Research Papers Classification System: Difference between revisions
(61 intermediate revisions by 36 users not shown) | |||
Line 3: | Line 3: | ||
= Introduction = | = Introduction = | ||
This paper introduces a paper classification system that utilizes the Term | With the increasing advance of computer science and information technology, an increasingly overwhelming number of papers have been published. Due to the great volume, it has become incredibly hard to find and categorize papers. Typically this is a very time consuming activity. This paper introduces a paper classification system that utilizes the Term Frequency-Inverse Document Frequency (TF-IDF), Latent Dirichlet Allocation (LDA), and K-means clustering. The most important technology the system used to process big data is the Hadoop Distributed File System (HDFS). The system is highly fault-tolerant and can be deployed on low-cost hardware. It can handle quantitatively complex research paper classification problems efficiently and accurately. | ||
===General Framework=== | ===General Framework=== | ||
Line 9: | Line 9: | ||
The paper classification system classifies research papers based on the abstracts given that the core of most papers is presented in the abstracts. | The paper classification system classifies research papers based on the abstracts given that the core of most papers is presented in the abstracts. | ||
[[ File:Systemflow.png |right|image on right| 400px]] | |||
<ol><li>Paper Crawling | <ol><li>Paper Crawling | ||
<p>Collects abstracts from research papers published during a given period</p></li> | <p>Collects abstracts and keywords from research papers published during a given period</p></li> | ||
<li>Preprocessing | <li>Preprocessing | ||
<p> <ol style="list-style-type:lower-alpha"><li>Removes stop words in the papers crawled, in which only nouns are extracted from the papers</li> | <p> <ol style="list-style-type:lower-alpha"><li>Removes stop words in the papers crawled, in which only nouns are extracted from the papers</li> | ||
Line 27: | Line 28: | ||
<p> Calculates the Document Frequency (DF) values which represents the frequency of keywords in a collection of research papers. The higher the DF value, the lower the importance of a keyword.</p> | <p> Calculates the Document Frequency (DF) values which represents the frequency of keywords in a collection of research papers. The higher the DF value, the lower the importance of a keyword.</p> | ||
</li> | </li> | ||
<li>TF-IDF calculation | <li>Term frequency-inverse document frequency (TF-IDF) calculation | ||
<p> Calculates the inverse of the DF which represents the importance of a keyword.</p> | <p> Calculates the inverse of the DF which represents the importance of a keyword.</p> | ||
</li> | </li> | ||
<li>Paper Classification | <li>Paper Classification | ||
<p> Classify papers by topics using the K-means clustering algorithm.</p> | <p> Classify papers by topics and similar subjects using the K-means clustering algorithm.</p> | ||
</li> | </li> | ||
</ol> | </ol> | ||
Line 37: | Line 38: | ||
===Technologies=== | ===Technologies=== | ||
The HDFS with a Hadoop cluster composed of one master node, one sub node, and four data nodes is what is used to process the massive paper data. Hadoop-2.6.5 version in Java is what is used to perform the TF-IDF calculation. Spark MLlib is what is used to perform the LDA. The Scikit-learn library is what is used to perform the K-means clustering. | The HDFS with a Hadoop cluster composed of one master node, one sub-node, and four data nodes is what is used to process the massive paper data. Hadoop-2.6.5 version in Java is what is used to perform the TF-IDF calculation. Spark MLlib is what is used to perform the LDA. The Scikit-learn library is what is used to perform the K-means clustering. | ||
===HDFS=== | ===HDFS=== | ||
Hadoop Distributed File | Hadoop Distributed File System (HDFS) was used to process big data in this system. HFDS has been shown to process big data rapidly and stably with high scalability which makes it a perfect choice for this problem. What Hadoop does is to break a big collection of data into different partitions and pass each partition to one individual processor. Each processor will only have information about the partition of data it has received. | ||
'''In this summary, we are going to focus on introducing the main algorithms of what this system uses, namely LDA, TF-IDF, and K-Means.''' | '''In this summary, we are going to focus on introducing the main algorithms of what this system uses, namely LDA, TF-IDF, and K-Means.''' | ||
Line 48: | Line 49: | ||
===Crawling of Abstract Data=== | ===Crawling of Abstract Data=== | ||
Under the assumption that audiences tend to first read the abstract of | Under the assumption that audiences tend to first read the abstract of a paper to gain an overall understanding of the material, it is reasonable to assume the abstract section includes “core words” that can be used to effectively classify a paper's subject. | ||
An abstract is crawled to have its stop words removed. Stop words are words that are usually ignored by search engines, such as “the”, “a”, and etc. | An abstract is crawled to have its stop words removed. Stop words are words that are usually ignored by search engines, such as “the”, “a”, and etc. Afterward, nouns are extracted, as a more condensed representation for efficient analysis. | ||
This is managed on HDFS. The TF-IDF value of each paper is calculated through map-reduce, an easy-to-use programming model and implementation for processing and generating large data sets. The user must specify (i) a map procedure, that filters and sorts the input data to produce a set of intermediate key/value pairs and (ii) a reduce function, which performs a summary operation on the intermediate values with the same key and returns a smaller set of output key/value pairs. The MapReduce interface enables this process by grouping the intermediate values with the same key and passing them as input to the reduce function. For example, one could count the number of times various words appear in a large number of documents by setting your map procedure to count the number of occurrences of each word in a single document, and your reduce function to sum all counts of a given word [[https://dl.acm.org/doi/pdf/10.1145/1327452.1327492?casa_token=_Zg_DWxQzKEAAAAA:EHII0CaP36_ojGMT8huqTGLNMSEc-CKzZAoXBxSXe6pr2WB0DCQvEKa30CFQW0NSbB2-CVo8GcBcJAg 1]]. | |||
===Managