernels using an unsupervised criterion. | |||
==== Key insight ==== | |||
Random filters can perform surprisingly well in convolutional networks. | |||
* Layers composed of convolution followed by pooling naturally become frequency-selective and translation-invariant, even with random weights. | |||
Inexpensive architecture selection | |||
* Evaluate multiple convolutional architectures by training only the last layer. | |||
* Choose the best-performing architecture and then fully train it using a more intensive method. | |||
== Residual Networks (ResNet) == | |||
=== Overview === | |||
Deeper models are harder to train due to vanishing/exploding gradients and can be worse than shallower networks if not properly trained. There are advanced networks to deal with the degradation problem. | |||
ResNet, short for Residual Networks, was introduced by Kaiming He et al. from Microsoft Research in 2015. It brought a significant breakthrough in deep learning by enabling the training of much deeper networks, addressing the vanishing gradient problem. | |||
[[Image:resnet.png|thumb|400px|center|ResNet Structure]] | |||
* ResNet introduces the concept of skip connections (or residual connections) that allow the gradient to be directly backpropagated to earlier layers | |||
* Skip connections help in overcoming the degradation problem, where the accuracy saturates and then degrades rapidly as the network depth increases | |||
=== Variants === | |||
Several variants of ResNet have been developed, including ResNet-50, ResNet-101, and ResNet-152, differing in the number of layers. | |||
=== Application === | |||
ResNet has been widely adopted for various computer vision tasks, including image classification, object detection, and facial recognition. | |||
== DenseNet == | |||
=== Overview === | |||
* DenseNet, short for Densely Connected Networks, was introduced by Gao Huang et al. in 2017. | |||
* It is known for its efficient connectivity between layers, which enhances feature propagation and reduces the number of parameters | |||
[[Image:densenet.png|thumb|500px|center|DenseNet Structure]] | |||
=== Key Feature === | |||
The key feature is '''Dense Connectivity'''. | |||
* In DenseNet, each layer receives feature maps from all preceding layers and passes its own feature maps to all subsequent layers | |||
* This dense connectivity improves the flow of information and gradients throughout the network, mitigating the vanishing gradient problem | |||
== Echo State Network == | |||
In RNNs, the ability to capture long-term dependencies is crucial. Set the recurrent and input weights such that the recurrent hidden units do a good job of capturing the history of past inputs, and only learn the output weights. The goal is to access the information from the past implicitly. | |||
The hidden state at time <math>t</math> can be expressed as: | |||
<math>s_t = \sigma(W s_{t-1} + U x_t)</math> | |||
where: | |||
* <math>W</math> represents the recurrent weight matrix, which connects previous hidden states to the current state, | |||
* <math>U</math> is the input weight matrix, responsible for incorporating the current input <math>x_t</math>, | |||
* <math>\sigma</math> is an activation function, like a non-linear function such as a sigmoid or tanh. | |||
It is important to control how small changes in the hidden state propagate through time to ensure the network does not become unstable. | |||
If a change <math>\Delta s</math> in the state at time <math>t</math> is aligned with an eigenvector <math>v</math> of the Jacobian <math>J</math> with eigenvalue <math>\lambda > 1</math>, then the small change <math>\Delta s</math> becomes <math>\lambda \Delta s</math> after one-time step, and <math>\lambda^t \Delta s</math> after <math>t</math> time steps. | |||
If the largest eigenvalue <math>\lambda < 1</math>, the map from <math>t</math> to <math>t+1</math> is contractive. | |||
The network forgets information about the long-term past. | |||
Set the weights to make the Jacobians slightly contractive. This allows the network to gradually forget irrelevant information while still remembering key long-term dependencies. | |||
[[Image:echo_state_net.png|thumb|500px|center|Echo State Network]] | |||
== Long Delays == | |||
RNNs often fail to capture these dependencies due to the vanishing gradient problem. Long delays use recurrent connections. It knows something from the past, help vanishing gradient - even if gradient get vanished during the path, it still has the direct information from the past. | |||
[[Image:long delay.png|thumb|500px|center|Long Delays]] | |||
== Leaky Units == | |||
In some cases, we do need to forget the path while in some we do not since we do not want to remember redundant information. | |||
Recall that: | |||
<math>s_t = \sigma(W s_{t-1} + U x_t)</math> | |||
Then consider the following refined form of the equation (convex combination of the current state and previous through a new parameter): | |||
<math>s_{t,i} = \left(1 - \frac{1}{\tau_i}\right) s_{t-1} + \frac{1}{\tau_i} \sigma(W s_{t-1} + U x_t)</math> | |||
where | |||
* <math>1 \leq \tau_i \leq \infty</math> | |||
* <math>\tau_i = 1</math> corresponds to an ordinary RNN | |||
* <math>\tau_i > 1</math> allows gradients to propagate more easily | |||
* <math>\tau_i \gg 1</math> means the state changes very slowly, integrating past values associated with the input sequence over a long duration | |||
Infinity means the current state is the previous state while one means completely forgetting the previous steps and only depends on the current observations. | |||
== Gated RNNs == | |||
=== Defnition === | |||
It might be useful for the neural network to forget the old state in some cases like if we only care about if the current letter is a or b. | |||
Example: <math>a\ a\ b\ b\ b\ b\ a\ a\ a\ a\ b\ a\ b</math> | |||
It might be useful to keep the memory of the past. | |||
Example: | |||
Instead of manually deciding when to clear the state, we want the neural network to learn to decide when to do it. | |||
=== Long-Short-Term-Memory (LSTM) === | |||
The Long-Short-Term-Memory (LSTM) algorithm was proposed in 1997 (Hochreiter and Schmidhuber, 1997). It is a type of recurrent neural network designed for approaching the vanishing gradient problem. | |||
Several variants of the LSTM are found in the literature: | |||
*Hochreiter and Schmidhuber, 1997 | |||
*Graves, 2012 | |||
*Graves et al., 2013 | |||
*Sutskever et al., 2014 | |||
The principle is always to have a linear self-loop through which gradients can flow for a long duration. | |||
== Gated Recurrent Units (GRU) == | |||
Here is the plain text version of the new image content: | |||
Recent work on gated RNNs, Gated Recurrent Units (GRU), was proposed in 2014. | |||
* Cho et al., 2014 | |||
* Chung et al., 2014, 2015 | |||
* Jozefowicz et al., 2015 | |||
* Chrupala et al., 2015 | |||
Standard RNN computes the hidden layer at the next time step directly: | |||
<math>s_t = \sigma(W s_{t-1} + U x_t)</math> | |||
There are two gates: the update gate and the reset gate. Update gate for the case that we want to keep the information around while reset gate is the case when forgetting. A temporary state locks down some of the values of the current state. | |||
GRU first computes an update gate (another layer) based on the current input vector and hidden state: | |||
<math>z_t = \sigma(U^{(z)} x_t + W^{(z)} s_{t-1})</math> | |||
It also computes the reset gate similarly but with different weights: | |||
<math>r_t = \sigma(U^{(r)} x_t + W^{(r)} s_{t-1})</math> | |||
New memory content is calculated as: | |||
<math>\tilde{s_t} = \tanh(U x_t + r_t \odot W s_{t-1})</math> | |||
which has current observations and forgetting something from the past | |||
If the reset gate is close to 0, this causes the network to ignore the previous hidden state, effectively allowing the model to drop irrelevant information. | |||
The final memory at time step <math>t</math> is a combination of the current and previous time steps: | |||
<math>s_t = z_t \odot s_{t-1} + (1 - z_t) \odot \tilde{s_t}</math> | |||
If the reset gate is close to 0, it will ignore the previous hidden state, allowing the model to discard irrelevant information. | |||
The update gate <math>z_t</math> controls how much of the past state should matter in the current time step. If <math>z_t</math> is close to 1, then we can effectively copy information from the past state across multiple time steps. | |||
Units that need to capture short-term dependencies often have highly active reset gates. | |||
== Cliping Gradients == | |||
A simple solution for clipping the gradient. (Mikolov, 2012; Pascanu et al., 2013): | |||
* Clip the parameter gradient from a mini-batch element-wise (Mikolov, 2012) just before the parameter update. | |||
* Clip the norm <math>g</math> of the gradient <math>g</math> (Pascanu et al., 2013a) just before the parameter update. | |||
The formula for clipping the gradient is: | |||
<math>g' = \min\left(1, \frac{c}{|g|}\right) g</math> | |||
where c is a constant. | |||
== Attention == | |||
The attention mechanism was introduced to improve the performance of the encoder-decoder model for machine translation. | |||
=== Common Representation === | |||
A single 'concept' is universally represented, transcending specific languages or forms. | |||
* '''Encoder''': Processes the word 'elephant' from its original source. | |||
* '''Output''': A universal representation vector (the abstract 'concept' of an elephant). | |||
* '''Decoders''': Translate this concept into various domains or applications. | |||
The 'concept' is an abstract entity that exists independently of any particular language or representation. | |||
For example, if we want to translate from English to Spanish | |||
* '''Encoder (English Input)''': The system processes the word "elephant." | |||
* '''Output (Universal Representation)''': The system generates an abstract concept or vector representing an "elephant," independent of any specific language. | |||
* '''Decoder (Spanish Output)''': The system decodes this concept and outputs the equivalent Spanish word: "elefante." | |||
[[Image:attn_exmaple.png|thumb|500px|center|Common Representation]] | |||
=== Sequence-to-Sequence Model === | |||
In the sequence-to-sequence model, every word <math>x_i</math> produces a hidden vector <math>h_i</math> in the encoder part of the autoencoder. The hidden vector of every word, <math>h_i</math>, is fed to the next hidden vector, <math>h_{i+1}</math>, by a projection matrix <math>W</math>. | |||
In this model, for the whole sequence, there is only one context vector <math>c</math>, which is equal to the last hidden vector of the encoder, i.e., <math>c = h_n</math>. | |||
[[Image:sqe2seq.png|thumb|800px|center|Sequence-to-Sequence Model]] | |||
Challenges: | |||
1. '''Long-range dependencies''': As the model processes long sequences, it can struggle to remember and utilize information from earlier steps, especially in cases where long-term context is crucial. | |||
2. '''Sequential processing''': Since these models process data step by step in sequence, they can't take full advantage of parallel processing, which limits the speed and efficiency of training. | |||
These are the core challenges that newer architectures, such as transformers, aim to address. | |||
=== Attention Definition === | |||
The basic idea behind the attention mechanism is directing the focus on important factors when processing data. Attention is a fancy name for '''weighted average'''. | |||
=== Sequence-to-Sequence Model with Attention === | |||
* Sequence-to-sequence models: | |||
Multiple RNN units serve as the encoder. They encode information into the context vectors. Multiple RNN units decode the concept in the context vector to different domain information. The limitations of this approach are long-range dependencies and prevention of parallelization. | |||
<math>p(y_i | y_1, \ldots, y_{i-1}) = g(y_{i-1}, l_i, c)</math> | |||
* Sequence-to-sequence with attention: | |||
Pass multiple context vectors to the decoder. | |||
<math>p(y_i | y_1, \ldots, y_{i-1}) = g(y_{i-1}, l_i, c_i)</math> | |||
[[Image:sqe2seq attn.png|thumb|800px|center|Sequence-to-Sequence Model with Attention]] | |||
There are some calculations: | |||
1. Similarity score: | |||
<math>s_{ij} = similarity(l_{i-1}, h_j)</math> | |||
2. Attention weight: | |||
<math>a_{ij} = \frac{e^{s_{ij}}}{\sum_{k=1}^{T} e^{s_{ik}}}</math> | |||
The attention weight <math>a_{ij}</math> is obtained by applying a softmax function to the similarity scores. This normalizes the scores across all encoder hidden states, turning them into a probability distribution. | |||
3. Context vector: | |||
<math>c_i = \sum_{j=1}^{T} a_{ij} h_j</math> | |||
The effectiveness of the correlation between inputs around position <math>j</math> and the output at position <math>i</math> is crucial. | |||
This score is determined based on: | |||
* The RNN hidden state <math>l_{i-1}</math> just before emitting <math>y_i</math>. | |||
* The <math>j^{th}</math> hidden state <math>h_j</math> of the input sentence. | |||
== Transformer Architecture == | |||
The basic concept behind transformers is attention as summarized in the paper by Vaswani et. al [https://arxiv.org/abs/1706.03762 'Attention is all you need']. This paper claims that all you need is attention and with the structure of attentions, basically you can handle the sequential data. It was based on GPT and many other models that we use in LLM and imaging processing. Transformer is an example of an encoder-decoder architecture. Unlike RNNs, Transformers can process sequences in parallel, making them faster to train on large datasets. Transformers have applications beyond NLP, such as Vision Transformers (ViT) in computer vision and protein structure prediction in biology (AlphaFold). Transformers' ability to capture long-range dependencies efficiently has made them the standard for many modern AI models. | |||
=== Encoder === | |||
The encoder consists of two main components: Self Attention and Feed Forward Neural Network. The architecture of the Encoder is given below: | |||
[[File:Encoder.png|center|thumb|Encoder architecture of the Transformer]] | |||
==== Self Attention ==== | |||
Understanding the individual words in a sentence is not enough to understand the whole sentence and one needs to understand how the words relate to each other. The attention mechanism forms composite representations. We aim to have embeddings of words and embeddings of compositions at the same time in different levels. Unlike word2vec introduced in 2013 by Mikolov et al., which captures the vector representations of words in an embedding space such that words that are similar are represented closer to each other, self-attention aims to capture the similarity between words based on context and in relation to each other. Multiple layers help form complex concept representations. For example, the context of the word "bank" differs based on if the surrounding words involve "money" or "river". | |||
[[File:word2vec.jpg|center|thumb|Illustration of word2vec where "King" - "Man" + "Woman" produces a vector close to the word "Queen"]] | |||
Self-attention is analogous to the fundamental retrieval strategy in databases where given a query, and key-value pairs, we use the query to identify the key and retrieve the corresponding value. The generalized definition for calculating the attention of a target word with respect to the input word: use the '''Query''' of the target and the '''Key''' of the input and then calculate a matching score. These matching scores act as the weights of the '''Value''' vectors. | |||
Then we look at the definition if matrix form. Given an input vector <math>\mathbf{x} \in \mathbb{R}^d</math>, the weights for the query, key, and value transformations are defined as: | |||
* <b>Query weight matrix</b>: <math>\mathbf{W}_q \in \mathbb{R}^{d \times p}</math> | |||
* <b>Key weight matrix</b>: <math>\mathbf{W}_k \in \mathbb{R}^{d \times p}</math> | |||
* <b>Value weight matrix</b>: <math>\mathbf{W}_v \in \mathbb{R}^{d \times r}</math> | |||
The transformations for the query (<math>\mathbf{q}</math>), key (<math>\mathbf{k}</math>), and value (<math>\mathbf{v}</math>) vectors are given by: | |||
* <b>Query vector</b>: <math>\mathbf{q} = \mathbf{W}_q^T \mathbf{x}</math> where <math>\mathbf{q} \in \mathbb{R}^{p \times 1}</math>, since <math>\mathbf{W}_q^T \in \mathbb{R}^{p \times d}</math>, and <math>\mathbf{x} \in \mathbb{R}^{d \times 1}</math> | |||
* <b>Key vector</b>: <math>\mathbf{k} = \mathbf{W}_k^T \mathbf{x}</math> where <math>\mathbf{k} \in \mathbb{R}^{p \times 1}</math>, since <math>\mathbf{W}_k^T \in \mathbb{R}^{p \times d}</math>, and <math>\mathbf{x} \in \mathbb{R}^{d \times 1}</math> | |||
* <b>Value vector</b>: <math>\mathbf{v} = \mathbf{W}_v^T \mathbf{x}</math> where <math>\mathbf{v} \in \mathbb{R}^{r \times 1}</math>, since <math>\mathbf{W}_v^T \in \mathbb{R}^{r \times d}</math>, and <math>\mathbf{x} \in \mathbb{R}^{d \times 1}</math> | |||
The transformations allow the attention mechanism to compute similarity scores between the query and key vectors and to use the value vectors to produce the final weighted output as <math>\mathbf{z_1} = \alpha_1 \mathbf{v}_1 + \alpha_2 \mathbf{v}_2 + \ldots + \alpha_n \mathbf{v}_n</math>, where <math>\mathbf{v}_i</math> are similar to the input in CNNs, and <math>\alpha_i</math> are similar to the kernels in CNNs. | |||
However, unlike the kernels in CNNs, the <math>\alpha_i</math>'s are data-dependent and given by: | |||
<math>\alpha_i = \text{softmax}\left(\frac{\mathbf{q}^T \mathbf{k}_i}{\sqrt{p}}\right)</math>. | |||
Extending this to the entire dataset, the equations are: | |||
<math> | |||
\mathbf{X} = [\mathbf{x}_1, \mathbf{x}_2, \ldots, \mathbf{x}_n] \in \mathbb{R}^{d \times n} | |||
</math> | |||
<math> | |||
\mathbf{Q} = [\mathbf{q}_1, \mathbf{q}_2, \ldots, \mathbf{q}_n] \in \mathbb{R}^{p \times n} | |||
</math> | |||
<math> | |||
\mathbf{K} = [\mathbf{k}_1, \mathbf{k}_2, \ldots, \mathbf{k}_n] \in \mathbb{R}^{p \times n} | |||
</math> | |||
<math> | |||
\mathbf{V} = [\mathbf{v}_1, \mathbf{v}_2, \ldots, \mathbf{v}_n] \in \mathbb{R}^{r \times n} | |||
</math> | |||
The transformations are defined as: | |||
<math> | |||
\mathbf{Q}_{p \times n} = \mathbf{W}_{q}^{T_{p \times d}} \mathbf{X}_{d \times n} | |||
</math> | |||
<math> | |||
\mathbf{K}_{p \times n} = \mathbf{W}_{k}^{T_{p \times d}} \mathbf{X}_{d \times n} | |||
</math> | |||
<math> | |||
\mathbf{V}_{r \times n} = \mathbf{W}_{v}^{T_{r \times d}} \mathbf{X}_{d \times n} | |||
</math> | |||
Therefore the output, | |||
<math> | |||
\mathbf{Z}_{r \times n} = \mathbf{V}_{r \times n} \cdot \text{softmax}\left(\frac{\mathbf{Q}^{T} \mathbf{K}}{\sqrt{p}}\right)_{n \times n} | |||
</math> | |||
Additionally, we have: | |||
<math> | |||
\mathbf{Q}^{T} \mathbf{K} = \mathbf{X}^{T} \mathbf{W}_{q} \mathbf{W}_{k}^{T} \mathbf{X} \quad \text{(an asymmetric kernel extracting the global similarity between words)} | |||
</math> | |||
Let us take a closer look at how one word is processed in the encoder. | |||
[[File:Encoder-Deeper-View.jpg|center|thumb|Deeper view of the Encoder]] | |||
The input vector x is passed through a linear transformation layer which transforms the input into query q, key k, and value v vectors, given by the equations above. This is passed through a stacked multi-head self-attention layer which extracts global information across pairwise sequence of words to produce the output vector Z also given by the equation above. The equation for Z can be compared to how similarity is computed between the key and value pairs in databases. This output is added to the residual input x to preserve the meaning of individual words in addition to the pairwise representations. This is normalized to produce the output <math>\mathbf{(Z+x)} \in \mathbb{R}^{h \times r}</math>. This serves as the input to the feed forward neural network. | |||
==== Feed Forward Neural Network ==== | |||
The structure of the feed forward network (FFN) is Linear Layer 1, followed by ReLU activation and then another linear layer 2. While the attention mechanism captures global information between words and hence aggregates across columns, the feed forward neural network aggregates across rows and takes a more broader look at each word independently. Depsite the individual processing, all positions share the same set of weights and biases in the FFN. In a classroom environment, the attention mechanism is similar to the teacher observing the interactions among students in a group, whereas the feed forward neural network resembles the teacher evaluating each student independently for their understanding of the assignment. The output of the feed forward layer r also has a residual connection from the previous layer which is normalized and passed as the input of the decoder as <math>\mathbf{(r+(z+x))}</math>. The encoder also has a positional encoding component which captures information about the position of words in a sequence. | |||
While the above figure zooms in at how a word vector x is processed by the encoder, the major advantage of the transformer architecture is its ability to handle multiple words in a sequence in parallel and so in practice, the above zoomed in version of the encoder is usually stacked to handle a group of words in parallel. | |||
==== Global v.s. Local Information | |||
For Attention Mechanism: | |||
* '''Global Understanding''': Captures relationships among different positions in the sequence. | |||
* '''Context Aggregation''': Spreads relevant information across the sequence, enabling each position to see a broader context. | |||
For Feed-Forward Networks (FFN): | |||
* '''Local Processing''': While attention looks across the entire sequence, FFN zooms back in to process each position independently. | |||
* '''Individual Refinement''': Enhances the representation of each position based on its own value, refining the local information gathered so far. | |||
=== Decoder === | |||
The decoder consist of three main components: Masked Self Attention, Cross Attention and Linear Layer. The architecture of the Decoder is given below: | |||
[[File:Transformer-Decoder.png|center|thumb|Decoder architecture of the Transformer]] | |||
==== Masked Self Attention ==== | |||
In masked self attention, we add a mask matrix <math>\mathbf{M} \in \mathbb{R}^{n \times n}</math> to the normalized argument within the softmax of <math>\mathbf{Z}</math> given by | |||
<math> | |||
\mathbf{Z}_{r \times n} = \mathbf{V}_{r \times n} \cdot \text{softmax}\left(\frac{\mathbf{Q}^{T} \mathbf{K} + \mathbf{M}}{\sqrt{p}}\right)_{n \times n} | |||
</math> | |||
The reason for adding a mask matrix M is to ensure that the output is informed only by the past words and not by the words further along in the sequence. The mask matrix therefore is given by: | |||
<math>M(i,j) = \begin{cases} | |||
0 & \text{if } j \leq i \\ | |||
-\infty & \text{if } j > i | |||
\end{cases}</math> | |||
This is because <math>\mathbf{Z}</math> is an upper triangular matrix with values for previous words since <math>softmax(x + 0)</math> is the same as <math>softmax(x)</math>, but <math>softmax(x - \infty)</math> will be equal to 0. | |||
==== Cross Attention ==== | |||
The intuition behind cross attention is similar to sequence-to-sequence models where the context vector is passed from the encoder to the decoder capturing relevant information from the input sequence. The residual connection plus the output of the masked self attention is passed as the query to the cross attention block, whereas the key and value pairs are the same as the output of the encoder. The relationship and relevance between words in different sentences are captured. | |||
==== Linear Layer ==== | |||
The linear layer is applied to the output of the feed forward neural network of the decoder and its primary role is to adjust the dimensionality of the network to match the size of the vocabulary. It involves learning a set of parameters - a matrix of dimension equal to <math> p \times len(vocab)</math>. This results in a <math> p \times 1</math> vector which is then passed through a probabilistic softmax layer to predict the next word in the sequence. | |||
==== Softmax Activation ==== | |||
The function transforms the linear layer's output into probabilities as mentioned above. The output represents the likelihood of a respective word being the next word in the sequence. | |||
=== Positional Encoding === | |||
So far, the encoder and decoder has no sense of the order of the words in the sequence. The sentences "I am a teacher", "Teacher I am", "Am I a teacher?" are processed the same way, even though the meaning may not be the same. To circumvent this issue, and due to the lack of the convolution or recurrence operations, a positional encoding scheme is embedded within the encoder and decoder. In this encoding, the position of the words in a sequence is encoded by a vector. The even positions are represented by a sinusoidal wave with 1 representing the peaks and 0 representing the troughs. Similarly, the odd positions are represented by a cosine wave. Thus, each position is encoded by a unique binary vector and each unique binary vector represents a specific position. | |||
== Bidirectional Encoder Representations from Transformers (BERT) == | |||
[https://arxiv.org/abs/1810.04805 BERT] introduced by Google is built by repeating the encoder of the transformer multiple times. The Bidirectional in BERT refers to its ability to attend to the future and past words in the sequence unlike Transformers which only looks at the past to make predictions about the future. The fundamental principles behind BERT are Masked Language Modeling, and Next Sentence Prediction. The architecture of BERT is given below. | |||
[[File:BERT.png|center|thumb|Masked Language Modeling]] | |||
=== Masked Language Modeling === | |||
BERT masks words in a corpus as shown in the figure below and makes the model learn to predict these words. It thereby pays attention to both words before and after the masked word and fills in the blank given the entire context. It is the same as a Transformer Encoder where 12 encoders (in contrast to the 6 in the original paper of Transformers) are stacked on top of each other. The output of the encoder is passed to s linear dense layer and softmax to predict the most probable word from the vocabulary of the corpus. | |||
[[File:MLM.png|center|thumb|Masked Language Modeling]] | |||
=== Next Sentence Prediction === | |||
It was also used to identify if given two sentences A and B, B logically follows the sentence A. This is possible due to the ability to fine-tune the weights of the pretrained BERT model for downstream prediction tasks. The pre-trained model can also be used to represent the input of a sentiment analysis task (for example) to a neural network in a better manner. The [CLS] token is prepended to the input sequence and is used to capture the context of the whole sentence such that during fine-tuning, the prediction may be conditioned on the representation of the [CLS] token. | |||
There are various flavors of BERT based on slight modifications to the original architecture and training processes. The advantage of fine tuning pretrained models is the opportunity to finetune them on a domain specific corpus leading to additional variants of BERT as BioBERT retrained on a biomedical corpus, BERTweet trained on around 850 million tweets and so on. | |||
== Transformers and GPT Models == | |||
=== Overview of Transformers === | |||
* Transformers consist of two main components: | |||
** Encoder | |||
** Decoder | |||
* BERT utilizes a stack of encoders, while GPT uses a stack of decoders. | |||
* GPT models are neural network-based language prediction models built on Transformer architecture. | |||
=== Decoder Structure === | |||
* The decoder has three parts: | |||
*# Masked Multi-Head Attention: Attends only to the left. | |||
*# Cross Attention: Attends to encoded inputs (removed in GPT). | |||
*# Feed Forward Neural Network. | |||
=== Differences Between BERT and GPT === | |||
* Both BERT and GPT use masked multi-head attention, but unlike BERT that masks a word in the middle of the sentence, and tries to predict the mask, GPT masks all the future words and tries to predict them. | |||
* Training methods differ: | |||
** BERT masks tokens in sentences. | |||
** GPT predicts the next token in a sequence. | |||
=== Training Process === | |||
* In GPT, the model predicts the next token based on the previous tokens, treating the input as a sequence. | |||
* The first GPT model (2018) had 117 million parameters and was trained on 7,000 books. | |||
* It was seen that larger models tend to generalize better and have better "emergent abilities", although the reason is still unclear. | |||
=== Evolution of GPT Models === | |||
* GPT-2 (2019) had 1.5 billion parameters. | |||
* GPT-3 (2020) had 175 billion parameters. | |||
* Larger models tend to generalize better. This trend suggests that increasing model size allows for deeper contextual understanding. | |||
=== Chain of Thought Reasoning === | |||
* GPT-4 introduced a method called “chain of thought,” allowing the model to predict intermediate steps in reasoning tasks. It involves multi-step thinking: The model generates a series of logical steps to arrive at the final answer, rather than answering in a single sentence. | |||
* Example: Problem: What is the result of 5 + 5 + 5? It is clear to see the answer is 15. With the chain of thought reasoning - Step 1: The first 5 plus the second 5 gives 10 (i.e. <math>5+5=10</math>). Step 2: add the last 5 to the result from Step 1 (i.e. <math>10+5=15</math>). | |||
=== Alignment and Instruction Following === | |||
* ChatGPT is designed to follow instructions and align with user preferences, addressing issues like harmful or politically incorrect outputs. | |||
* InstructGPT is a variant of GPT that focuses on following instructions using human feedback via Reinforcement Learning from Human Feedback (RLHF). | |||
=== Text-Text Transfer Transformer (T5) === | |||
* T5 combines encoder and decoder structures and treats various NLP tasks as text-to-text problems, allowing for diverse applications like translation and summarization. | |||
* NLP tasks such as sentiment analysis which were primarily treated as classification problems are cast as text-to-text problems. | |||
* Next span prediction is used where a set of sequential tokens are removed and replaced with sentinel tokens. | |||
* T5 architecture operates on an encoder-decoder model and encoder input padding can be performed on both left and right. | |||
=== Training Techniques in T5 === | |||
* T5 masks spans of tokens rather than individual tokens, focusing on global context rather than local dependencies. 15% of tokens are randomly removed and replaced with sentinel tokens. | |||
=== Benchmarking and Performance === | |||
* Models are often evaluated against benchmarks like GLUE and SuperGLUE, which consist of various NLP tasks. | |||
=== Training and Fine-Tuning LLMs === | |||
* LLMs are trained on massive datasets of unlabeled text using unsupervised learning. | |||
* The training process involves predicting the next token in a sequence. | |||
* Fine-tuning is done on labeled data to align the model with specific tasks or instructions. This process is called Supervised Fine-Tuning (SFT). | |||
=== Aligning LLMs with Human Feedback === | |||
* Reinforcement Learning with Human Feedback (RLHF) is used to ensure LLMs produce safe and ethical outputs. | |||
* RLHF involves generating multiple responses for a given prompt and having humans rank them. | |||
* A reward model is trained on this ranked data to predict the quality of a response. | |||
* The original LLM is then fine-tuned using this reward model. | |||
* RLHF was introduced in 2017 and has been widely used in LLMs like ChatGPT. | |||
=== Direct Preference Optimization (DPO) === | |||
* DPO is a newer approach that aims to replace RLHF. | |||
* It directly collects user preferences for different responses, eliminating the need for a reward model. | |||
* DPO is easier to implement and more stable than RLHF. | |||
=== Project Ideas for Deep Learning === | |||
# Enhancing Speculative Decoding for Faster Large Language Modeling | |||
#* This project aims to improve the speed of LLMs by using techniques like rejection sampling and importance sampling. | |||
#* The goal is to find alternative sampling methods to rejection sampling, which is computationally expensive. | |||
# Reducing the Computational Complexity of Transformers | |||
#* Transformers are computationally expensive, especially for long sequences. | |||
#* This project explores methods like ORCID, which uses data-dependent convolution to reduce complexity. | |||
#* The goal is to develop more efficient transformers that can handle longer sequences. | |||
# Diffusion Decoding for Peptide De Novo Sequencing | |||
#* Peptide sequencing is a crucial problem in bioinformatics, involving determining the amino acid sequence of a peptide. | |||
#* This project proposes using diffusion models for peptide sequencing, replacing the traditional GPT-based approach. | |||
#* Diffusion models can potentially improve accuracy by predicting all tokens simultaneously, rather than sequentially. | |||
# Using ORCID for DNA Analysis | |||
#* This project explores using ORCID, a model that can handle larger sequences, for DNA analysis. | |||
#* The goal is to leverage ORCID’s ability to handle long sequences to improve DNA analysis tasks. | |||
# Symbolic Regression with Diffusion Models | |||
#* Symbolic regression involves finding a mathematical formula that best fits a given dataset. | |||
#* This project proposes using diffusion models for symbolic regression, potentially improving the accuracy and efficiency of the process. | |||
== Transformers and Variational Autoencoders == | |||
=== Large Language Models (LLMs) and Transformers === | |||
==== Key Components of Transformers ==== | |||
* Attention Mechanism Formula: The core of the attention mechanism is computed using the following formula: | |||
<center><math display=block>V \text{softmax} \left( \frac{Q^T K}{\sqrt{p}} \right) \quad \text{(n x n)}</math></center> | |||
* Dimensions: | |||
** <math>X</math> as a <math display=inline>d \times n</math> matrix, <math>n</math> as sequence length, and <math>d</math> as data dimensionality. | |||
** <math>Q</math>, <math>K</math> and <math>V</math> are the query, key, and value matrices, respectively, and they are derived from <math>W_Q</math>, <math>W_K</math> and <math>W_V</math>. | |||
** Given a sequence <math>X = \begin{bmatrix} x_1 & \cdots & x_n \end{bmatrix}_{d \times n}</math> we define: | |||
*** <math>Q = W_Q^T X \quad \text{(p x n)}, \quad W_Q^T \in \mathbb{R}^{p \times d}, \quad X \in \mathbb{R}^{d \times n}</math> | |||
*** <math>K = W_K^T X \quad \text{(p x n)}, \quad W_K^T \in \mathbb{R}^{p \times d}</math> | |||
*** <math>V = W_V^T X \quad \text{(m x n)}, \quad W_V^T \in \mathbb{R}^{m \times d}</math> | |||
==== Recap of Transformers ==== | |||
* Building Blocks of LLMs: Transformers. | |||
* Computational Complexity: | |||
** Issue: Transformers have quadratic complexity concerning sequence length. | |||
** Reason: Complexity arises due to calculations in the attention mechanism. | |||
==== Approaches to Reduce Complexity ==== | |||
* <b>Issue</b>: Long sequences result in an <math>n \times n</math> matrix, making computation demanding. | |||
* <b>Solution</b>: Techniques like the Performer model approximate attention, reducing complexity to linear time. | |||
=== Performer Model === | |||
==== Overview ==== | |||
* <b>Objective</b>: Address quadratic complexity in transformers by approximating attention using kernel methods. | |||
* <b>Kernel Approximation</b>: Utilizes random features to approximate kernels, e.g., Gaussian kernels. The kernel function <math>K(x, y)</math> is defined as: | |||
<center><math display=block>K_{\text{Gauss}}(x, y) = \varphi(x)^T \varphi(y)</math></center> | |||
* For the Gaussian kernel approximation, the transformation <math>\varphi_{\text{Gauss}}(x)</math> is given by: | |||
<center><math display=block>\varphi_{\text{Gauss}}(x) = \frac{1}{\sqrt{r}}\begin{pmatrix} \sin(\omega_1^T x), & \sin(\omega_2^T x), & \dots, & \sin(\omega_r^T x), \cos(\omega_1^T x), & \cos(\omega_2^T x), & \dots, & \cos(\omega_r^T x)\end{pmatrix}</math></center> | |||
* In general for kernel <math>K</math>: | |||
<center><math display=block>\phi(x) = \frac{h(x)}{\sqrt{ r }}(f_{1}(w_{1}^Tx) \dots f_{1}(w_{r}^Tx) \dots f_{l}(w_{1}^Tx) \dots f_{l}(w_{r}^Tx)) \quad \text{(l x r)}</math></center> | |||
==== Advantage ==== | |||
'''Scalability''': Performers are highly scalable for very long sequences. | |||
'''Memory Efficiency''': Performers drastically reduce memory usage by linearizing attention. | |||
'''Robust Performance''': Despite the approximation, Performers retain high accuracy and performance, comparable to standard transformers. | |||
==== Application ==== | |||
Performers are beneficial in applications requiring efficient processing of long sequences, such as natural language processing, DNA sequence analysis, and other domains where traditional transformers are computationally prohibitive. | |||
=== Softmax and Attention Mechanism in Transformers === | |||
==== Softmax Formula ==== | |||
* In the context of transformers, the softmax function is used to normalize the attention scores: | |||
<center><math display=block>\text{SM}[s]_i = \frac{e^{s_i}}{\sum_j e^{s_j}}</math></center> | |||
* To calculate the softmax numerically, we can consider: | |||
<center> | |||
<math>e^{Q^T K} = A</math> | |||
</center> | |||
<center> | |||
<math>d = \begin{bmatrix} | |||
& & & & \\ | |||
& & & & \\ | |||
& & & & | |||
\end{bmatrix}_{n \times n} | |||
\begin{bmatrix} | |||
1 \\ | |||
1 \\ | |||
\vdots \\ | |||
1 | |||
\end{bmatrix}_{n \times 1} \\</math> | |||
</center> | |||
<center> | |||
<math>D = \operatorname{diag}(d) = \begin{bmatrix} d_1 & 0 & \cdots & 0 \\ 0 & d_2 & \cdots & 0 \\ \vdots & \vdots & \ddots & \vdots \\ 0 & 0 & \cdots & d_n \end{bmatrix}_{n \times n}</math> | |||
</center> | |||
<center> | |||
<math>\operatorname{SM}[s]_i = \frac{e^{s_i}}{\sum_j e^{s_j}} = A D^{-1}</math> | |||
</center> | |||
* We want to be able to do the matrix multiplication in the following way, which has linear time complexity for long sequences in transformers: | |||
<center><math>\begin{bmatrix} | |||
V & \Phi^T & \Phi | |||
\end{bmatrix} | |||
\quad \text{with dimensions:} | |||
\quad | |||
\begin{bmatrix} | |||
m \times n & n \times r’ & r’ \times n | |||
\end{bmatrix} | |||
= m \times n</math></center> | |||
==== Steps in Kernel Approximation ==== | |||
* <b>Kernel Formulation</b>: Approximate <math>\phi(x)^T \phi(y)</math> as a function of random vectors. | |||
* <b>Random Feature Transformation</b>: Performers use sinusoidal functions (sine and cosine) as random features for approximating similarity between sequences. | |||
* The softmax can also be approximated using random features, with a specific <math>\varphi(x)</math> tailored for softmax. | |||
<center><math>e^{-\frac{1}{2}\lVert x - y \rVert^2} = e^{-\frac{(x - y)^T (x - y)}{2}} = e^{-\frac{x^T x + y^T y - 2 x^T y}{2}} = e^{-\frac{x^T x}{2}} \cdot e^{-\frac{y^T y}{2}} \cdot e^{x^T y}</math></center> | |||
<center><math>e^{x^T y} = e^{-\lVert x - y \rVert^2} \cdot e^{\frac{x^T x}{2}} \cdot e^{\frac{y^T y}{2}} = \varphi_{\text{Gauss}}(x)^T \varphi_{\text{Gauss}}(y) \cdot e^{\frac{x^T x}{2}} \cdot e^{\frac{y^T y}{2}} = \varphi_{\text{Gauss}}(x)^T e^{\frac{x^T x}{2}} \cdot \varphi_{\text{Gauss}}(y) e^{\frac{y^T y}{2}}</math></center> | |||
* So, the kernel approximation for the softmax is: | |||
<center><math>\varphi_{\text{SM}}(x) = \frac{e^{\frac{x^T x}{2}}}{\sqrt{r}} \begin{pmatrix} \sin(\omega_1^T x), & \sin(\omega_2^T x), & \dots, & \sin(\omega_r^T x), \cos(\omega_1^T x), & \cos(\omega_2^T x), & \dots, & \cos(\omega_r^T x)\end{pmatrix}</math></center> | |||
=== Variational Auto-encoders (VAEs) === | |||
* <b>Overview</b>: VAEs are a type of generative model that extends traditional auto-encoders by introducing stochastic elements. While traditional auto-encoders are deterministic and focus on reconstructing inputs, VAEs aim to learn a distribution over the latent space. This approach extends traditional autoencoders by introducing a probabilistic framework that enables them to capture complex data distributions. | |||
==== Background: Auto-encoders ==== | |||
* <b>Basic Structure</b>: Consists of an encoder, a bottleneck layer, and a decoder. | |||
* <b>Objective</b>: Maps input <math>x</math> into a lower-dimensional representation (<math>z = u^Tx</math>) by compressing it and reconstructs it. It minimizes the differences in <math>x</math> and the reconstructed version of it (<math>\hat{x} = uz</math>): <math>\min \lvert x - \hat{x} \rvert</math> | |||
* <b>PCA Connection</b>: A simple, linear auto-encoder resembles PCA in dimensionality reduction. | |||
* <b>Limitation</b>: While VAEs are powerful, they may struggle to capture highly complex data distributions compared to more recent generative models like GANs. | |||
==== Moving to Variational Auto-encoders ==== | |||
* <b>Generative Model Aspect</b>: Variational Auto-encoders enforce a specific distribution on <math>z</math>, allowing them to generate new samples. | |||
* <b>Gaussian Distribution Constraint</b>: By enforcing a Gaussian distribution on <math>z</math>, the model can generate new samples in the learned data distribution. So Variational Auto-encoder introduces a prior distribution (typically Gaussian) on <math>z</math> and maximizes the Evidence Lower Bound (ELBO) instead of reconstruction alone. | |||
==== Approximation of the Posterior Distribution ==== | |||
<center><math>p(z|x) = \frac{p(x|z) , p(z)}{p(x)}</math></center> | |||
<center><math>p(x) = \int_z p(x|z) , p(z) , dz</math></center> | |||
* <math>\mathbf{q_{\theta}(z)}</math> is the approximate posterior distribution of the latent variable <math>z</math>, parameterized by <math>\theta</math>. | |||
* <b>Purpose of</b> <math>\mathbf{q_{\theta}(z)}</math>: In a VAE, we aim to learn a latent variable <math>z</math> that represents the underlying structure of the data <math>x</math>. Ideally, we want the true posterior <math>p(z|x)</math>, which is often intractable to compute directly. To address this, we approximate <math>p(z|x)</math> with a simpler distribution <math>q_{\theta}(z|x)</math>, where <math>\theta</math> represents the parameters (typically learned through a neural network). | |||
* <b>Key Points about</b> <math>\mathbf{q_{\theta}(z)}</math>: | |||
** <b>Approximate Posterior</b>: <math>q_{\theta}(z|x)</math> is an approximation of the true posterior <math>p(z|x)</math>. | |||
** <b>Parameterized by</b> <math>\mathbf{\theta}</math>: The parameters <math>\theta</math> define the structure of this distribution, often through a neural network in a VAE. | |||
** <b>Optimization</b>: During training, we optimize <math>\theta</math> to make <math>q_{\theta}(z|x)</math> as close as possible to <math>p(z|x)</math> by minimizing the KL divergence between <math>q_{\theta}(z|x)</math> and <math>p(z|x)</math>: <math>\min_{\theta} \text{KL} \left( q_{\theta}(z) || p(z|x) \right)</math> | |||
==== Information Theory ==== | |||
In VAEs, the model learns a latent space where each data point is mapped not to a single point, but to a probability distribution. This design choice allows the VAE to: Capture the information content of the input data in a compressed, structured form. Represent the uncertainty in the data by encoding it as a distribution rather than as a single deterministic vector. The VAE learns this by balancing reconstruction accuracy with the information-theoretic regularization of the latent space, which enforces a smooth and organized representation. | |||
* <b>Information content</b>: | |||
** <math>I</math>, represents the information content or self-information of an event with probability <math>p(x)</math>. It quantifies how much “surprise” or “information” is associated with a specific outcome <math>x</math> occurring. | |||
** The formula for information content <math>I</math> of an event <math>x</math> is: <math>I = -\log p(x)</math> | |||
** <math>I</math> is higher for less probable events (low <math>p(x)</math>), indicating more “surprise” or “information” content when that event occurs. | |||
** In information theory, this concept helps quantify the amount of information gained from observing an event, with rare events providing more information than common ones. | |||
** For example, given a sentence "Tomorrow, it either rains, or not." <math>p(x) = 1 </math> and so <math> I = -log(1) = 0 </math> since there is no insight to glean in that sentence. | |||
* <b>Entropy</b>: | |||
** <math>H</math> represents entropy. Entropy measures the average amount of information (or “uncertainty”) contained in a random variable. It is often used to quantify the uncertainty or unpredictability of a probability distribution. | |||
** The formula for entropy <math>H</math> of a discrete random variable <math>x</math> with a probability distribution <math>p(x)</math> is: <math>H = -\sum p(x) \log p(x)</math> | |||
** <math>H</math> is maximized when the distribution is most uncertain (e.g., in a uniform distribution). | |||
** In the context of VAEs, entropy is used to understand the distribution of latent variables and how much “information” or “uncertainty” they contain. | |||
* <b>KL divergence</b>: | |||
** KL divergence, stands for the Kullback-Leibler divergence between two probability distributions <math>q</math> and <math>p</math>. It is a measure of how one probability distribution <math>q</math> diverges from a second, reference probability distribution <math>p</math>. | |||
** The KL divergence from <math>q(x)</math> to <math>p(x)</math> is defined as: <math>\text{KL}(q || p) = \sum q(x) \log q(x) - \sum q(x) \log p(x) = \sum_x q(x) \log \frac{q(x)}{p(x)}</math> | |||
** KL divergence is not symmetric; <math>\text{KL}(q \| p) \neq \text{KL}(p \| q)</math>. This means that it matters which distribution we consider as the reference. | |||
** KL divergence is always non-negative, <math>\text{KL}(q \| p) \geq 0</math>, and equals zero if and only if <math>q = p</math> (almost everywhere). This is known as Gibbs’ inequality. | |||
** In variational inference and variational auto-encoders (VAEs), KL divergence is used to measure the difference between the approximate posterior <math>q_{\theta}(z|x)</math> and the true posterior <math>p(z|x)</math>. Minimizing <math>\text{KL}(q_{\theta}(z|x) | p(z|x))</math> helps make the approximation <math>q_{\theta}(z|x)</math> closer to the true posterior. | |||
** KL divergence quantifies the “information loss” if we approximate <math>p(x)</math> with <math>q(x)</math>. In essence, it tells us how much extra information (or “surprise”) is needed to describe samples from <math>q</math> as if they were from <math>p</math>. | |||
** For the continuous space: | |||
<center><math>\text{KL}(q(z) \| p(z|x)) = -\int q(z) \log \frac{p(z|x)}{q(z)} \, dz</math></center> | |||
<center><math>p(z|x) = \frac{p(x|z) \, p(z)}{p(x)}</math></center> | |||
<center><math>p(x|z) \, p(z) = p(x,z)</math></center> | |||
<center><math>\text{KL}(q(z) \| p(z|x))= -\int q(z) \log \frac{p(x, z)}{q(z) \cdot p(x)} \, dz</math></center> | |||
<center><math>= -\int q(z) \left[ \log \frac{p(x, z)}{q(z)} + \log \frac{1}{p(x)} \right] dz</math></center> | |||
<center><math>= -\int q(z) \log \frac{p(x, z)}{q(z)} \, dz + \int q(z) \log p(x) \, dz = -\int q(z) \log \frac{p(x, z)}{q(z)} \, dz + \log p(x) \int q(z) \, dz</math></center> | |||
<center><math>\int q(z) \, dz = 1</math></center> | |||
<center><math>\log p(x) = \text{KL}(q(z) \| p(z|x)) + \int q(z) \log \frac{p(x, z)}{q(z)} \, dz = \text{KL}(q(z) \| p(z|x)) + \text{ELBO}</math></center> | |||
<center><math>\text{ELBO} = \int q(z) \log \frac{p(x, z)}{q(z)} \, dz</math></center> | |||
<center><math>= \int q(z) \log \frac{p(x|z) \, p(z)}{q(z)} \, dz</math></center> | |||
<center><math>= \int q(z) \left[ \log p(x|z) + \log \frac{p(z)}{q(z)} \right] dz</math></center> | |||
<center><math>= \int q(z) \log p(x|z) \, dz + \int q(z) \log \frac{p(z)}{q(z)} \, dz</math></center> | |||
<center><math>= \mathbb{E}_{q(z)}[\log p(x|z)] - \text{KL}(q(z) \| p(z))</math></center> | |||
<center><math>\text{ELBO} = \mathbb{E}_{q_{\theta}(z|x)}[\log p(x|z)] - \text{KL}(q_{\theta}(z|x) | p(z))</math></center> | |||
==== KL Divergence and Evidence Lower Bound (ELBO) ==== | |||
* <b>KL Divergence</b>: Measures the similarity between two distributions; minimized to bring <math>q</math> close to <math>p</math>. | |||
* <b>Evidence Lower Bound (ELBO)</b>: | |||
** Variational auto-encoders maximize ELBO which is similar to minimizing KL divergence since <math> log(p(x)) </math> is a constant. | |||
** Maximizing ELBO ensures <math>q</math> closely approximates <math>p</math>, meaning maximizing ELBO ensures that the learned latent distribution is close to the target distribution. This approach allows VAEs to generate new samples by sampling from the latent space. | |||
==== ELBO Decomposition ==== | |||
* <b>ELBO Equation</b>: <math>ELBO = q(z) log p(x|z) - KL[q(z) || p(z)]</math> | |||
* First Term: Reconstruction likelihood (maximize this). | |||
* Second Term: Ensures latent variable <math>z</math> approximates the desired distribution. | |||
==== Practical Implementation: Reconstruction Loss and Gaussian Constraint ==== | |||
* <b>Objective in VAE Training</b>: | |||
** Minimize reconstruction loss (similar to traditional auto-encoders). | |||
** Ensure <math>z</math> follows a Gaussian distribution. | |||
* <b>Regularization Term in ELBO</b>: In VAEs, the Evidence Lower Bound (ELBO) includes a KL divergence term to ensure that the learned latent distribution remains close to a prior distribution (e.g., a Gaussian <math>p(z) = N(0, I)</math>). | |||
==== Re-parameterization Trick ==== | |||
* <b>Challenge</b>: The stochastic nature of <math>z</math> complicates backpropagation. | |||
* <b>Solution</b>: To make the stochastic layer differentiable, VAEs use the re-parameterization trick, where <math>z</math> is expressed as a deterministic function with a noise component. So we use re-parameterization to enable gradient-based optimization and we minimize: | |||
<center><math>\min \left( \lvert x - \hat{x} \rvert^2 + \text{KL}(q(z) \| N(0, 1)) \right)</math></center> | |||
==== Reverse Processes ==== | |||
* We need to know the reverse diffusion process <math>p_{\theta}(x_{t-1}|x_t)=N(x_{t-1};\mu_{\theta}(x_t,t),\Sigma_{\theta}(x_t,t))</math> | |||
* Mean of q(x_{t-1}|x_t,x_0): | |||
<math>\begin{align} | |||
q(x_{t-1}|x_t,x_0) &= q(x_{t}|x_{t-1},x_0) \frac{q(x_{t-1}|x_0)}{q(x_t|x_0)} \\ | |||
&\propto \exp\left( | |||
-\frac{1}{2} | |||
\left( | |||
\frac{(x_t - \sqrt{\alpha_t}x_{t-1})^2}{\beta_t} | |||
+ | |||
\frac{(x_{t-1} - \sqrt{\bar{\alpha}_{t-1}}x_{0})^2}{1-\bar{\alpha}_{t-1}} | |||
- | |||
\frac{(x_t - \sqrt{\bar{\alpha}_{t}}x_{0})^2}{1-\bar{\alpha}_{t}} | |||
\right) | |||
\right)\\ | |||
&= \exp\left( | |||
-\frac{1}{2} | |||
\left( | |||
\left( | |||
\frac{\alpha_t}{\beta_t} | |||
+ | |||
\frac{1}{1-\bar{\alpha_{t-1}}} | |||
\right) | |||
x_{t-1}^2 | |||
- | |||
\left( | |||
\frac{2\sqrt{\alpha_t}}{\beta_t} | |||
x_t | |||
+ | |||
\frac{2\sqrt{\bar{\alpha_{t-1}}}}{1-\bar{\alpha_{t-1}}} | |||
x_0 | |||
\right) | |||
x_{t-1} | |||
+ | |||
C(x_t,x_0) | |||
\right) | |||
\right) | |||
\end{align} | |||
</math> | |||
, where <math>C(x_t,x_0)</math> is not a function of <math>x_{t-1}</math> | |||
Define <math>\alpha_t=1-\beta_t, \bar{\alpha_t}=\prod_{i=1}^T{\alpha_i}</math>, | |||
<math>\tilde{\mu_t}(x_t,x_0)=\frac{\sqrt{\alpha_t}(1-\bar{\alpha_{t-1}})}{1-\bar{\alpha_t}}x_t | |||
+ | |||
\frac{\sqrt{\bar{\alpha_{t-1}}}\beta_t}{1-\bar{\alpha_t}}x_0 | |||
</math> | |||
Since <math>x_0=\frac{1}{\sqrt{\bar{\alpha_t}}}(x_t-\sqrt{1-\bar{\alpha_t}}\epsilon_t)</math>, we have | |||
<math>\tilde{\mu_t}(x_t,x_0)=\frac{1}{\sqrt{\bar{\alpha_t}}}\left(x_t-\frac{1-\alpha_t}{\sqrt{1-\bar{\alpha_t}}}\epsilon_t\right)</math> | |||
* KL for two Gaussian | |||
* Loss function | |||
== Graph Neural Network (GNN) == | |||
Graph Neural Networks are a class of deep learning methods designed to perform inference on data described by graphs. | |||
=== Application === | |||
* '''Graphs are Structured Data''': Many real-world datasets can be represented as graphs. Examples: social networks, protein-interaction networks, the World Wide Web, and molecules, where entities and their relationships are mapped as nodes and edges. In social networks, each user can be represented as a node and the relationships or interactions between them — such as friendships and follows — are represented as edges connecting the nodes. GNN can be used to recommend friends and content by learning from the network structure. Also, GNN can help to track the spread of information or behaviors across the network. | |||
* '''Images as Graphs''': Images can be considered as graphs where each pixel acts as a node. In this representation, each pixel node connects to its adjacent pixels through edges and form a grid-like graph structure that captures the spatial relationships in the image. | |||
* '''Text as Graphs''': Text data can also be represented as graphs. Here, each token or word is a node, and each node is connected by edges to the preceding and following tokens, which can capture the sequential dependencies in the text. | |||
* '''CNN as GNN''': Convolutional Neural Networks (CNNs) can be viewed as a specialized form of Graph Neural Networks (GNNs) applied to grid-like structures. Each pixel in an image represents a node connected to its neighbors, with convolutions aggregating features across the whole grid. | |||
==== Tasks ==== | |||
There are 3 different levels of tasks. | |||
* '''Graph-Level Tasks''': These involve predicting a property of the entire graph. For example, in molecular graphs, we may predict whether a particular molecule will bind to a receptor based on the graph structure. | |||
* '''Node-Level Tasks''': These focus on predicting the identity or role of each individual node within the graph. This could involve classifying nodes based on their attributes or their position within the network. | |||
* '''Edge-Level Tasks''': These involve analyzing or predicting relationships between pairs of nodes, such as determining whether two nodes in a social network graph are friends or not. | |||
=== Graph === | |||
==== Definition ==== | |||
A graph, denoted as <math>G</math>, can be defined by a set of vertices (or nodes) <math>V</math> and edges (connections between the vertices) <math>V</math>, along with an adjacency matrix <math>A</math>. This matrix represents the relationships between vertices in a binary format. | |||
In graph notation, we write this as <math>G = (V, E, A)</math>, where: | |||
* '''<math>V</math>''': Vertices or nodes in the graph. | |||
* '''<math>E</math>''': Edges, which indicate connections between pairs of vertices. | |||
* '''<math>A</math>''': Adjacency matrix, a binary matrix that indicates the presence (1) or absence (0) of an edge between vertex pairs. | |||
The adjacency matrix is a binary matrix indicating whether pairs of vertices are adjacent and is structured so that: | |||
The entry <math>A_{ij} = 1</math> if there is an edge between vertex <math>i</math> and vertex <math>j</math>. | |||
The entry <math>A_{ij} = 0</math> if there is no edge between vertex <math>i</math> and vertex <math>j</math>. | |||
In this way, the adjacency matrix provides a clear view of the connections within the graph. | |||
==== Laplacian of a Graph ==== | |||
=====Laplacian Matrix Definition===== | |||
<math>L = D - A</math> | |||
<math>D</math>: Degree matrix, which is a diagonal matrix where each diagonal entry represents the degree of each vertex. | |||
<math>A</math>: Adjacency matrix, as previously defined. | |||
=====Degree Matrix Notation===== | |||
The degree matrix <math>D</math> is a square matrix in which each diagonal element <math>d_i</math> represents the degree of the corresponding vertex <math>i</math>. | |||
<math>D = \begin{bmatrix} d_1 & 0 & \dots & 0 \\ | |||
0 & d_2 & \dots & 0 \\ | |||
\vdots & \vdots & \ddots & \vdots \\ | |||
0 & 0 & \dots & d_n \end{bmatrix}_{n \times n}</math> | |||
Degree of a Vertex is defined as <math>d_i = \sum_j A_{ij}</math>. The degree <math>d_i</math> is the sum of the elements in the <math>i</math>-th row of <math>A</math>, representing the number of edges connected to vertex <math>i</math>. | |||
=====Example===== | |||
For an adjacency matrix <math>A</math> as follows: | |||
<math>A = \begin{bmatrix} 0 & 1 & 1 & 0 \\ 1 & 0 & 1 & 0 \\ 1 & 1 & 0 & 1 \\ 0 & 0 & 1 & 0 \end{bmatrix}</math> | |||
The sum of each row (representing each vertex's degree) results in: | |||
<math>\text{Sum} = \begin{bmatrix} \sum A_{1j} \\ \sum A_{2j} \\ \sum A_{3j} \\ \sum A_{4j} \end{bmatrix} = \begin{bmatrix} 2 \\ 2 \\ 3 \\ 1 \end{bmatrix}</math> | |||
This yields the degree matrix <math>D</math> as: | |||
<math>D = \begin{bmatrix} 2 & 0 & 0 & 0 \\ 0 & 2 & 0 & 0 \\ 0 & 0 & 3 & 0 \\ 0 & 0 & 0 & 1 \end{bmatrix}</math> | |||
The Laplacian matrix <math>L</math> is then calculated as: | |||
<math>L = D - A</math> | |||
==== Graph Cut Optimization Problem ==== | |||
The graph cut optimization problem aims to partition a graph into segments with minimal interconnections between them. | |||
* '''Objective''': The goal is to find a cut that divides the graph into distinct segments while minimizing the connections between them. | |||
* '''Minimization Target''': The target function to minimize is <math>\sum A_{ij} (y_i - y_j)^2</math>, which captures the cost associated with the "cut" between segments. | |||
* '''Equation''': The minimization can be expressed as <math>y^T L y</math>, where <math>L</math> is the Laplacian matrix, and <math>y</math> is a vector representing the segment assignment of each node. | |||
* '''Constraint Applied''': The constraint <math>y^T y = 1</math> is applied to ensure non-trivial solutions. | |||
* '''Vector <math>y</math>''': This vector represents the assignment of nodes to segments, with dimensions <math>n \times 1</math>, where <math>n</math> is the number of nodes. | |||
* '''Solution Method''': Optimal partitioning is achieved by performing eigen decomposition on the Laplacian matrix <math>L</math>. | |||
===== Eigen Decomposition of Laplacian ===== | |||
The Laplacian matrix <math>L</math> can be decomposed as <math>L = U \Lambda U^T</math>, where: | |||
<math>U</math> represents the orthonormal eigenvectors. These eigenvectors are both orthogonal to each other and normalized. | |||
<math>\Lambda</math> is a diagonal matrix containing the eigenvalues. Each diagonal entry in <math>\Lambda</math> corresponds to an eigenvalue that pairs with an eigenvector in <math>U</math>. | |||
Starting with the standard Laplacian, defined as <math>L = D - A</math>: | |||
<math>D</math> is the degree matrix. | |||
<math>A</math> is the adjacency matrix. | |||
The normalized Laplacian is given by: <math>\tilde{L} = D^{-\frac{1}{2}} (D - A) D^{-\frac{1}{2}}</math> | |||
This expression can be simplified to: <math>\tilde{L} = I - D^{-\frac{1}{2}} A D^{-\frac{1}{2}}</math>, where <math>I</math> is the identity matrix. | |||
===== Laplacian Eigenvectors and Fourier Analysis Analogy ===== | |||
* '''Mathematical Definition''': Decomposition of a signal into sinusoidal components. | |||
* '''Frequency Domain''': A signal is transformed to represent it as a sum of its frequency components. | |||
* '''Basis Functions''': Sine and cosine functions serve as the basis for this transformation. | |||
* '''Orthogonality''': These basis functions are orthogonal, ensuring unique frequency representation. | |||
* '''Graph Signals''': Functions defined over the nodes of a graph. | |||
* '''Spectral Domain''': Eigenvectors of <math>L</math> transform graph signals into the spectral domain. | |||
* '''Eigenvector Basis''': Analogous to sines and cosines, eigenvectors form a basis for graph signals. | |||
* '''Orthogonality''': Eigenvectors are orthogonal, providing a unique spectral representation for graph signals. | |||
* '''Low Eigenvalues''': Correspond to "low-frequency" eigenvectors. These eigenvectors change slowly over the graph. Represent large-scale, smooth structures in the graph. | |||
* '''High Eigenvalues''': Correspond to "high-frequency" eigenvectors. These eigenvectors change rapidly between connected nodes. Capture fine details or irregularities in the graph. | |||
* '''Ordering''': Eigenvalues (and eigenvectors) are ordered from lowest to highest. | |||
==== Fourier Transform ==== | |||
The eigen-decomposition of the graph Laplacian <math>L</math> can be written as: <math>L = U^T \Lambda U</math>, where: | |||
* <math>U = [u_1, ..., u_n]</math> is the matrix of orthonormal eigenvectors. | |||
* <math>\Lambda</math> is a diagonal matrix where each entry <math>\Lambda_{ii} = \lambda_i</math> represents an eigenvalue (also known as the spectrum of the Laplacian). | |||
* The equation <math>U^T U = I</math> indicates that the eigenvectors are orthonormal. | |||
The eigenvectors from <math>u_1</math> to <math>u_n</math> are also called Fourier functions, as they serve as a basis for transforming signals on the graph. | |||
The Fourier transform projects a signal <math>x</math> onto the Fourier functions, resulting in coefficients that form the Fourier series. | |||
In the context of graphs, the graph Fourier transform projects an input graph signal onto an orthonormal basis formed by the eigenvectors of the normalized graph Laplacian. | |||
Suppose <math>x \in \mathbb{R}^n</math> is a feature vector containing the values of all nodes in a graph, where <math>x_i</math> represents the value at the <math>i</math>-th node. | |||
* The graph Fourier transform of a signal <math>x</math> is given by: <math>f(x) = U^T x = \hat{x}</math> | |||
* The inverse graph Fourier transform is defined as: <math>f^{-1}(\hat{x}) = U \hat{x} = U U^T x = x</math> | |||
==== ConvGNNs ==== | |||
The graph convolution of an input signal <math>x</math> with a filter <math>g \in \mathbb{R}^n</math> is defined as follows: | |||
Convolution Theorem: <math>f(x * g) = (f(x) \cdot f(g))</math> | |||
This can be expanded as: | |||
* <math>x * g = f^{-1}(f(x) \cdot f(g))</math> | |||
* <math>= U (U^T x \cdot U^T g)</math> | |||
* <math>= U g_\theta U^T x</math> | |||
Where <math>g_\theta = \text{diag}(U^T g)</math>. | |||
===== Neural Network Layers ===== | |||
* Feedforward Neural Networks (FFNN): | |||
Computation proceeds in layers, with each layer represented as <math>h' = \sigma(Wh)</math>. The output of each layer <math>h'</math> serves as the input for the next layer. The initial input <math>h_0</math> is the feature vector <math>x</math>. | |||
* Convolutional Neural Networks (CNN): | |||
Layer computation involves convolution, with each layer defined as <math>h' = \sigma(w * h)</math>. <math>w</math> represents the learned filters or kernels that slide over <math>h</math>. | |||
==== Vanilla Spectral GNN ==== | |||
The graph convolutional layer of the Spectral CNN is defined as: | |||
<math>x * g = f^{-1}(f(x) \cdot f(g))</math> | |||
<math>= U (U^T x \cdot U^T g)</math> | |||
<math>= U g_\theta U^T x</math> | |||
With: | |||
* <math>H' = \sigma(U \Theta U^T H)</math> | |||
* <math>X = H^{(0)}</math> | |||
* <math>g_\theta = \Theta</math> | |||
Here, <math>\Theta</math> is a diagonal matrix with learnable parameters, where <math>U^T g = \begin{bmatrix} u_1^T g \ \vdots \ u_n^T g \end{bmatrix}</math> and <math>\Theta = \begin{bmatrix} u_1^T g & \dots & u_n^T g \end{bmatrix}</math>. | |||
Limitation: Eigen-decomposition requires <math>O(n^2)</math> computational complexity. | |||
==== ChebNet ==== | |||
Approximates the filter <math>g_\theta</math> by Chebyshev [*] polynomials of the | |||
diagonal matrix of eigenvalues <math>\Lambda</math>. | |||
===== Chebyshev Polynomials of the first kind ===== | |||
* <math>T_0(x) = 1</math> | |||
*<math>T_1(x) = x</math> | |||
* For <math>i \geq 2</math>, the polynomials are defined as: <math>T_i(x) = 2x T_{i-1}(x) - T_{i-2}(x)</math> | |||
===== ChebNet Graph Convolution ===== | |||
*<math>g_{\theta}=\sum_{i}{\theta_i{T_i}(\tilde{\Alpha}})</math>. | |||
*<math>g_{\theta}</math> is a Graph convolutional filter with parameter <math>\theta</math>. | |||
*<math>\tilde{\Alpha}</math> is are scaled eigenvalues of the Laplacian matrix: <math>\tilde{\Alpha}=\frac{2\Alpha}{\lambda_{max}}-I_n</math>, which makes the normalized eigenvalues fall into [-1,1]. | |||
*<math>x*{g}=Ug_{\theta}U^Tx</math> | |||
*<math>x*{g}=\sum_{i}\theta_iUT_i(\tilde{\Alpha})U^Tx</math> | |||
*<math>x*{g_{\theta}}=U\left(\sum_{i}\theta_iT_i(\tilde{\Alpha})\right)U^Tx</math> | |||
*This is equal to: <math>x*{g_{\theta}}=\sum_{i=1}^k{\theta_iT_i(\tilde{L})x}</math>, where <math>\tilde{L}=\frac{2L}{\lambda_{max}}-I_n</math> |
Latest revision as of 16:47, 21 November 2024
Regularization in Deep Learning
Introduction
Regularization is a fundamental concept in machine learning, particularly in deep learning, where models with a high number of parameters are prone to overfitting. Overfitting occurs when a model learns the noise in the training data rather than the underlying distribution, leading to poor generalization on unseen data. Regularization techniques aim to constrain the model’s capacity, thus preventing overfitting and improving generalization. This chapter will explore various regularization methods in detail, complete with mathematical formulations, intuitive explanations, and practical implementations.
Classical Regularization: Parameter Norm Penalties
L2 Regularization (Weight Decay)
L2 Parameter Regularization (Weight Decay)
Overview
L2 parameter regularization, commonly known as weight decay, is a technique used to prevent overfitting in machine learning models by penalizing large weights. This penalty helps in constraining the model's complexity.
The regularization term is given by:
[math]\displaystyle{ \mathcal{R}(w) = \frac{\lambda}{2} \|w\|_2^2 }[/math]
where:
- [math]\displaystyle{ \lambda }[/math] is the regularization strength (a hyperparameter),
- [math]\displaystyle{ w }[/math] represents the model weights,
- [math]\displaystyle{ \|w\|_2 }[/math] denotes the L2 norm of the weight vector.
Gradient of the Total Objective Function
The gradient of the total objective function, which includes both the loss and the regularization term, is given by:
[math]\displaystyle{ \nabla_w \mathcal{L}_{\text{total}}(w; X, y) = \lambda w + \nabla_w \mathcal{L}(w; X, y) }[/math]
The weight update rule with L2 regularization using gradient descent is:
[math]\displaystyle{ w := w - \eta (\lambda w + \nabla_w \mathcal{L}(w; X, y)) }[/math]
where [math]\displaystyle{ \eta }[/math] is the learning rate.
Quadratic Approximation to the Objective Function
Consider a quadratic approximation to the objective function:
[math]\displaystyle{ \mathcal{L}(w) \approx \mathcal{L}(w^*) + \frac{1}{2} (w - w^*)^\top H (w - w^*) }[/math]
where:
- [math]\displaystyle{ w^* }[/math] is the optimum weight vector,
- [math]\displaystyle{ H }[/math] is the Hessian matrix of second derivatives.
The modified gradient equation becomes:
[math]\displaystyle{ \lambda w + H (w - w^*) = 0 }[/math]
Solving for [math]\displaystyle{ w }[/math], we get:
[math]\displaystyle{ w = (H + \lambda I)^{-1} H w^* }[/math]
where [math]\displaystyle{ I }[/math] is the identity matrix.
Eigenvalue Decomposition
Assume [math]\displaystyle{ H = Q \Lambda Q^\top }[/math] where [math]\displaystyle{ Q }[/math] is the orthogonal matrix of eigenvectors and [math]\displaystyle{ \Lambda }[/math] is the diagonal matrix of eigenvalues.
Then the weight vector can be expressed as:
[math]\displaystyle{ w = Q(\Lambda + \lambda I)^{-1} \Lambda Q^\top w^* }[/math]
The effect of weight decay is to rescale the coefficients of the eigenvectors. The [math]\displaystyle{ i }[/math]-th component is rescaled by a factor of [math]\displaystyle{ \frac{\lambda_i}{\lambda_i + \lambda} }[/math], where [math]\displaystyle{ \lambda_i }[/math] is the [math]\displaystyle{ i }[/math]-th eigenvalue.
- If [math]\displaystyle{ \lambda_i \gt \lambda }[/math], the effect of regularization is relatively small.
- Components with [math]\displaystyle{ \lambda_i \lt \lambda }[/math] will be shrunk to have nearly zero magnitude.
Effective Number of Parameters
Directions along which the parameters contribute significantly to reducing the objective function are preserved. A small eigenvalue of the Hessian indicates that movement in this direction will not significantly increase the gradient.
The effective number of parameters can be defined as:
[math]\displaystyle{ \text{Effective Number of Parameters} = \sum_i \frac{\lambda_i}{\lambda_i + \lambda} }[/math]
As [math]\displaystyle{ \lambda }[/math] increases, the effective number of parameters decreases, which reduces the model's complexity.
(Placeholder for Image) (Include an image illustrating the effect of weight decay on the eigenvalues and the effective number of parameters)
Dataset Augmentation
Overview
Dataset augmentation is a technique used to improve the generalization ability of machine learning models by artificially increasing the size of the training dataset. This is particularly useful when the amount of available data is limited. The idea is to create new, synthetic data by applying various transformations to the original dataset.
- Key Idea: The best way to make a machine learning model generalize better is to train it on more data. When the amount of available data is limited, creating synthetic data (e.g., by applying transformations like rotation, translation, and noise addition) and adding it to the training set can be effective.
- Practical Example: Operations like translating training images a few pixels in each direction can greatly improve generalization. Another approach is to train neural networks with random noise applied to their inputs, which also serves as a form of dataset augmentation. This technique can be applied not only to the input layer but also to hidden layers, effectively performing dataset augmentation at multiple levels of abstraction.
---
Noise Injection
Overview
Noise injection is a regularization strategy that can be applied in two main ways:
1. Adding Noise to the Input: This method can be interpreted as a form of dataset augmentation and also has a direct connection to traditional regularization methods. 2. Adding Noise to the Weights: This method is primarily used in the context of recurrent neural networks and can be viewed as a stochastic implementation of Bayesian inference over the weights.
Mathematical Proof for Injecting Noise at the Input
Consider a regression setting where we have an input-output pair \( (x, y) \) and the goal is to minimize the expected loss function:
[math]\displaystyle{ J = \mathbb{E}_{x, y} \left[(f(x) - y)^2\right] }[/math]
Now, suppose we inject noise into the input \( x \), where the noise \( \epsilon \) is drawn from a distribution with mean zero (e.g., Gaussian noise \( \epsilon \sim \mathcal{N}(0, \sigma^2) \)). The modified objective function with noise-injected inputs becomes:
[math]\displaystyle{ J_{\text{noise}} = \mathbb{E}_{x, y, \epsilon} \left[(f(x + \epsilon) - y)^2\right] }[/math]
To understand the effect of noise injection, we can expand the function \( f(x + \epsilon) \) around \( x \) using a Taylor series:
[math]\displaystyle{ f(x + \epsilon) = f(x) + \epsilon^\top \nabla_x f(x) + \frac{1}{2} \epsilon^\top \nabla_x^2 f(x) \epsilon + \mathcal{O}(\|\epsilon\|^3) }[/math]
Since the expectation of the noise \( \epsilon \) is zero:
[math]\displaystyle{ \mathbb{E}[\epsilon] = 0 }[/math]
and assuming that the noise is isotropic with covariance matrix \( \sigma^2 I \), the expectation of the second-order term becomes:
[math]\displaystyle{ \mathbb{E}[\epsilon \epsilon^\top] = \sigma^2 I }[/math]
Substituting the Taylor expansion into the objective function:
[math]\displaystyle{ J_{\text{noise}} = \mathbb{E}_{x, y} \left[(f(x) - y)^2\right] + \frac{\sigma^2}{2} \mathbb{E}_{x, y} \left[\|\nabla_x f(x)\|^2\right] + \mathcal{O}(\sigma^4) }[/math]
This shows that the objective function with noise injection is equivalent to the original objective function plus a regularization term that penalizes large gradients of the function \( f(x) \). Specifically, the added term [math]\displaystyle{ \frac{\sigma^2}{2} \mathbb{E}_{x, y} \left[\|\nabla_x f(x)\|^2\right] }[/math] reduces the sensitivity of the network's output to small variations in its input.
Key Result:
For small noise variance \( \sigma^2 \), the minimization of the loss function with noise-injected input is equivalent to minimizing the original loss function with an additional regularization term that penalizes large gradients:
[math]\displaystyle{ J_{\text{noise}} \approx J + \frac{\sigma^2}{2} \mathbb{E}_{x, y} \left[\|\nabla_x f(x)\|^2\right] }[/math]
This regularization term effectively reduces the sensitivity of the output with respect to small changes in the input \( x \), which is beneficial in avoiding overfitting.
Connection to Weight Decay:
In linear models, where \( f(x) = w^\top x \), the gradient \( \nabla_x f(x) \) is simply the weight vector \( w \). Therefore, the regularization term becomes:
[math]\displaystyle{ \frac{\sigma^2}{2} \|w\|^2 }[/math]
which is equivalent to L2 regularization or weight decay.
Manifold Tangent Classifier
Overview
The Manifold Tangent Classifier (MTC) is a classification technique that leverages the idea that data often lies on a lower-dimensional manifold within the high-dimensional input space. The key assumption is that examples on the same manifold share the same category, and the classifier should be invariant to local factors of variation that correspond to movements on the manifold.
- Key Idea: The classifier should be invariant to variations along the manifold while being sensitive to changes that move the data off the manifold.
Tangent Propagation Algorithm
One approach to achieve invariance to manifold variations is to use the Tangent-Prop algorithm (Simard et al., 1992). The main idea is to add a penalty to the loss function that encourages the neural network's output to be locally invariant to known factors of variation. This is achieved by requiring the gradient of the output with respect to the input to be orthogonal to the known manifold tangent vectors \( v_i \) at each point \( x \).
The regularization term can be expressed as:
[math]\displaystyle{ \text{Regularizer} = \lambda \sum_{i} \left(\frac{\partial f(x)}{\partial x} \cdot v_i \right)^2 }[/math]
where:
- \( \frac{\partial f(x)}{\partial x} \) is the gradient of the neural network output with respect to the input,
- \( v_i \) are the known tangent vectors of the manifold,
- \( \lambda \) is the regularization strength.
This regularization ensures that the directional derivative of \( f(x) \) in the directions \( v_i \) is small, promoting invariance along the manifold.
Manifold Tangent Classifier (MTC)
A more recent approach, introduced by Rifai et al. (2011), eliminates the need to know the tangent vectors a priori. The Manifold Tangent Classifier automatically learns these tangent vectors during training, making it more flexible and applicable to a wider range of problems.
---
Early Stopping as a Form of Regularization
Overview
Early stopping is one of the most commonly used forms of regularization in deep learning. Instead of running the optimization algorithm until it reaches a local minimum of the training error, early stopping involves monitoring the validation error during training and halting the process when the validation error stops improving.
- Key Idea: During training, whenever the error on the validation set improves, a copy of the model parameters is stored. The training is stopped when the validation error has not improved for a predetermined amount of time, and the best model parameters (those that resulted in the lowest validation error) are returned.
Mathematical Formulation
Assume that \( w \) represents the model weights (ignoring bias parameters). We take a quadratic approximation to the objective function \( J(w) \) around the empirically optimal value of the weights \( w^* \):
[math]\displaystyle{ J(w) \approx J(w^*) + \frac{1}{2} (w - w^*)^\top H (w - w^*) }[/math]
where:
- \( H \) is the Hessian matrix of second derivatives.
The gradient of the objective function is:
[math]\displaystyle{ \nabla_w J(w) = H(w - w^*) }[/math]
During training, the parameter vector is updated according to:
[math]\displaystyle{ w^{(t+1)} = w^{(t)} - \eta \nabla_w J(w^{(t)}) }[/math]
Substituting the expression for the gradient:
[math]\displaystyle{ w^{(t+1)} - w^* = (I - \eta H) (w^{(t)} - w^*) }[/math]
where \( \eta \) is the learning rate. If we assume that the initial weights are zero (i.e., \( w^{(0)} = 0 \)), we can express the weight update after \( t \) iterations as:
[math]\displaystyle{ w^{(t)} - w^* = (I - \eta H)^t (w^{(0)} - w^*) }[/math]
If we perform an eigenvalue decomposition of \( H \), we get:
[math]\displaystyle{ H = Q \Lambda Q^\top }[/math]
where \( Q \) is the orthogonal matrix of eigenvectors, and \( \Lambda \) is the diagonal matrix of eigenvalues. The weight update can then be rewritten as:
[math]\displaystyle{ w^{(t)} - w^* = Q (I - \eta \Lambda)^t Q^\top (w^{(0)} - w^*) }[/math]
Assuming \( w^{(0)} = 0 \) and that \( |1 - \eta \lambda_i| < 1 \) for all eigenvalues \( \lambda_i \), after \( t \) training updates, we have:
[math]\displaystyle{ Q^\top w^{(t)} \approx [I - (1 - \eta \Lambda)^t] Q^\top w^* }[/math]
Taking the logarithm and using the series expansion for \( \log(1 + x) \), it can be shown that the number of training iterations \( t \) plays a role inversely proportional to the L2 regularization parameter \( \lambda \), and the inverse of \( t \) plays the role of the weight decay coefficient.
Key Insight:
This result shows that early stopping can be interpreted as a form of implicit regularization, where the number of training iterations controls the effective complexity of the model.
Early Stopping as a Form of Regularization
Overview
Early stopping is one of the most commonly used forms of regularization in deep learning. Instead of running the optimization algorithm until it reaches a local minimum of the training error, early stopping involves monitoring the validation error during training and halting the process when the validation error stops improving.
- Key Idea: During training, whenever the error on the validation set improves, a copy of the model parameters is stored. The training is stopped when the validation error has not improved for a predetermined amount of time, and the best model parameters (those that resulted in the lowest validation error) are returned.
Mathematical Formulation
Assume that \( w \) represents the model weights (ignoring bias parameters). We take a quadratic approximation to the objective function \( J(w) \) around the empirically optimal value of the weights \( w^* \):
[math]\displaystyle{ J(w) \approx J(w^*) + \frac{1}{2} (w - w^*)^\top H (w - w^*) }[/math]
where:
\( H \) is the Hessian matrix of second derivatives.
The gradient of the objective function is:
[math]\displaystyle{ \nabla_w J(w) = H(w - w^*) }[/math]
During training, the parameter vector is updated according to:
[math]\displaystyle{ w^{(t+1)} = w^{(t)} - \eta \nabla_w J(w^{(t)}) }[/math]
Substituting the expression for the gradient:
[math]\displaystyle{ w^{(t+1)} - w^* = (I - \eta H) (w^{(t)} - w^*) }[/math]
where \( \eta \) is the learning rate. If we assume that the initial weights are zero (i.e., \( w^{(0)} = 0 \)), we can express the weight update after \( t \) iterations as:
[math]\displaystyle{ w^{(t)} - w^* = (I - \eta H)^t (w^{(0)} - w^*) }[/math]
If we perform an eigenvalue decomposition of \( H \), we get:
[math]\displaystyle{ H = Q \Lambda Q^\top }[/math]
where \( Q \) is the orthogonal matrix of eigenvectors, and \( \Lambda \) is the diagonal matrix of eigenvalues. The weight update can then be rewritten as:
[math]\displaystyle{ w^{(t)} - w^* = Q (I - \eta \Lambda)^t Q^\top (w^{(0)} - w^*) }[/math]
Assuming \( w^{(0)} = 0 \) and that \( |1 - \eta \lambda_i| < 1 \) for all eigenvalues \( \lambda_i \), after \( t \) training updates, we have:
[math]\displaystyle{ Q^\top w^{(t)} \approx [I - (1 - \eta \Lambda)^t] Q^\top w^* }[/math]
Taking the logarithm and using the series expansion for \( \log(1 + x) \), it can be shown that the number of training iterations \( t \) plays a role inversely proportional to the L2 regularization parameter \( \lambda \), and the inverse of \( t \) plays the role of the weight decay coefficient.
Key Insight:
This result shows that early stopping can be interpreted as a form of implicit regularization, where the number of training iterations controls the effective complexity of the model.
Label Smoothing
Label smoothing is a technique to prevent a model from becoming over-confident on a specific class by not forcing the model to fit the data exactly. This approach provides more flexibility and generalization abilities.
Suppose the predicted output is [math]\displaystyle{ y = [0, 1, 0] }[/math], then after applying label smoothing, it becomes [math]\displaystyle{ y = [0.033, 0.933, 0.033] }[/math].
The formula for label smoothing is:
[math]\displaystyle{ y_{\text{smooth}} = (1 - a) \cdot y + \frac{a}{k} }[/math]
where:
- [math]\displaystyle{ a }[/math] is the smoothing factor,
- [math]\displaystyle{ y }[/math] is the original label,
- [math]\displaystyle{ k }[/math] is the number of classes.
The reason for using label smoothing:
- Prevents overfitting: By preventing the model from becoming too confident in its predictions, label smoothing reduces the likelihood of overfitting.
- Improves generalization: Label smoothing can help the model generalize better to unseen data, as it discourages overconfidence in training.
Bagging/Ensemble
Bagging (short for bootstrap aggregating) is a machine learning ensemble technique for reducing generalization error by combining several models (Breiman, 1994)
Explanation: Aggregating is to ombine the predictions of each model, often using voting (for classification) or averaging (for regression).
It works by training several different models separately, and then have all of the models vote on the output for test examples. For example, random forest, which is a popular bagging algorithm that builds multiple decision trees on different bootstrap samples of the data and aggregates their predictions.
The reason why bagging works:
- Variance reduction: By training multiple models on different data subsets, bagging reduces the variance of the predictions. This means that it helps models generalize better to new, unseen data.
- Handling overfitting: Bagging is particularly useful for high-variance models (e.g., decision trees) as it helps reduce overfitting.
While bagging is highly useful for traditional machine learning models, it is less commonly used in deep learning because modern neural networks are already highly expressive. Instead, other ensemble methods, such as model ensembling or dropout, are used.
Code Sample:
from sklearn.ensemble import RandomForestClassifier
from sklearn.datasets import load_iris
from sklearn.model_selection import train_test_split
- Load a dataset (Iris dataset for example) data = load_iris()
X_train, X_test, y_train, y_test = train_test_split(data.data, data.target, test_size=0.3, random_state=42)
- Train a random forest classifier (bagging technique)
rf_model = RandomForestClassifier(n_estimators=100, random_state=42)
rf_model.fit(X_train, y_train)
- Make predictions
y_pred = rf_model.predict(X_test)
- Evaluate the model
accuracy = rf_model.score(X_test, y_test)
Dropout
Overview
Dropout is one of the techniques for preventing overfitting in deepneural network which contains a large number of parameters.
The key idea is to randomly drop units from the neural networkduring training.
- During training, dropout samples from number of different “thinned” network.
- At test time, we approximate the effect of averaging the predictions of all these thinned networks
Model
Consider a neural network with [math]\displaystyle{ L }[/math] hidden layers:
- Let [math]\displaystyle{ z^{(l)} }[/math] denote the vector inputs into layer [math]\displaystyle{ l }[/math].
- Let [math]\displaystyle{ y^{(l)} }[/math] denote the vector of outputs from layer [math]\displaystyle{ l }[/math].
- Let [math]\displaystyle{ W^{(l)} }[/math] and [math]\displaystyle{ b^{(l)} }[/math] represent the weights and biases at layer [math]\displaystyle{ l }[/math].
With dropout, the feed-forward operation becomes:
[math]\displaystyle{ r^{(l)} \sim \text{Bernoulli}(p) }[/math]
[math]\displaystyle{ y^{(l)} = r^{(l)} \odot y^{(l)} }[/math]
where [math]\displaystyle{ \odot }[/math] denotes element-wise multiplication.
The feed-forward equation for layer [math]\displaystyle{ l+1 }[/math] becomes:
[math]\displaystyle{ z^{(l+1)} = W^{(l+1)} y^{(l)} + b^{(l+1)} }[/math]
[math]\displaystyle{ y^{(l+1)} = f(z^{(l+1)}) }[/math]
where [math]\displaystyle{ f }[/math] is the activation function.
For any layer [math]\displaystyle{ l }[/math], [math]\displaystyle{ r^{(l)} }[/math] is a vector of independent Bernoulli random variables, each of which has a probability [math]\displaystyle{ p }[/math] of being 1. The vector [math]\displaystyle{ y^{(l)} }[/math] is the input after some hidden units are dropped. The rest of the model remains the same as a regular feed-forward neural network.
Training
Dropout neural network can be trained using stochastic gradientdescent. The only difference here is that we only back propagate on eachthinned network. The gradient for each parameter are averaged over the training cases in each mini-batch.
Test Time
Use a single neural net without dropout If a unit is retained with probability p during training, the outgoing weights of that unit are multiplied by p at test time. [math]\displaystyle{ p \cdot w }[/math]
Additional Regularization
L1 norm
L1 norm regularization, also known as Lasso, is a technique that adds a penalty equal to the absolute value of the magnitude of coefficients to the loss function. This encourages sparsity in the learned weights, meaning it forces some of the weights to become exactly zero, effectively selecting important features and reducing model complexity.
Mixup
Mixup is a data augmentation technique that creates new training examples by taking convex combinations of pairs of input data and their labels. By blending images and labels together, the model learns smoother decision boundaries and becomes more robust to adversarial examples and noise.
Cutout
Cutout is a form of data augmentation where random square regions are masked out (set to zero) in input images during training. This forces the model to focus on a broader range of features across the image rather than relying on any single part, leading to better generalization and robustness.
Gradient Clipping
Gradient clipping is a technique used to prevent the gradients from becoming too large during training, which can cause the model to diverge. This is done by capping the gradients at a predefined threshold, ensuring that updates remain stable, especially in models like recurrent neural networks (RNNs) where exploding gradients are a common issue.
DropConnect
DropConnect is a variation of Dropout, but instead of dropping neurons, it randomly drops connections (weights) between neurons during training. This prevents the co-adaptation of neurons while allowing individual neurons to contribute to learning.
Data Augmentation (beyond Mixup and Cutout)
- Random Flips and Rotations: Randomly flipping (either vertical or horizontal) or rotating images during training to make the model invariant to certain transformations.
- Color Jittering: Modifying the brightness, contrast, saturation, and hue of images to make the model more robust to variations in color.
- Random Cropping and Scaling: Randomly cropping and scaling images to force the model to learn from different perspectives and contexts within the data.
For PyTorch augmentation, one can refer https://pytorch.org/vision/stable/transforms.html
Generalization Paradox
- Models with many parameters tend to overfit
- However, deep neural network, despite using many parameters, works well with unseen data (look up the Double Descent Curve), the reason remains unknown
This phenomenon is illustrated by the Double Descent Curve, where after reaching a peak in test error (due to overfitting), the error decreases again with further model complexity. The precise reasons remain uncertain, but hypotheses include implicit regularization from optimization methods like SGD, hierarchical feature learning, and the redundancy offered by overparameterization.
Batch Normalization
Overview
Batch normalization is a technique used to improve the training process of deep neural networks by normalizing the inputs of each layer. Despite the initial intuition for the method being somewhat incorrect, it has proven to be highly effective in practice. Batch normalization speeds up convergence, allows for larger learning rates, and makes the model less sensitive to initialization, resulting in more stable and efficient training.
Batch normalization motivated by internal covariate shift (2015 lofee & Szegedy)
Internal Covariance Shift
Batch normalization was originally proposed as a solution to the internal covariance shift problem, where the distribution of inputs to each layer changes during training. This shift complicates training because the model must constantly adapt to new input distributions.
The transformation of layers can be described as:
[math]\displaystyle{ l = F_2(F_1(u, \theta_1), \theta_2) }[/math]
For a mini-batch of activations [math]\displaystyle{ X = \{x_1, x_2, \dots, x_m\} }[/math] from a specific layer, batch normalization proceeds as follows:
1. Compute the mean: [math]\displaystyle{ \mu_B = \frac{1}{m} \sum_{i=1}^{m} x_i }[/math]
2. Compute the variance: [math]\displaystyle{ \sigma_B^2 = \frac{1}{m} \sum_{i=1}^{m} (x_i - \mu_B)^2 }[/math]
3. Normalize the activations: [math]\displaystyle{ \hat{x}_i = \frac{x_i - \mu_B}{\sqrt{\sigma_B^2 + \epsilon}} }[/math]
4. Scale and shift the normalized activations using learned parameters [math]\displaystyle{ \gamma }[/math] (scale) and [math]\displaystyle{ \beta }[/math] (shift): [math]\displaystyle{ y_i = \gamma \hat{x}_i + \beta }[/math]
where:
- [math]\displaystyle{ x_i }[/math] represents the activations in the mini-batch,
- [math]\displaystyle{ \mu_B }[/math] is the mean of the mini-batch,
- [math]\displaystyle{ \sigma_B^2 }[/math] is the variance of the mini-batch,
- [math]\displaystyle{ \epsilon }[/math] is a small constant added for numerical stability,
- [math]\displaystyle{ \hat{x}_i }[/math] is the normalized activation,
- [math]\displaystyle{ \gamma }[/math] and [math]\displaystyle{ \beta }[/math] are learned parameters for scaling and shifting.
The batch normalization improves validation accuracy by removing the dropout and enables higher learning rate
Batch Normalization
As mentioned earlier, the original intuition behind batch normalization was found to be incorrect after further research. A paper by Santurkar, S., Tsipras, D., Ilyas, A., & Madry, A. (NeurIPS 2019) contradicted the original 2015 paper on BatchNorm by highlighting the following points:
- Batch normalization does not fix covariate shift.
- If we fix covariate shift, it doesn't help.
- lf we intentionally increase lCS, it doesn't harm.
- Batch Norm is not the only possible normalization. There are alternatives.
Instead, they argue that Batch normalization works better due to other factors, particularly related to its effect on the optimization process:
1. Reparameterization of the loss function:
- Improved Lipschitzness: Batch normalization improves the Lipschitz continuity of the loss function, meaning that the loss changes at a smaller rate, and the magnitudes of the gradients are smaller. This makes the gradients of the loss more "Lipschitz."
- Better β-smoothness: The loss exhibits significantly better smoothness, which aids in optimization by preventing large, erratic changes in the gradient.
2. Variation of the loss function: BatchNorm reduces the variability of the value of the loss. Consider the variation of the loss function: [math]\displaystyle{ \mathcal{L}(x + \eta \nabla \mathcal{L}(x)) }[/math] where [math]\displaystyle{ \eta \in [0.05, 0.4] }[/math]. A smaller variability of the loss indicates that the steps taken during training are less likely to cause the loss to increase uncontrollably.
3. Gradient predictiveness: BatchNorm enhances the predictiveness of the gradients, meaning the changes in the loss gradient are more stable and predictable. This can be expressed as: [math]\displaystyle{ || \nabla \mathcal{L}(x) - \nabla \mathcal{L}(x + \eta \nabla \mathcal{L}(x)) || }[/math], where [math]\displaystyle{ \eta \in [0.05, 0.4] }[/math]. A good gradient predictiveness implies that the gradient evaluated at a given point remains relevant over longer distances, which allows for larger step sizes during training.
Alternatives to Batch Norm
Weight Normalization: Weight normalization is a technique where the weights, instead of the activations, are normalized. This method reparameterizes the weight vectors to accelerate the training of deep neural networks.
Tim Salimans and Diederik P. Kingma, "Weight Normalization: A Simple Reparameterization to Accelerate Training of Deep Neural Networks," 2016.
ELU (Exponential Linear Unit) and SELU (Scaled Exponential Linear Unit): ELU and SELU are two proposed activation functions that have a decaying slope instead of a sharp saturation. They can be used as alternatives to BatchNorm by providing smoother, non-linear activation without requiring explicit normalization.
Djork-Arné Clevert, Thomas Unterthiner, and Sepp Hochreiter, "Fast and Accurate Deep Network Learning by Exponential Linear Units (ELUs)," In International Conference on Learning Representations (ICLR), 2016. Günter Klambauer, Thomas Unterthiner, Andreas Mayr, and Sepp Hochreiter, "Self-Normalizing Neural Networks," ICLR, 2017.
Convolutional Neural Network (CNN)
Introduction
Convolutional networks are simply neural networks that use convolution instead of general matrix multiplication in at least one of their layers. CNN is mainly used for image processing.
Convolution
In ML, convolution means dot product
[math]\displaystyle{ h = \sigma(\langle x, w\rangle+b) }[/math]
- Same x, different w -- multi-layer perception (MLP)
- Different x, same w -- CNN (weight sharing)
From class, the following operation is called convolution
[math]\displaystyle{ s(t) = \int x(a)w(t-a)ds }[/math]
The convolution operation is typically denoted with an asterisk:
[math]\displaystyle{ s(t) = (x \ast w)(t) }[/math]
Discrete Convolution
If we now assume that x and w are defined only on integer t, we can define the discrete convolution:
[math]\displaystyle{ s[t] = (x \ast w)(t) = \sum_{a=-\infty}^{\infty} x[a] \, w[t-a] }[/math]
[math]\displaystyle{ w[t-a] }[/math] represents the sequence [math]\displaystyle{ w[t] }[/math] shifted by [math]\displaystyle{ a }[/math] units.
In practice
We often use convolutions over more than one axis at a time.
[math]\displaystyle{ s[i,j] = (I * K)[i,j] = \sum_m \sum_n I[m,n] K[i-m, j-n] }[/math]
- Input: usually a multidimensional array of data.
- Kernel: usually a multidimensional array of parameters that should be learned.
We assume that these functions are zero everywhere but the finite set of points for which we store the values.
We can implement the infinite summation as a summation over a finite number of array elements.
Convolution and Cross-Correlation
Convolution is commutative:
[math]\displaystyle{ s[i,j] = (I * K)[i,j] = \sum_m \sum_n I[i-m, j-n] K[m, n] }[/math]
Cross-correlation:
[math]\displaystyle{ s[i,j] = (I * K)[i,j] = \sum_m \sum_n I[i+m, j+n] K[m, n] }[/math]
Many machine learning libraries implement cross-correlation but call it convolution. In the context of backpropagation in neural networks, cross-correlation simplifies the computation of gradients with respect to the input and kernel.
Visualization of Cross-Correlation and Convolution with Matlab (https://www.youtube.com/v/Ma0YONjMZLI)
Image to Convolved Feature
- Kernel\filter size: weight [math]\displaystyle{ \times }[/math] height, e.g. 3 [math]\displaystyle{ \times }[/math] 3 in below example
- Stride: how many pixels to move the filter each time
- Padding: add zeros (or any other value) around the boundary of the input
Example
The following image illustrates a 2D convolution operation between an input image and a filter to produce a convolved feature map.
Image (Input): The grid on the left represents a 5[math]\displaystyle{ \times }[/math]5 matrix of pixel values. The orange-highlighted 3[math]\displaystyle{ \times }[/math]3 region is part of the image currently being convolved with the filter.
Convolution Operation: The filter values are applied to the selected region in an element-wise multiplication followed by a summation. The operation is as follows:
[math]\displaystyle{ (1 \times 1) + (1 \times 0) + (1 \times 1) + (0 \times 0) + (1 \times 1) + (1 \times 0) + (0 \times 1) + (0 \times 0) + (1 \times 1) = 4 }[/math]
Convolved Feature Map: The result value [math]\displaystyle{ 4 }[/math], is placed in the corresponding position (top-left) of the convolved feature map on the right.
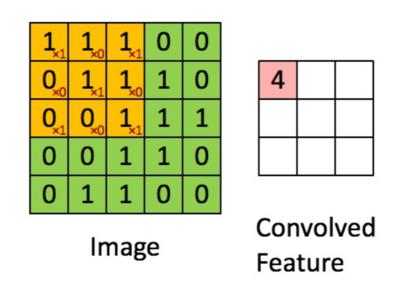
This process is repeated as the filter slides across the entire image. The final feature map is shown below.
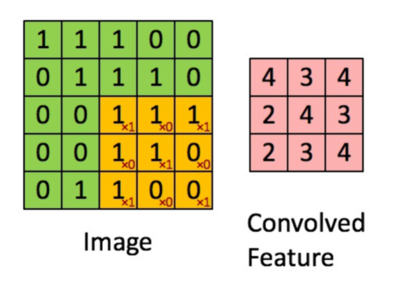
Sparse Interactions
In feed forward neural network every output unit interacts with every input unit.
- When we have [math]\displaystyle{ m }[/math] inputs and [math]\displaystyle{ n }[/math] outputs, then matrix multiplication requires [math]\displaystyle{ (m \times n) }[/math] parameters. and the algorithms used in practice have [math]\displaystyle{ O(m \times n) }[/math] runtime (per example)
Convolutional networks, typically have sparse connectivity (sparse weights). This is accomplished by making the kernel smaller than the input.
- Limit the number of connections each output may have to [math]\displaystyle{ k }[/math], then requires only [math]\displaystyle{ (k \times n) }[/math] parameters and [math]\displaystyle{ O(k \times n) }[/math] runtime
Parameter Sharing
- In a traditional neural net, each element of the weight matrix is multiplied by one element of the input. i.e. It is used once when computing the output of a layer.
- In CNNs, each member of the kernel is used at every position of the input
- Instead of learning a separate set of parameters for every location, we learn only one set
Equivariance
A function [math]\displaystyle{ f(x) }[/math] is equivaraint to a function [math]\displaystyle{ g }[/math] is the following holds:
[math]\displaystyle{ f(g(x)) = g(f(x)) }[/math]
In simple terms: Applying the function [math]\displaystyle{ f }[/math] after [math]\displaystyle{ g }[/math] is equivalent to applying [math]\displaystyle{ g }[/math] after [math]\displaystyle{ f }[/math]
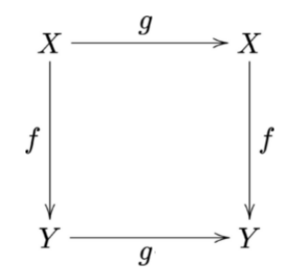
Equivariance in CNNs
- CNNs are naturally equivariant to translation (Covlution = Shift)
- If an input image is shifted, the output feature map shifts correspondingly, preserving spatial structure
- Importance: This property ensures that CNNs can detect features like edges or corners, no matter where they appear in the image
A convolutional layer has equivariance to translation. For example,
[math]\displaystyle{ g(x)[i] = x[i-1] }[/math]
If we apply this transformation to x, then apply convolution, the result will be the same as if we applied convolution to x, then applied the transformation to the output.
For images, convolution creates a 2-D map of where certain features appear in the input. Note that convolution is not equivariant to some other transformations, such as changes in the scale (rescaling) or rotation of an image.
Importance of Data Augmentation
Data augmentation is commonly used to make CNNs robust against variations in scale, rotation, or other transformations. This involves artificially modifying the training data by applying transformations like flipping, scaling, and rotating to expose the network to different variations of the same object.
Convolutional Networks
The first stage (Convolution): The layer performs several convolutions in parallel to produce a set of preactivations. The convolution stage is designed to detect local features in the input image, such as edges and patterns. It does this by applying filters/kernels across the input image.
The second stage (Detector): Each preactivation is run through a nonlinear activation function (e.g. rectified linear). This stage introduces nonlinearity into the model, enabling it to learn complex patterns. Without this nonlinearity, the model would be limited to learning only linear relationships.
The third stage (Pooling) Pooling reduces the spatial dimensions of the feature maps (height and width), helping to make the model more invariant to small translations and distortions in the input image. It also reduces computational load and helps prevent overfitting.
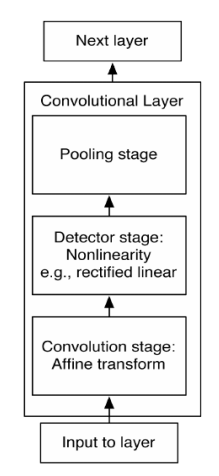
Pooling
Down-sample input size to reduce computation and memory
Popular Pooling functions
- The maximum of a rectangular neighborhood (Max pooling operation)
- The average of a rectangular neighborhood
- The L2 norm of a rectangular neighborhood
- A weighted average based on the distance from the central pixel
Pooling with Downsampling
Max-pooling with a pool width of 3 and a stride between pools of 2. This reduces the representation size by a factor of 2, which reduces the the computational and statistical burden on the next layer.
Pooling and Translations
Pooling helps to make the representation become invariant to small translations of the input. Invariance to local translation can be a very useful property if we care more about whether some feature is present than exactly where it is. For example: In a face, we need not know the exact location of the eyes.
Input of Varying Size
Example: we want to classify images of variable size.
The input to the classification layer must have a fixed size. In the final pooling output (for example) four sets of summary statistics, one for each quadrant of an image, regardless of the image size. Feature after pooling is [math]\displaystyle{ 2 \times 2 }[/math].
It is also possible to dynamically pool features together, for example, by running a clustering algorithm on the locations of interesting features (Boureau et al., 2011).
i.e. a different set of pooling regions for each image.
Learn a single pooling structure that is then applied to all images (Jia et al., 2012)
Convolution and Pooling as an Infinitely Strong Prior
- Weak Prior: a prior distribution that has high entropy, which means there is a high level of uncertainty or spread in the distribution. An example of this would be a Gaussian distribution with high variance
- Strong Prior: has very low entropy, which implies a high level of certainty or concentration in the distribution. An example of this would be a Gaussian distribution with low variance
- Infinitely Strong Prior: places zero probability on some parameters and says a convolutional net is similar to a fully connected net with an infinitely strong prior over its weights
The weights for one hidden unit must be identical to the weights of its neighbor, but shifted in space. The weights must be zero, except for in the small, spatially contiguous receptive field assigned to that hidden unit.
Use of convolution as infinitely strong prior probability distribution over the parameters of a layer. This prior says that the function the layer should learn contains only local interactions and is equivariantto translation.
The use of pooling is infinitely strong prior that each unit should be invariant to small translations. Convolution and pooling can cause underfitting.
Practical Issues
The input is usually not just a grid of real values. It is a grid of vector-valued observations. For example, a color image has a red, green, and blue intensity at each pixel.
When working with images, we usually think of the input and output of the convolution as 3-D tensors. One index into the different channels and two indices into the coordinates of each channel.
Software implementations usually work in batch mode, so they will actually use 4-D tensors, with the fourth axis indexing different examples in the batch.
Training
Suppose we want to train a convolutional network that incorporates convolution of kernel stack [math]\displaystyle{ K }[/math] applied to multi-channel image [math]\displaystyle{ V }[/math] with stride [math]\displaystyle{ s }[/math]:[math]\displaystyle{ c(K; V; s) }[/math]
Suppose we want to minimize some loss function [math]\displaystyle{ J(V; K) }[/math]. During forward propagation, we will need to use [math]\displaystyle{ c }[/math] itself to output [math]\displaystyle{ Z }[/math].
[math]\displaystyle{ Z }[/math] is propagated through the rest of the network and used to compute [math]\displaystyle{ J }[/math].
- During backpropagation, we receive a tensor [math]\displaystyle{ \mathbf{G} }[/math] such that:
[math]\displaystyle{ G_{i,j,k} = \frac{\partial}{\partial Z_{i,j,k}} J(V, K) }[/math]
- To train the network, we compute the derivatives with respect to the weights in the kernel:
[math]\displaystyle{ g(\mathbf{G}, \mathbf{V}, s)_{i,j,k,l} = \frac{\partial}{\partial Z_{i,j,k}} J(V, K) = \sum_{m,n} G_{i,m,n} V_{j,ms+k,ns+l} }[/math]
- If this layer is not the bottom layer of the network, we compute the gradient with respect to [math]\displaystyle{ \mathbf{V} }[/math] to backpropagate the error further:
[math]\displaystyle{ h(\mathbf{K}, \mathbf{G}, s)_{i,j,k} = \frac{\partial}{\partial V_{i,j,k}} J(V, K) = \sum_{l,m \lvert s l + m = j} \sum_{n,p \lvert s n + p = k} \sum_{q} K_{q,i,m,p} G_{i,l,n} }[/math]
Random or Unsupervised Features
The most computationally expensive part of training a convolutional network is learning the features.
- Supervised training with gradient descent requires full forward and backward propagation through the entire network for every gradient update.
- One approach to reduce this cost is to use features that are not learned in a supervised manner, such as random or unsupervised features.
Random Initilizations
Simply initialize the convolution kernels randomly. In high-dimension space, random vectors are almost orthogonal to each other (correlated). Features captured by different kernels are independent.
Unsupervised Learning
Learn the convolution kernels using an unsupervised criterion.
Key insight
Random filters can perform surprisingly well in convolutional networks.
- Layers composed of convolution followed by pooling naturally become frequency-selective and translation-invariant, even with random weights.
Inexpensive architecture selection
- Evaluate multiple convolutional architectures by training only the last layer.
- Choose the best-performing architecture and then fully train it using a more intensive method.
Residual Networks (ResNet)
Overview
Deeper models are harder to train due to vanishing/exploding gradients and can be worse than shallower networks if not properly trained. There are advanced networks to deal with the degradation problem.
ResNet, short for Residual Networks, was introduced by Kaiming He et al. from Microsoft Research in 2015. It brought a significant breakthrough in deep learning by enabling the training of much deeper networks, addressing the vanishing gradient problem.
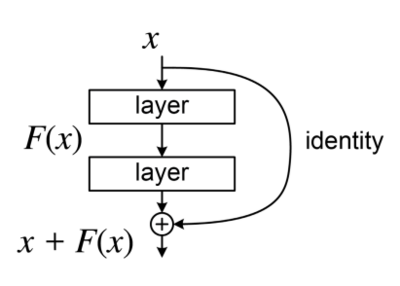
- ResNet introduces the concept of skip connections (or residual connections) that allow the gradient to be directly backpropagated to earlier layers
- Skip connections help in overcoming the degradation problem, where the accuracy saturates and then degrades rapidly as the network depth increases
Variants
Several variants of ResNet have been developed, including ResNet-50, ResNet-101, and ResNet-152, differing in the number of layers.
Application
ResNet has been widely adopted for various computer vision tasks, including image classification, object detection, and facial recognition.
DenseNet
Overview
- DenseNet, short for Densely Connected Networks, was introduced by Gao Huang et al. in 2017.
- It is known for its efficient connectivity between layers, which enhances feature propagation and reduces the number of parameters
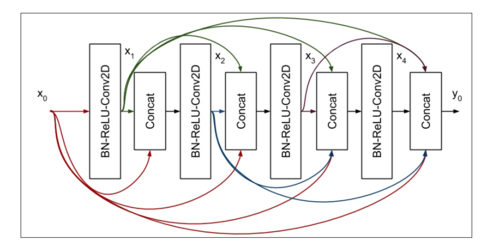
Key Feature
The key feature is Dense Connectivity.
- In DenseNet, each layer receives feature maps from all preceding layers and passes its own feature maps to all subsequent layers
- This dense connectivity improves the flow of information and gradients throughout the network, mitigating the vanishing gradient problem
Echo State Network
In RNNs, the ability to capture long-term dependencies is crucial. Set the recurrent and input weights such that the recurrent hidden units do a good job of capturing the history of past inputs, and only learn the output weights. The goal is to access the information from the past implicitly.
The hidden state at time [math]\displaystyle{ t }[/math] can be expressed as:
[math]\displaystyle{ s_t = \sigma(W s_{t-1} + U x_t) }[/math]
where:
- [math]\displaystyle{ W }[/math] represents the recurrent weight matrix, which connects previous hidden states to the current state,
- [math]\displaystyle{ U }[/math] is the input weight matrix, responsible for incorporating the current input [math]\displaystyle{ x_t }[/math],
- [math]\displaystyle{ \sigma }[/math] is an activation function, like a non-linear function such as a sigmoid or tanh.
It is important to control how small changes in the hidden state propagate through time to ensure the network does not become unstable.
If a change [math]\displaystyle{ \Delta s }[/math] in the state at time [math]\displaystyle{ t }[/math] is aligned with an eigenvector [math]\displaystyle{ v }[/math] of the Jacobian [math]\displaystyle{ J }[/math] with eigenvalue [math]\displaystyle{ \lambda \gt 1 }[/math], then the small change [math]\displaystyle{ \Delta s }[/math] becomes [math]\displaystyle{ \lambda \Delta s }[/math] after one-time step, and [math]\displaystyle{ \lambda^t \Delta s }[/math] after [math]\displaystyle{ t }[/math] time steps.
If the largest eigenvalue [math]\displaystyle{ \lambda \lt 1 }[/math], the map from [math]\displaystyle{ t }[/math] to [math]\displaystyle{ t+1 }[/math] is contractive.
The network forgets information about the long-term past.
Set the weights to make the Jacobians slightly contractive. This allows the network to gradually forget irrelevant information while still remembering key long-term dependencies.
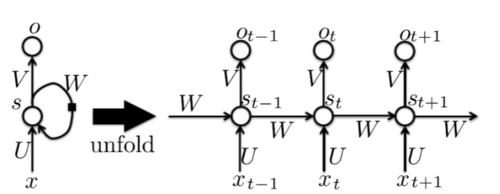
Long Delays
RNNs often fail to capture these dependencies due to the vanishing gradient problem. Long delays use recurrent connections. It knows something from the past, help vanishing gradient - even if gradient get vanished during the path, it still has the direct information from the past.
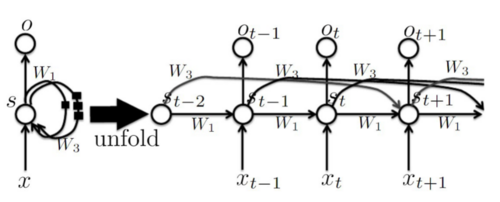
Leaky Units
In some cases, we do need to forget the path while in some we do not since we do not want to remember redundant information.
Recall that:
[math]\displaystyle{ s_t = \sigma(W s_{t-1} + U x_t) }[/math]
Then consider the following refined form of the equation (convex combination of the current state and previous through a new parameter):
[math]\displaystyle{ s_{t,i} = \left(1 - \frac{1}{\tau_i}\right) s_{t-1} + \frac{1}{\tau_i} \sigma(W s_{t-1} + U x_t) }[/math]
where
- [math]\displaystyle{ 1 \leq \tau_i \leq \infty }[/math]
- [math]\displaystyle{ \tau_i = 1 }[/math] corresponds to an ordinary RNN
- [math]\displaystyle{ \tau_i \gt 1 }[/math] allows gradients to propagate more easily
- [math]\displaystyle{ \tau_i \gg 1 }[/math] means the state changes very slowly, integrating past values associated with the input sequence over a long duration
Infinity means the current state is the previous state while one means completely forgetting the previous steps and only depends on the current observations.
Gated RNNs
Defnition
It might be useful for the neural network to forget the old state in some cases like if we only care about if the current letter is a or b.
Example: [math]\displaystyle{ a\ a\ b\ b\ b\ b\ a\ a\ a\ a\ b\ a\ b }[/math]
It might be useful to keep the memory of the past.
Example:
Instead of manually deciding when to clear the state, we want the neural network to learn to decide when to do it.
Long-Short-Term-Memory (LSTM)
The Long-Short-Term-Memory (LSTM) algorithm was proposed in 1997 (Hochreiter and Schmidhuber, 1997). It is a type of recurrent neural network designed for approaching the vanishing gradient problem.
Several variants of the LSTM are found in the literature:
- Hochreiter and Schmidhuber, 1997
- Graves, 2012
- Graves et al., 2013
- Sutskever et al., 2014
The principle is always to have a linear self-loop through which gradients can flow for a long duration.
Gated Recurrent Units (GRU)
Here is the plain text version of the new image content:
Recent work on gated RNNs, Gated Recurrent Units (GRU), was proposed in 2014.
- Cho et al., 2014
- Chung et al., 2014, 2015
- Jozefowicz et al., 2015
- Chrupala et al., 2015
Standard RNN computes the hidden layer at the next time step directly:
[math]\displaystyle{ s_t = \sigma(W s_{t-1} + U x_t) }[/math]
There are two gates: the update gate and the reset gate. Update gate for the case that we want to keep the information around while reset gate is the case when forgetting. A temporary state locks down some of the values of the current state.
GRU first computes an update gate (another layer) based on the current input vector and hidden state:
[math]\displaystyle{ z_t = \sigma(U^{(z)} x_t + W^{(z)} s_{t-1}) }[/math]
It also computes the reset gate similarly but with different weights:
[math]\displaystyle{ r_t = \sigma(U^{(r)} x_t + W^{(r)} s_{t-1}) }[/math]
New memory content is calculated as:
[math]\displaystyle{ \tilde{s_t} = \tanh(U x_t + r_t \odot W s_{t-1}) }[/math]
which has current observations and forgetting something from the past
If the reset gate is close to 0, this causes the network to ignore the previous hidden state, effectively allowing the model to drop irrelevant information.
The final memory at time step [math]\displaystyle{ t }[/math] is a combination of the current and previous time steps:
[math]\displaystyle{ s_t = z_t \odot s_{t-1} + (1 - z_t) \odot \tilde{s_t} }[/math]
If the reset gate is close to 0, it will ignore the previous hidden state, allowing the model to discard irrelevant information.
The update gate [math]\displaystyle{ z_t }[/math] controls how much of the past state should matter in the current time step. If [math]\displaystyle{ z_t }[/math] is close to 1, then we can effectively copy information from the past state across multiple time steps.
Units that need to capture short-term dependencies often have highly active reset gates.
Cliping Gradients
A simple solution for clipping the gradient. (Mikolov, 2012; Pascanu et al., 2013):
- Clip the parameter gradient from a mini-batch element-wise (Mikolov, 2012) just before the parameter update.
- Clip the norm [math]\displaystyle{ g }[/math] of the gradient [math]\displaystyle{ g }[/math] (Pascanu et al., 2013a) just before the parameter update.
The formula for clipping the gradient is:
[math]\displaystyle{ g' = \min\left(1, \frac{c}{|g|}\right) g }[/math]
where c is a constant.
Attention
The attention mechanism was introduced to improve the performance of the encoder-decoder model for machine translation.
Common Representation
A single 'concept' is universally represented, transcending specific languages or forms.
- Encoder: Processes the word 'elephant' from its original source.
- Output: A universal representation vector (the abstract 'concept' of an elephant).
- Decoders: Translate this concept into various domains or applications.
The 'concept' is an abstract entity that exists independently of any particular language or representation.
For example, if we want to translate from English to Spanish
- Encoder (English Input): The system processes the word "elephant."
- Output (Universal Representation): The system generates an abstract concept or vector representing an "elephant," independent of any specific language.
- Decoder (Spanish Output): The system decodes this concept and outputs the equivalent Spanish word: "elefante."
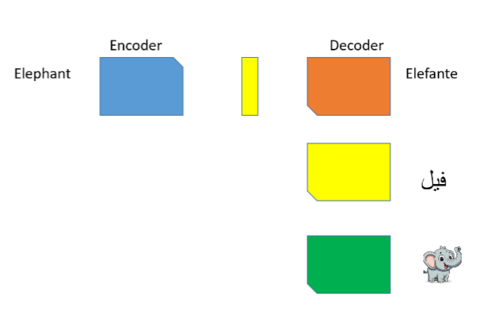
Sequence-to-Sequence Model
In the sequence-to-sequence model, every word [math]\displaystyle{ x_i }[/math] produces a hidden vector [math]\displaystyle{ h_i }[/math] in the encoder part of the autoencoder. The hidden vector of every word, [math]\displaystyle{ h_i }[/math], is fed to the next hidden vector, [math]\displaystyle{ h_{i+1} }[/math], by a projection matrix [math]\displaystyle{ W }[/math].
In this model, for the whole sequence, there is only one context vector [math]\displaystyle{ c }[/math], which is equal to the last hidden vector of the encoder, i.e., [math]\displaystyle{ c = h_n }[/math].
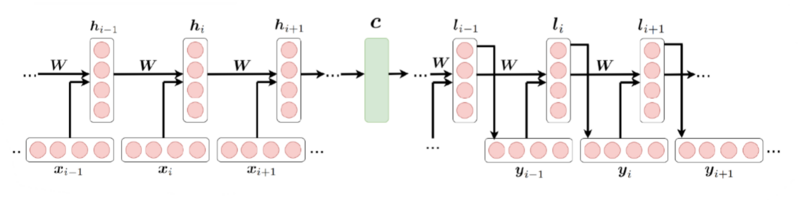
Challenges:
1. Long-range dependencies: As the model processes long sequences, it can struggle to remember and utilize information from earlier steps, especially in cases where long-term context is crucial.
2. Sequential processing: Since these models process data step by step in sequence, they can't take full advantage of parallel processing, which limits the speed and efficiency of training.
These are the core challenges that newer architectures, such as transformers, aim to address.
Attention Definition
The basic idea behind the attention mechanism is directing the focus on important factors when processing data. Attention is a fancy name for weighted average.
Sequence-to-Sequence Model with Attention
- Sequence-to-sequence models:
Multiple RNN units serve as the encoder. They encode information into the context vectors. Multiple RNN units decode the concept in the context vector to different domain information. The limitations of this approach are long-range dependencies and prevention of parallelization.
[math]\displaystyle{ p(y_i | y_1, \ldots, y_{i-1}) = g(y_{i-1}, l_i, c) }[/math]
- Sequence-to-sequence with attention:
Pass multiple context vectors to the decoder.
[math]\displaystyle{ p(y_i | y_1, \ldots, y_{i-1}) = g(y_{i-1}, l_i, c_i) }[/math]
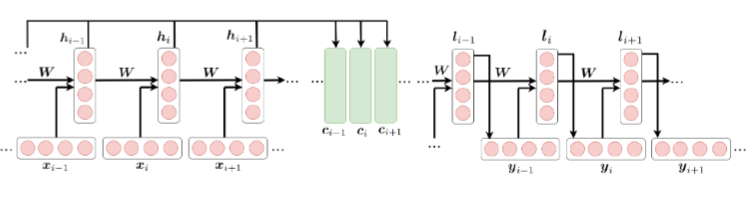
There are some calculations:
1. Similarity score: [math]\displaystyle{ s_{ij} = similarity(l_{i-1}, h_j) }[/math]
2. Attention weight: [math]\displaystyle{ a_{ij} = \frac{e^{s_{ij}}}{\sum_{k=1}^{T} e^{s_{ik}}} }[/math]
The attention weight [math]\displaystyle{ a_{ij} }[/math] is obtained by applying a softmax function to the similarity scores. This normalizes the scores across all encoder hidden states, turning them into a probability distribution.
3. Context vector: [math]\displaystyle{ c_i = \sum_{j=1}^{T} a_{ij} h_j }[/math]
The effectiveness of the correlation between inputs around position [math]\displaystyle{ j }[/math] and the output at position [math]\displaystyle{ i }[/math] is crucial.
This score is determined based on:
- The RNN hidden state [math]\displaystyle{ l_{i-1} }[/math] just before emitting [math]\displaystyle{ y_i }[/math].
- The [math]\displaystyle{ j^{th} }[/math] hidden state [math]\displaystyle{ h_j }[/math] of the input sentence.
Transformer Architecture
The basic concept behind transformers is attention as summarized in the paper by Vaswani et. al 'Attention is all you need'. This paper claims that all you need is attention and with the structure of attentions, basically you can handle the sequential data. It was based on GPT and many other models that we use in LLM and imaging processing. Transformer is an example of an encoder-decoder architecture. Unlike RNNs, Transformers can process sequences in parallel, making them faster to train on large datasets. Transformers have applications beyond NLP, such as Vision Transformers (ViT) in computer vision and protein structure prediction in biology (AlphaFold). Transformers' ability to capture long-range dependencies efficiently has made them the standard for many modern AI models.
Encoder
The encoder consists of two main components: Self Attention and Feed Forward Neural Network. The architecture of the Encoder is given below:
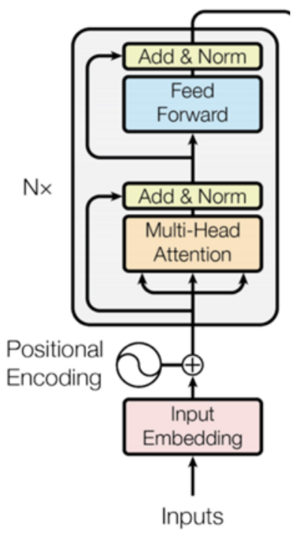
Self Attention
Understanding the individual words in a sentence is not enough to understand the whole sentence and one needs to understand how the words relate to each other. The attention mechanism forms composite representations. We aim to have embeddings of words and embeddings of compositions at the same time in different levels. Unlike word2vec introduced in 2013 by Mikolov et al., which captures the vector representations of words in an embedding space such that words that are similar are represented closer to each other, self-attention aims to capture the similarity between words based on context and in relation to each other. Multiple layers help form complex concept representations. For example, the context of the word "bank" differs based on if the surrounding words involve "money" or "river".
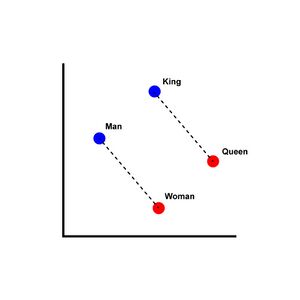
Self-attention is analogous to the fundamental retrieval strategy in databases where given a query, and key-value pairs, we use the query to identify the key and retrieve the corresponding value. The generalized definition for calculating the attention of a target word with respect to the input word: use the Query of the target and the Key of the input and then calculate a matching score. These matching scores act as the weights of the Value vectors.
Then we look at the definition if matrix form. Given an input vector [math]\displaystyle{ \mathbf{x} \in \mathbb{R}^d }[/math], the weights for the query, key, and value transformations are defined as:
- Query weight matrix: [math]\displaystyle{ \mathbf{W}_q \in \mathbb{R}^{d \times p} }[/math]
- Key weight matrix: [math]\displaystyle{ \mathbf{W}_k \in \mathbb{R}^{d \times p} }[/math]
- Value weight matrix: [math]\displaystyle{ \mathbf{W}_v \in \mathbb{R}^{d \times r} }[/math]
The transformations for the query ([math]\displaystyle{ \mathbf{q} }[/math]), key ([math]\displaystyle{ \mathbf{k} }[/math]), and value ([math]\displaystyle{ \mathbf{v} }[/math]) vectors are given by:
- Query vector: [math]\displaystyle{ \mathbf{q} = \mathbf{W}_q^T \mathbf{x} }[/math] where [math]\displaystyle{ \mathbf{q} \in \mathbb{R}^{p \times 1} }[/math], since [math]\displaystyle{ \mathbf{W}_q^T \in \mathbb{R}^{p \times d} }[/math], and [math]\displaystyle{ \mathbf{x} \in \mathbb{R}^{d \times 1} }[/math]
- Key vector: [math]\displaystyle{ \mathbf{k} = \mathbf{W}_k^T \mathbf{x} }[/math] where [math]\displaystyle{ \mathbf{k} \in \mathbb{R}^{p \times 1} }[/math], since [math]\displaystyle{ \mathbf{W}_k^T \in \mathbb{R}^{p \times d} }[/math], and [math]\displaystyle{ \mathbf{x} \in \mathbb{R}^{d \times 1} }[/math]
- Value vector: [math]\displaystyle{ \mathbf{v} = \mathbf{W}_v^T \mathbf{x} }[/math] where [math]\displaystyle{ \mathbf{v} \in \mathbb{R}^{r \times 1} }[/math], since [math]\displaystyle{ \mathbf{W}_v^T \in \mathbb{R}^{r \times d} }[/math], and [math]\displaystyle{ \mathbf{x} \in \mathbb{R}^{d \times 1} }[/math]
The transformations allow the attention mechanism to compute similarity scores between the query and key vectors and to use the value vectors to produce the final weighted output as [math]\displaystyle{ \mathbf{z_1} = \alpha_1 \mathbf{v}_1 + \alpha_2 \mathbf{v}_2 + \ldots + \alpha_n \mathbf{v}_n }[/math], where [math]\displaystyle{ \mathbf{v}_i }[/math] are similar to the input in CNNs, and [math]\displaystyle{ \alpha_i }[/math] are similar to the kernels in CNNs.
However, unlike the kernels in CNNs, the [math]\displaystyle{ \alpha_i }[/math]'s are data-dependent and given by: [math]\displaystyle{ \alpha_i = \text{softmax}\left(\frac{\mathbf{q}^T \mathbf{k}_i}{\sqrt{p}}\right) }[/math].
Extending this to the entire dataset, the equations are:
[math]\displaystyle{ \mathbf{X} = [\mathbf{x}_1, \mathbf{x}_2, \ldots, \mathbf{x}_n] \in \mathbb{R}^{d \times n} }[/math]
[math]\displaystyle{ \mathbf{Q} = [\mathbf{q}_1, \mathbf{q}_2, \ldots, \mathbf{q}_n] \in \mathbb{R}^{p \times n} }[/math]
[math]\displaystyle{ \mathbf{K} = [\mathbf{k}_1, \mathbf{k}_2, \ldots, \mathbf{k}_n] \in \mathbb{R}^{p \times n} }[/math]
[math]\displaystyle{ \mathbf{V} = [\mathbf{v}_1, \mathbf{v}_2, \ldots, \mathbf{v}_n] \in \mathbb{R}^{r \times n} }[/math]
The transformations are defined as:
[math]\displaystyle{ \mathbf{Q}_{p \times n} = \mathbf{W}_{q}^{T_{p \times d}} \mathbf{X}_{d \times n} }[/math]
[math]\displaystyle{ \mathbf{K}_{p \times n} = \mathbf{W}_{k}^{T_{p \times d}} \mathbf{X}_{d \times n} }[/math]
[math]\displaystyle{ \mathbf{V}_{r \times n} = \mathbf{W}_{v}^{T_{r \times d}} \mathbf{X}_{d \times n} }[/math]
Therefore the output, [math]\displaystyle{ \mathbf{Z}_{r \times n} = \mathbf{V}_{r \times n} \cdot \text{softmax}\left(\frac{\mathbf{Q}^{T} \mathbf{K}}{\sqrt{p}}\right)_{n \times n} }[/math]
Additionally, we have:
[math]\displaystyle{ \mathbf{Q}^{T} \mathbf{K} = \mathbf{X}^{T} \mathbf{W}_{q} \mathbf{W}_{k}^{T} \mathbf{X} \quad \text{(an asymmetric kernel extracting the global similarity between words)} }[/math]
Let us take a closer look at how one word is processed in the encoder.
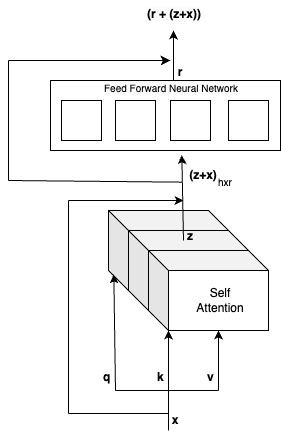
The input vector x is passed through a linear transformation layer which transforms the input into query q, key k, and value v vectors, given by the equations above. This is passed through a stacked multi-head self-attention layer which extracts global information across pairwise sequence of words to produce the output vector Z also given by the equation above. The equation for Z can be compared to how similarity is computed between the key and value pairs in databases. This output is added to the residual input x to preserve the meaning of individual words in addition to the pairwise representations. This is normalized to produce the output [math]\displaystyle{ \mathbf{(Z+x)} \in \mathbb{R}^{h \times r} }[/math]. This serves as the input to the feed forward neural network.
Feed Forward Neural Network
The structure of the feed forward network (FFN) is Linear Layer 1, followed by ReLU activation and then another linear layer 2. While the attention mechanism captures global information between words and hence aggregates across columns, the feed forward neural network aggregates across rows and takes a more broader look at each word independently. Depsite the individual processing, all positions share the same set of weights and biases in the FFN. In a classroom environment, the attention mechanism is similar to the teacher observing the interactions among students in a group, whereas the feed forward neural network resembles the teacher evaluating each student independently for their understanding of the assignment. The output of the feed forward layer r also has a residual connection from the previous layer which is normalized and passed as the input of the decoder as [math]\displaystyle{ \mathbf{(r+(z+x))} }[/math]. The encoder also has a positional encoding component which captures information about the position of words in a sequence.
While the above figure zooms in at how a word vector x is processed by the encoder, the major advantage of the transformer architecture is its ability to handle multiple words in a sequence in parallel and so in practice, the above zoomed in version of the encoder is usually stacked to handle a group of words in parallel.
==== Global v.s. Local Information
For Attention Mechanism:
- Global Understanding: Captures relationships among different positions in the sequence.
- Context Aggregation: Spreads relevant information across the sequence, enabling each position to see a broader context.
For Feed-Forward Networks (FFN):
- Local Processing: While attention looks across the entire sequence, FFN zooms back in to process each position independently.
- Individual Refinement: Enhances the representation of each position based on its own value, refining the local information gathered so far.
Decoder
The decoder consist of three main components: Masked Self Attention, Cross Attention and Linear Layer. The architecture of the Decoder is given below:
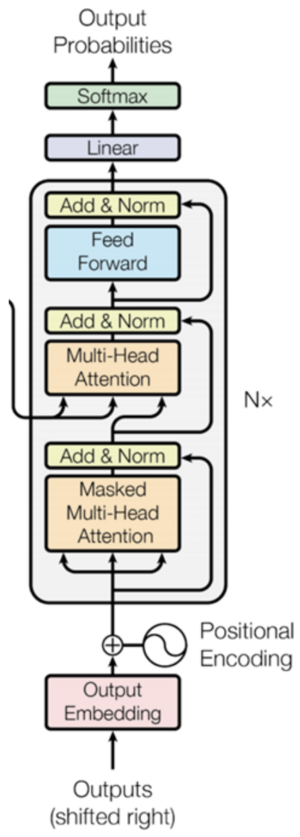
Masked Self Attention
In masked self attention, we add a mask matrix [math]\displaystyle{ \mathbf{M} \in \mathbb{R}^{n \times n} }[/math] to the normalized argument within the softmax of [math]\displaystyle{ \mathbf{Z} }[/math] given by [math]\displaystyle{ \mathbf{Z}_{r \times n} = \mathbf{V}_{r \times n} \cdot \text{softmax}\left(\frac{\mathbf{Q}^{T} \mathbf{K} + \mathbf{M}}{\sqrt{p}}\right)_{n \times n} }[/math] The reason for adding a mask matrix M is to ensure that the output is informed only by the past words and not by the words further along in the sequence. The mask matrix therefore is given by:
[math]\displaystyle{ M(i,j) = \begin{cases} 0 & \text{if } j \leq i \\ -\infty & \text{if } j \gt i \end{cases} }[/math]
This is because [math]\displaystyle{ \mathbf{Z} }[/math] is an upper triangular matrix with values for previous words since [math]\displaystyle{ softmax(x + 0) }[/math] is the same as [math]\displaystyle{ softmax(x) }[/math], but [math]\displaystyle{ softmax(x - \infty) }[/math] will be equal to 0.
Cross Attention
The intuition behind cross attention is similar to sequence-to-sequence models where the context vector is passed from the encoder to the decoder capturing relevant information from the input sequence. The residual connection plus the output of the masked self attention is passed as the query to the cross attention block, whereas the key and value pairs are the same as the output of the encoder. The relationship and relevance between words in different sentences are captured.
Linear Layer
The linear layer is applied to the output of the feed forward neural network of the decoder and its primary role is to adjust the dimensionality of the network to match the size of the vocabulary. It involves learning a set of parameters - a matrix of dimension equal to [math]\displaystyle{ p \times len(vocab) }[/math]. This results in a [math]\displaystyle{ p \times 1 }[/math] vector which is then passed through a probabilistic softmax layer to predict the next word in the sequence.
Softmax Activation
The function transforms the linear layer's output into probabilities as mentioned above. The output represents the likelihood of a respective word being the next word in the sequence.
Positional Encoding
So far, the encoder and decoder has no sense of the order of the words in the sequence. The sentences "I am a teacher", "Teacher I am", "Am I a teacher?" are processed the same way, even though the meaning may not be the same. To circumvent this issue, and due to the lack of the convolution or recurrence operations, a positional encoding scheme is embedded within the encoder and decoder. In this encoding, the position of the words in a sequence is encoded by a vector. The even positions are represented by a sinusoidal wave with 1 representing the peaks and 0 representing the troughs. Similarly, the odd positions are represented by a cosine wave. Thus, each position is encoded by a unique binary vector and each unique binary vector represents a specific position.
Bidirectional Encoder Representations from Transformers (BERT)
BERT introduced by Google is built by repeating the encoder of the transformer multiple times. The Bidirectional in BERT refers to its ability to attend to the future and past words in the sequence unlike Transformers which only looks at the past to make predictions about the future. The fundamental principles behind BERT are Masked Language Modeling, and Next Sentence Prediction. The architecture of BERT is given below.
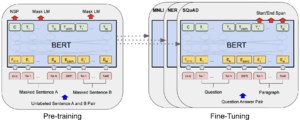
Masked Language Modeling
BERT masks words in a corpus as shown in the figure below and makes the model learn to predict these words. It thereby pays attention to both words before and after the masked word and fills in the blank given the entire context. It is the same as a Transformer Encoder where 12 encoders (in contrast to the 6 in the original paper of Transformers) are stacked on top of each other. The output of the encoder is passed to s linear dense layer and softmax to predict the most probable word from the vocabulary of the corpus.
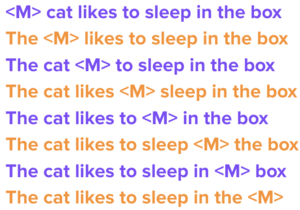
Next Sentence Prediction
It was also used to identify if given two sentences A and B, B logically follows the sentence A. This is possible due to the ability to fine-tune the weights of the pretrained BERT model for downstream prediction tasks. The pre-trained model can also be used to represent the input of a sentiment analysis task (for example) to a neural network in a better manner. The [CLS] token is prepended to the input sequence and is used to capture the context of the whole sentence such that during fine-tuning, the prediction may be conditioned on the representation of the [CLS] token.
There are various flavors of BERT based on slight modifications to the original architecture and training processes. The advantage of fine tuning pretrained models is the opportunity to finetune them on a domain specific corpus leading to additional variants of BERT as BioBERT retrained on a biomedical corpus, BERTweet trained on around 850 million tweets and so on.
Transformers and GPT Models
Overview of Transformers
- Transformers consist of two main components:
- Encoder
- Decoder
- BERT utilizes a stack of encoders, while GPT uses a stack of decoders.
- GPT models are neural network-based language prediction models built on Transformer architecture.
Decoder Structure
- The decoder has three parts:
- Masked Multi-Head Attention: Attends only to the left.
- Cross Attention: Attends to encoded inputs (removed in GPT).
- Feed Forward Neural Network.
Differences Between BERT and GPT
- Both BERT and GPT use masked multi-head attention, but unlike BERT that masks a word in the middle of the sentence, and tries to predict the mask, GPT masks all the future words and tries to predict them.
- Training methods differ:
- BERT masks tokens in sentences.
- GPT predicts the next token in a sequence.
Training Process
- In GPT, the model predicts the next token based on the previous tokens, treating the input as a sequence.
- The first GPT model (2018) had 117 million parameters and was trained on 7,000 books.
- It was seen that larger models tend to generalize better and have better "emergent abilities", although the reason is still unclear.
Evolution of GPT Models
- GPT-2 (2019) had 1.5 billion parameters.
- GPT-3 (2020) had 175 billion parameters.
- Larger models tend to generalize better. This trend suggests that increasing model size allows for deeper contextual understanding.
Chain of Thought Reasoning
- GPT-4 introduced a method called “chain of thought,” allowing the model to predict intermediate steps in reasoning tasks. It involves multi-step thinking: The model generates a series of logical steps to arrive at the final answer, rather than answering in a single sentence.
- Example: Problem: What is the result of 5 + 5 + 5? It is clear to see the answer is 15. With the chain of thought reasoning - Step 1: The first 5 plus the second 5 gives 10 (i.e. [math]\displaystyle{ 5+5=10 }[/math]). Step 2: add the last 5 to the result from Step 1 (i.e. [math]\displaystyle{ 10+5=15 }[/math]).
Alignment and Instruction Following
- ChatGPT is designed to follow instructions and align with user preferences, addressing issues like harmful or politically incorrect outputs.
- InstructGPT is a variant of GPT that focuses on following instructions using human feedback via Reinforcement Learning from Human Feedback (RLHF).
Text-Text Transfer Transformer (T5)
- T5 combines encoder and decoder structures and treats various NLP tasks as text-to-text problems, allowing for diverse applications like translation and summarization.
- NLP tasks such as sentiment analysis which were primarily treated as classification problems are cast as text-to-text problems.
- Next span prediction is used where a set of sequential tokens are removed and replaced with sentinel tokens.
- T5 architecture operates on an encoder-decoder model and encoder input padding can be performed on both left and right.
Training Techniques in T5
- T5 masks spans of tokens rather than individual tokens, focusing on global context rather than local dependencies. 15% of tokens are randomly removed and replaced with sentinel tokens.
Benchmarking and Performance
- Models are often evaluated against benchmarks like GLUE and SuperGLUE, which consist of various NLP tasks.
Training and Fine-Tuning LLMs
- LLMs are trained on massive datasets of unlabeled text using unsupervised learning.
- The training process involves predicting the next token in a sequence.
- Fine-tuning is done on labeled data to align the model with specific tasks or instructions. This process is called Supervised Fine-Tuning (SFT).
Aligning LLMs with Human Feedback
- Reinforcement Learning with Human Feedback (RLHF) is used to ensure LLMs produce safe and ethical outputs.
- RLHF involves generating multiple responses for a given prompt and having humans rank them.
- A reward model is trained on this ranked data to predict the quality of a response.
- The original LLM is then fine-tuned using this reward model.
- RLHF was introduced in 2017 and has been widely used in LLMs like ChatGPT.
Direct Preference Optimization (DPO)
- DPO is a newer approach that aims to replace RLHF.
- It directly collects user preferences for different responses, eliminating the need for a reward model.
- DPO is easier to implement and more stable than RLHF.
Project Ideas for Deep Learning
- Enhancing Speculative Decoding for Faster Large Language Modeling
- This project aims to improve the speed of LLMs by using techniques like rejection sampling and importance sampling.
- The goal is to find alternative sampling methods to rejection sampling, which is computationally expensive.
- Reducing the Computational Complexity of Transformers
- Transformers are computationally expensive, especially for long sequences.
- This project explores methods like ORCID, which uses data-dependent convolution to reduce complexity.
- The goal is to develop more efficient transformers that can handle longer sequences.
- Diffusion Decoding for Peptide De Novo Sequencing
- Peptide sequencing is a crucial problem in bioinformatics, involving determining the amino acid sequence of a peptide.
- This project proposes using diffusion models for peptide sequencing, replacing the traditional GPT-based approach.
- Diffusion models can potentially improve accuracy by predicting all tokens simultaneously, rather than sequentially.
- Using ORCID for DNA Analysis
- This project explores using ORCID, a model that can handle larger sequences, for DNA analysis.
- The goal is to leverage ORCID’s ability to handle long sequences to improve DNA analysis tasks.
- Symbolic Regression with Diffusion Models
- Symbolic regression involves finding a mathematical formula that best fits a given dataset.
- This project proposes using diffusion models for symbolic regression, potentially improving the accuracy and efficiency of the process.
Transformers and Variational Autoencoders
Large Language Models (LLMs) and Transformers
Key Components of Transformers
- Attention Mechanism Formula: The core of the attention mechanism is computed using the following formula:
- Dimensions:
- [math]\displaystyle{ X }[/math] as a [math]\displaystyle{ d \times n }[/math] matrix, [math]\displaystyle{ n }[/math] as sequence length, and [math]\displaystyle{ d }[/math] as data dimensionality.
- [math]\displaystyle{ Q }[/math], [math]\displaystyle{ K }[/math] and [math]\displaystyle{ V }[/math] are the query, key, and value matrices, respectively, and they are derived from [math]\displaystyle{ W_Q }[/math], [math]\displaystyle{ W_K }[/math] and [math]\displaystyle{ W_V }[/math].
- Given a sequence [math]\displaystyle{ X = \begin{bmatrix} x_1 & \cdots & x_n \end{bmatrix}_{d \times n} }[/math] we define:
- [math]\displaystyle{ Q = W_Q^T X \quad \text{(p x n)}, \quad W_Q^T \in \mathbb{R}^{p \times d}, \quad X \in \mathbb{R}^{d \times n} }[/math]
- [math]\displaystyle{ K = W_K^T X \quad \text{(p x n)}, \quad W_K^T \in \mathbb{R}^{p \times d} }[/math]
- [math]\displaystyle{ V = W_V^T X \quad \text{(m x n)}, \quad W_V^T \in \mathbb{R}^{m \times d} }[/math]
Recap of Transformers
- Building Blocks of LLMs: Transformers.
- Computational Complexity:
- Issue: Transformers have quadratic complexity concerning sequence length.
- Reason: Complexity arises due to calculations in the attention mechanism.
Approaches to Reduce Complexity
- Issue: Long sequences result in an [math]\displaystyle{ n \times n }[/math] matrix, making computation demanding.
- Solution: Techniques like the Performer model approximate attention, reducing complexity to linear time.
Performer Model
Overview
- Objective: Address quadratic complexity in transformers by approximating attention using kernel methods.
- Kernel Approximation: Utilizes random features to approximate kernels, e.g., Gaussian kernels. The kernel function [math]\displaystyle{ K(x, y) }[/math] is defined as:
- For the Gaussian kernel approximation, the transformation [math]\displaystyle{ \varphi_{\text{Gauss}}(x) }[/math] is given by:
- In general for kernel [math]\displaystyle{ K }[/math]:
Advantage
Scalability: Performers are highly scalable for very long sequences.
Memory Efficiency: Performers drastically reduce memory usage by linearizing attention.
Robust Performance: Despite the approximation, Performers retain high accuracy and performance, comparable to standard transformers.
Application
Performers are beneficial in applications requiring efficient processing of long sequences, such as natural language processing, DNA sequence analysis, and other domains where traditional transformers are computationally prohibitive.
Softmax and Attention Mechanism in Transformers
Softmax Formula
- In the context of transformers, the softmax function is used to normalize the attention scores:
- To calculate the softmax numerically, we can consider:
[math]\displaystyle{ e^{Q^T K} = A }[/math]
[math]\displaystyle{ d = \begin{bmatrix} & & & & \\ & & & & \\ & & & & \end{bmatrix}_{n \times n} \begin{bmatrix} 1 \\ 1 \\ \vdots \\ 1 \end{bmatrix}_{n \times 1} \\ }[/math]
[math]\displaystyle{ D = \operatorname{diag}(d) = \begin{bmatrix} d_1 & 0 & \cdots & 0 \\ 0 & d_2 & \cdots & 0 \\ \vdots & \vdots & \ddots & \vdots \\ 0 & 0 & \cdots & d_n \end{bmatrix}_{n \times n} }[/math]
[math]\displaystyle{ \operatorname{SM}[s]_i = \frac{e^{s_i}}{\sum_j e^{s_j}} = A D^{-1} }[/math]
- We want to be able to do the matrix multiplication in the following way, which has linear time complexity for long sequences in transformers:
Steps in Kernel Approximation
- Kernel Formulation: Approximate [math]\displaystyle{ \phi(x)^T \phi(y) }[/math] as a function of random vectors.
- Random Feature Transformation: Performers use sinusoidal functions (sine and cosine) as random features for approximating similarity between sequences.
- The softmax can also be approximated using random features, with a specific [math]\displaystyle{ \varphi(x) }[/math] tailored for softmax.
- So, the kernel approximation for the softmax is:
Variational Auto-encoders (VAEs)
- Overview: VAEs are a type of generative model that extends traditional auto-encoders by introducing stochastic elements. While traditional auto-encoders are deterministic and focus on reconstructing inputs, VAEs aim to learn a distribution over the latent space. This approach extends traditional autoencoders by introducing a probabilistic framework that enables them to capture complex data distributions.
Background: Auto-encoders
- Basic Structure: Consists of an encoder, a bottleneck layer, and a decoder.
- Objective: Maps input [math]\displaystyle{ x }[/math] into a lower-dimensional representation ([math]\displaystyle{ z = u^Tx }[/math]) by compressing it and reconstructs it. It minimizes the differences in [math]\displaystyle{ x }[/math] and the reconstructed version of it ([math]\displaystyle{ \hat{x} = uz }[/math]): [math]\displaystyle{ \min \lvert x - \hat{x} \rvert }[/math]
- PCA Connection: A simple, linear auto-encoder resembles PCA in dimensionality reduction.
- Limitation: While VAEs are powerful, they may struggle to capture highly complex data distributions compared to more recent generative models like GANs.
Moving to Variational Auto-encoders
- Generative Model Aspect: Variational Auto-encoders enforce a specific distribution on [math]\displaystyle{ z }[/math], allowing them to generate new samples.
- Gaussian Distribution Constraint: By enforcing a Gaussian distribution on [math]\displaystyle{ z }[/math], the model can generate new samples in the learned data distribution. So Variational Auto-encoder introduces a prior distribution (typically Gaussian) on [math]\displaystyle{ z }[/math] and maximizes the Evidence Lower Bound (ELBO) instead of reconstruction alone.
Approximation of the Posterior Distribution
- [math]\displaystyle{ \mathbf{q_{\theta}(z)} }[/math] is the approximate posterior distribution of the latent variable [math]\displaystyle{ z }[/math], parameterized by [math]\displaystyle{ \theta }[/math].
- Purpose of [math]\displaystyle{ \mathbf{q_{\theta}(z)} }[/math]: In a VAE, we aim to learn a latent variable [math]\displaystyle{ z }[/math] that represents the underlying structure of the data [math]\displaystyle{ x }[/math]. Ideally, we want the true posterior [math]\displaystyle{ p(z|x) }[/math], which is often intractable to compute directly. To address this, we approximate [math]\displaystyle{ p(z|x) }[/math] with a simpler distribution [math]\displaystyle{ q_{\theta}(z|x) }[/math], where [math]\displaystyle{ \theta }[/math] represents the parameters (typically learned through a neural network).
- Key Points about [math]\displaystyle{ \mathbf{q_{\theta}(z)} }[/math]:
- Approximate Posterior: [math]\displaystyle{ q_{\theta}(z|x) }[/math] is an approximation of the true posterior [math]\displaystyle{ p(z|x) }[/math].
- Parameterized by [math]\displaystyle{ \mathbf{\theta} }[/math]: The parameters [math]\displaystyle{ \theta }[/math] define the structure of this distribution, often through a neural network in a VAE.
- Optimization: During training, we optimize [math]\displaystyle{ \theta }[/math] to make [math]\displaystyle{ q_{\theta}(z|x) }[/math] as close as possible to [math]\displaystyle{ p(z|x) }[/math] by minimizing the KL divergence between [math]\displaystyle{ q_{\theta}(z|x) }[/math] and [math]\displaystyle{ p(z|x) }[/math]: [math]\displaystyle{ \min_{\theta} \text{KL} \left( q_{\theta}(z) || p(z|x) \right) }[/math]
Information Theory
In VAEs, the model learns a latent space where each data point is mapped not to a single point, but to a probability distribution. This design choice allows the VAE to: Capture the information content of the input data in a compressed, structured form. Represent the uncertainty in the data by encoding it as a distribution rather than as a single deterministic vector. The VAE learns this by balancing reconstruction accuracy with the information-theoretic regularization of the latent space, which enforces a smooth and organized representation.
- Information content:
- [math]\displaystyle{ I }[/math], represents the information content or self-information of an event with probability [math]\displaystyle{ p(x) }[/math]. It quantifies how much “surprise” or “information” is associated with a specific outcome [math]\displaystyle{ x }[/math] occurring.
- The formula for information content [math]\displaystyle{ I }[/math] of an event [math]\displaystyle{ x }[/math] is: [math]\displaystyle{ I = -\log p(x) }[/math]
- [math]\displaystyle{ I }[/math] is higher for less probable events (low [math]\displaystyle{ p(x) }[/math]), indicating more “surprise” or “information” content when that event occurs.
- In information theory, this concept helps quantify the amount of information gained from observing an event, with rare events providing more information than common ones.
- For example, given a sentence "Tomorrow, it either rains, or not." [math]\displaystyle{ p(x) = 1 }[/math] and so [math]\displaystyle{ I = -log(1) = 0 }[/math] since there is no insight to glean in that sentence.
- Entropy:
- [math]\displaystyle{ H }[/math] represents entropy. Entropy measures the average amount of information (or “uncertainty”) contained in a random variable. It is often used to quantify the uncertainty or unpredictability of a probability distribution.
- The formula for entropy [math]\displaystyle{ H }[/math] of a discrete random variable [math]\displaystyle{ x }[/math] with a probability distribution [math]\displaystyle{ p(x) }[/math] is: [math]\displaystyle{ H = -\sum p(x) \log p(x) }[/math]
- [math]\displaystyle{ H }[/math] is maximized when the distribution is most uncertain (e.g., in a uniform distribution).
- In the context of VAEs, entropy is used to understand the distribution of latent variables and how much “information” or “uncertainty” they contain.
- KL divergence:
- KL divergence, stands for the Kullback-Leibler divergence between two probability distributions [math]\displaystyle{ q }[/math] and [math]\displaystyle{ p }[/math]. It is a measure of how one probability distribution [math]\displaystyle{ q }[/math] diverges from a second, reference probability distribution [math]\displaystyle{ p }[/math].
- The KL divergence from [math]\displaystyle{ q(x) }[/math] to [math]\displaystyle{ p(x) }[/math] is defined as: [math]\displaystyle{ \text{KL}(q || p) = \sum q(x) \log q(x) - \sum q(x) \log p(x) = \sum_x q(x) \log \frac{q(x)}{p(x)} }[/math]
- KL divergence is not symmetric; [math]\displaystyle{ \text{KL}(q \| p) \neq \text{KL}(p \| q) }[/math]. This means that it matters which distribution we consider as the reference.
- KL divergence is always non-negative, [math]\displaystyle{ \text{KL}(q \| p) \geq 0 }[/math], and equals zero if and only if [math]\displaystyle{ q = p }[/math] (almost everywhere). This is known as Gibbs’ inequality.
- In variational inference and variational auto-encoders (VAEs), KL divergence is used to measure the difference between the approximate posterior [math]\displaystyle{ q_{\theta}(z|x) }[/math] and the true posterior [math]\displaystyle{ p(z|x) }[/math]. Minimizing [math]\displaystyle{ \text{KL}(q_{\theta}(z|x) | p(z|x)) }[/math] helps make the approximation [math]\displaystyle{ q_{\theta}(z|x) }[/math] closer to the true posterior.
- KL divergence quantifies the “information loss” if we approximate [math]\displaystyle{ p(x) }[/math] with [math]\displaystyle{ q(x) }[/math]. In essence, it tells us how much extra information (or “surprise”) is needed to describe samples from [math]\displaystyle{ q }[/math] as if they were from [math]\displaystyle{ p }[/math].
- For the continuous space:
KL Divergence and Evidence Lower Bound (ELBO)
- KL Divergence: Measures the similarity between two distributions; minimized to bring [math]\displaystyle{ q }[/math] close to [math]\displaystyle{ p }[/math].
- Evidence Lower Bound (ELBO):
- Variational auto-encoders maximize ELBO which is similar to minimizing KL divergence since [math]\displaystyle{ log(p(x)) }[/math] is a constant.
- Maximizing ELBO ensures [math]\displaystyle{ q }[/math] closely approximates [math]\displaystyle{ p }[/math], meaning maximizing ELBO ensures that the learned latent distribution is close to the target distribution. This approach allows VAEs to generate new samples by sampling from the latent space.
ELBO Decomposition
- ELBO Equation: [math]\displaystyle{ ELBO = q(z) log p(x|z) - KL[q(z) || p(z)] }[/math]
- First Term: Reconstruction likelihood (maximize this).
- Second Term: Ensures latent variable [math]\displaystyle{ z }[/math] approximates the desired distribution.
Practical Implementation: Reconstruction Loss and Gaussian Constraint
- Objective in VAE Training:
- Minimize reconstruction loss (similar to traditional auto-encoders).
- Ensure [math]\displaystyle{ z }[/math] follows a Gaussian distribution.
- Regularization Term in ELBO: In VAEs, the Evidence Lower Bound (ELBO) includes a KL divergence term to ensure that the learned latent distribution remains close to a prior distribution (e.g., a Gaussian [math]\displaystyle{ p(z) = N(0, I) }[/math]).
Re-parameterization Trick
- Challenge: The stochastic nature of [math]\displaystyle{ z }[/math] complicates backpropagation.
- Solution: To make the stochastic layer differentiable, VAEs use the re-parameterization trick, where [math]\displaystyle{ z }[/math] is expressed as a deterministic function with a noise component. So we use re-parameterization to enable gradient-based optimization and we minimize:
Reverse Processes
- We need to know the reverse diffusion process [math]\displaystyle{ p_{\theta}(x_{t-1}|x_t)=N(x_{t-1};\mu_{\theta}(x_t,t),\Sigma_{\theta}(x_t,t)) }[/math]
- Mean of q(x_{t-1}|x_t,x_0):
[math]\displaystyle{ \begin{align} q(x_{t-1}|x_t,x_0) &= q(x_{t}|x_{t-1},x_0) \frac{q(x_{t-1}|x_0)}{q(x_t|x_0)} \\ &\propto \exp\left( -\frac{1}{2} \left( \frac{(x_t - \sqrt{\alpha_t}x_{t-1})^2}{\beta_t} + \frac{(x_{t-1} - \sqrt{\bar{\alpha}_{t-1}}x_{0})^2}{1-\bar{\alpha}_{t-1}} - \frac{(x_t - \sqrt{\bar{\alpha}_{t}}x_{0})^2}{1-\bar{\alpha}_{t}} \right) \right)\\ &= \exp\left( -\frac{1}{2} \left( \left( \frac{\alpha_t}{\beta_t} + \frac{1}{1-\bar{\alpha_{t-1}}} \right) x_{t-1}^2 - \left( \frac{2\sqrt{\alpha_t}}{\beta_t} x_t + \frac{2\sqrt{\bar{\alpha_{t-1}}}}{1-\bar{\alpha_{t-1}}} x_0 \right) x_{t-1} + C(x_t,x_0) \right) \right) \end{align} }[/math]
, where [math]\displaystyle{ C(x_t,x_0) }[/math] is not a function of [math]\displaystyle{ x_{t-1} }[/math]
Define [math]\displaystyle{ \alpha_t=1-\beta_t, \bar{\alpha_t}=\prod_{i=1}^T{\alpha_i} }[/math],
[math]\displaystyle{ \tilde{\mu_t}(x_t,x_0)=\frac{\sqrt{\alpha_t}(1-\bar{\alpha_{t-1}})}{1-\bar{\alpha_t}}x_t + \frac{\sqrt{\bar{\alpha_{t-1}}}\beta_t}{1-\bar{\alpha_t}}x_0 }[/math]
Since [math]\displaystyle{ x_0=\frac{1}{\sqrt{\bar{\alpha_t}}}(x_t-\sqrt{1-\bar{\alpha_t}}\epsilon_t) }[/math], we have
[math]\displaystyle{ \tilde{\mu_t}(x_t,x_0)=\frac{1}{\sqrt{\bar{\alpha_t}}}\left(x_t-\frac{1-\alpha_t}{\sqrt{1-\bar{\alpha_t}}}\epsilon_t\right) }[/math]
- KL for two Gaussian
- Loss function
Graph Neural Network (GNN)
Graph Neural Networks are a class of deep learning methods designed to perform inference on data described by graphs.
Application
- Graphs are Structured Data: Many real-world datasets can be represented as graphs. Examples: social networks, protein-interaction networks, the World Wide Web, and molecules, where entities and their relationships are mapped as nodes and edges. In social networks, each user can be represented as a node and the relationships or interactions between them — such as friendships and follows — are represented as edges connecting the nodes. GNN can be used to recommend friends and content by learning from the network structure. Also, GNN can help to track the spread of information or behaviors across the network.
- Images as Graphs: Images can be considered as graphs where each pixel acts as a node. In this representation, each pixel node connects to its adjacent pixels through edges and form a grid-like graph structure that captures the spatial relationships in the image.
- Text as Graphs: Text data can also be represented as graphs. Here, each token or word is a node, and each node is connected by edges to the preceding and following tokens, which can capture the sequential dependencies in the text.
- CNN as GNN: Convolutional Neural Networks (CNNs) can be viewed as a specialized form of Graph Neural Networks (GNNs) applied to grid-like structures. Each pixel in an image represents a node connected to its neighbors, with convolutions aggregating features across the whole grid.
Tasks
There are 3 different levels of tasks.
- Graph-Level Tasks: These involve predicting a property of the entire graph. For example, in molecular graphs, we may predict whether a particular molecule will bind to a receptor based on the graph structure.
- Node-Level Tasks: These focus on predicting the identity or role of each individual node within the graph. This could involve classifying nodes based on their attributes or their position within the network.
- Edge-Level Tasks: These involve analyzing or predicting relationships between pairs of nodes, such as determining whether two nodes in a social network graph are friends or not.
Graph
Definition
A graph, denoted as [math]\displaystyle{ G }[/math], can be defined by a set of vertices (or nodes) [math]\displaystyle{ V }[/math] and edges (connections between the vertices) [math]\displaystyle{ V }[/math], along with an adjacency matrix [math]\displaystyle{ A }[/math]. This matrix represents the relationships between vertices in a binary format.
In graph notation, we write this as [math]\displaystyle{ G = (V, E, A) }[/math], where:
- [math]\displaystyle{ V }[/math]: Vertices or nodes in the graph.
- [math]\displaystyle{ E }[/math]: Edges, which indicate connections between pairs of vertices.
- [math]\displaystyle{ A }[/math]: Adjacency matrix, a binary matrix that indicates the presence (1) or absence (0) of an edge between vertex pairs.
The adjacency matrix is a binary matrix indicating whether pairs of vertices are adjacent and is structured so that:
The entry [math]\displaystyle{ A_{ij} = 1 }[/math] if there is an edge between vertex [math]\displaystyle{ i }[/math] and vertex [math]\displaystyle{ j }[/math]. The entry [math]\displaystyle{ A_{ij} = 0 }[/math] if there is no edge between vertex [math]\displaystyle{ i }[/math] and vertex [math]\displaystyle{ j }[/math].
In this way, the adjacency matrix provides a clear view of the connections within the graph.
Laplacian of a Graph
Laplacian Matrix Definition
[math]\displaystyle{ L = D - A }[/math]
[math]\displaystyle{ D }[/math]: Degree matrix, which is a diagonal matrix where each diagonal entry represents the degree of each vertex.
[math]\displaystyle{ A }[/math]: Adjacency matrix, as previously defined.
Degree Matrix Notation
The degree matrix [math]\displaystyle{ D }[/math] is a square matrix in which each diagonal element [math]\displaystyle{ d_i }[/math] represents the degree of the corresponding vertex [math]\displaystyle{ i }[/math]. [math]\displaystyle{ D = \begin{bmatrix} d_1 & 0 & \dots & 0 \\ 0 & d_2 & \dots & 0 \\ \vdots & \vdots & \ddots & \vdots \\ 0 & 0 & \dots & d_n \end{bmatrix}_{n \times n} }[/math]
Degree of a Vertex is defined as [math]\displaystyle{ d_i = \sum_j A_{ij} }[/math]. The degree [math]\displaystyle{ d_i }[/math] is the sum of the elements in the [math]\displaystyle{ i }[/math]-th row of [math]\displaystyle{ A }[/math], representing the number of edges connected to vertex [math]\displaystyle{ i }[/math].
Example
For an adjacency matrix [math]\displaystyle{ A }[/math] as follows:
[math]\displaystyle{ A = \begin{bmatrix} 0 & 1 & 1 & 0 \\ 1 & 0 & 1 & 0 \\ 1 & 1 & 0 & 1 \\ 0 & 0 & 1 & 0 \end{bmatrix} }[/math]
The sum of each row (representing each vertex's degree) results in:
[math]\displaystyle{ \text{Sum} = \begin{bmatrix} \sum A_{1j} \\ \sum A_{2j} \\ \sum A_{3j} \\ \sum A_{4j} \end{bmatrix} = \begin{bmatrix} 2 \\ 2 \\ 3 \\ 1 \end{bmatrix} }[/math]
This yields the degree matrix [math]\displaystyle{ D }[/math] as:
[math]\displaystyle{ D = \begin{bmatrix} 2 & 0 & 0 & 0 \\ 0 & 2 & 0 & 0 \\ 0 & 0 & 3 & 0 \\ 0 & 0 & 0 & 1 \end{bmatrix} }[/math]
The Laplacian matrix [math]\displaystyle{ L }[/math] is then calculated as:
[math]\displaystyle{ L = D - A }[/math]
Graph Cut Optimization Problem
The graph cut optimization problem aims to partition a graph into segments with minimal interconnections between them.
- Objective: The goal is to find a cut that divides the graph into distinct segments while minimizing the connections between them.
- Minimization Target: The target function to minimize is [math]\displaystyle{ \sum A_{ij} (y_i - y_j)^2 }[/math], which captures the cost associated with the "cut" between segments.
- Equation: The minimization can be expressed as [math]\displaystyle{ y^T L y }[/math], where [math]\displaystyle{ L }[/math] is the Laplacian matrix, and [math]\displaystyle{ y }[/math] is a vector representing the segment assignment of each node.
- Constraint Applied: The constraint [math]\displaystyle{ y^T y = 1 }[/math] is applied to ensure non-trivial solutions.
- Vector [math]\displaystyle{ y }[/math]: This vector represents the assignment of nodes to segments, with dimensions [math]\displaystyle{ n \times 1 }[/math], where [math]\displaystyle{ n }[/math] is the number of nodes.
- Solution Method: Optimal partitioning is achieved by performing eigen decomposition on the Laplacian matrix [math]\displaystyle{ L }[/math].
Eigen Decomposition of Laplacian
The Laplacian matrix [math]\displaystyle{ L }[/math] can be decomposed as [math]\displaystyle{ L = U \Lambda U^T }[/math], where:
[math]\displaystyle{ U }[/math] represents the orthonormal eigenvectors. These eigenvectors are both orthogonal to each other and normalized. [math]\displaystyle{ \Lambda }[/math] is a diagonal matrix containing the eigenvalues. Each diagonal entry in [math]\displaystyle{ \Lambda }[/math] corresponds to an eigenvalue that pairs with an eigenvector in [math]\displaystyle{ U }[/math].
Starting with the standard Laplacian, defined as [math]\displaystyle{ L = D - A }[/math]:
[math]\displaystyle{ D }[/math] is the degree matrix. [math]\displaystyle{ A }[/math] is the adjacency matrix. The normalized Laplacian is given by: [math]\displaystyle{ \tilde{L} = D^{-\frac{1}{2}} (D - A) D^{-\frac{1}{2}} }[/math]
This expression can be simplified to: [math]\displaystyle{ \tilde{L} = I - D^{-\frac{1}{2}} A D^{-\frac{1}{2}} }[/math], where [math]\displaystyle{ I }[/math] is the identity matrix.
Laplacian Eigenvectors and Fourier Analysis Analogy
- Mathematical Definition: Decomposition of a signal into sinusoidal components.
- Frequency Domain: A signal is transformed to represent it as a sum of its frequency components.
- Basis Functions: Sine and cosine functions serve as the basis for this transformation.
- Orthogonality: These basis functions are orthogonal, ensuring unique frequency representation.
- Graph Signals: Functions defined over the nodes of a graph.
- Spectral Domain: Eigenvectors of [math]\displaystyle{ L }[/math] transform graph signals into the spectral domain.
- Eigenvector Basis: Analogous to sines and cosines, eigenvectors form a basis for graph signals.
- Orthogonality: Eigenvectors are orthogonal, providing a unique spectral representation for graph signals.
- Low Eigenvalues: Correspond to "low-frequency" eigenvectors. These eigenvectors change slowly over the graph. Represent large-scale, smooth structures in the graph.
- High Eigenvalues: Correspond to "high-frequency" eigenvectors. These eigenvectors change rapidly between connected nodes. Capture fine details or irregularities in the graph.
- Ordering: Eigenvalues (and eigenvectors) are ordered from lowest to highest.
Fourier Transform
The eigen-decomposition of the graph Laplacian [math]\displaystyle{ L }[/math] can be written as: [math]\displaystyle{ L = U^T \Lambda U }[/math], where:
- [math]\displaystyle{ U = [u_1, ..., u_n] }[/math] is the matrix of orthonormal eigenvectors.
- [math]\displaystyle{ \Lambda }[/math] is a diagonal matrix where each entry [math]\displaystyle{ \Lambda_{ii} = \lambda_i }[/math] represents an eigenvalue (also known as the spectrum of the Laplacian).
- The equation [math]\displaystyle{ U^T U = I }[/math] indicates that the eigenvectors are orthonormal.
The eigenvectors from [math]\displaystyle{ u_1 }[/math] to [math]\displaystyle{ u_n }[/math] are also called Fourier functions, as they serve as a basis for transforming signals on the graph.
The Fourier transform projects a signal [math]\displaystyle{ x }[/math] onto the Fourier functions, resulting in coefficients that form the Fourier series.
In the context of graphs, the graph Fourier transform projects an input graph signal onto an orthonormal basis formed by the eigenvectors of the normalized graph Laplacian.
Suppose [math]\displaystyle{ x \in \mathbb{R}^n }[/math] is a feature vector containing the values of all nodes in a graph, where [math]\displaystyle{ x_i }[/math] represents the value at the [math]\displaystyle{ i }[/math]-th node.
- The graph Fourier transform of a signal [math]\displaystyle{ x }[/math] is given by: [math]\displaystyle{ f(x) = U^T x = \hat{x} }[/math]
- The inverse graph Fourier transform is defined as: [math]\displaystyle{ f^{-1}(\hat{x}) = U \hat{x} = U U^T x = x }[/math]
ConvGNNs
The graph convolution of an input signal [math]\displaystyle{ x }[/math] with a filter [math]\displaystyle{ g \in \mathbb{R}^n }[/math] is defined as follows:
Convolution Theorem: [math]\displaystyle{ f(x * g) = (f(x) \cdot f(g)) }[/math]
This can be expanded as:
- [math]\displaystyle{ x * g = f^{-1}(f(x) \cdot f(g)) }[/math]
- [math]\displaystyle{ = U (U^T x \cdot U^T g) }[/math]
- [math]\displaystyle{ = U g_\theta U^T x }[/math]
Where [math]\displaystyle{ g_\theta = \text{diag}(U^T g) }[/math].
Neural Network Layers
- Feedforward Neural Networks (FFNN):
Computation proceeds in layers, with each layer represented as [math]\displaystyle{ h' = \sigma(Wh) }[/math]. The output of each layer [math]\displaystyle{ h' }[/math] serves as the input for the next layer. The initial input [math]\displaystyle{ h_0 }[/math] is the feature vector [math]\displaystyle{ x }[/math].
- Convolutional Neural Networks (CNN):
Layer computation involves convolution, with each layer defined as [math]\displaystyle{ h' = \sigma(w * h) }[/math]. [math]\displaystyle{ w }[/math] represents the learned filters or kernels that slide over [math]\displaystyle{ h }[/math].
Vanilla Spectral GNN
The graph convolutional layer of the Spectral CNN is defined as:
[math]\displaystyle{ x * g = f^{-1}(f(x) \cdot f(g)) }[/math] [math]\displaystyle{ = U (U^T x \cdot U^T g) }[/math] [math]\displaystyle{ = U g_\theta U^T x }[/math]
With:
- [math]\displaystyle{ H' = \sigma(U \Theta U^T H) }[/math]
- [math]\displaystyle{ X = H^{(0)} }[/math]
- [math]\displaystyle{ g_\theta = \Theta }[/math]
Here, [math]\displaystyle{ \Theta }[/math] is a diagonal matrix with learnable parameters, where [math]\displaystyle{ U^T g = \begin{bmatrix} u_1^T g \ \vdots \ u_n^T g \end{bmatrix} }[/math] and [math]\displaystyle{ \Theta = \begin{bmatrix} u_1^T g & \dots & u_n^T g \end{bmatrix} }[/math].
Limitation: Eigen-decomposition requires [math]\displaystyle{ O(n^2) }[/math] computational complexity.
ChebNet
Approximates the filter [math]\displaystyle{ g_\theta }[/math] by Chebyshev [*] polynomials of the diagonal matrix of eigenvalues [math]\displaystyle{ \Lambda }[/math].
Chebyshev Polynomials of the first kind
- [math]\displaystyle{ T_0(x) = 1 }[/math]
- [math]\displaystyle{ T_1(x) = x }[/math]
- For [math]\displaystyle{ i \geq 2 }[/math], the polynomials are defined as: [math]\displaystyle{ T_i(x) = 2x T_{i-1}(x) - T_{i-2}(x) }[/math]
ChebNet Graph Convolution
- [math]\displaystyle{ g_{\theta}=\sum_{i}{\theta_i{T_i}(\tilde{\Alpha}}) }[/math].
- [math]\displaystyle{ g_{\theta} }[/math] is a Graph convolutional filter with parameter [math]\displaystyle{ \theta }[/math].
- [math]\displaystyle{ \tilde{\Alpha} }[/math] is are scaled eigenvalues of the Laplacian matrix: [math]\displaystyle{ \tilde{\Alpha}=\frac{2\Alpha}{\lambda_{max}}-I_n }[/math], which makes the normalized eigenvalues fall into [-1,1].
- [math]\displaystyle{ x*{g}=Ug_{\theta}U^Tx }[/math]
- [math]\displaystyle{ x*{g}=\sum_{i}\theta_iUT_i(\tilde{\Alpha})U^Tx }[/math]
- [math]\displaystyle{ x*{g_{\theta}}=U\left(\sum_{i}\theta_iT_i(\tilde{\Alpha})\right)U^Tx }[/math]
- This is equal to: [math]\displaystyle{ x*{g_{\theta}}=\sum_{i=1}^k{\theta_iT_i(\tilde{L})x} }[/math], where [math]\displaystyle{ \tilde{L}=\frac{2L}{\lambda_{max}}-I_n }[/math]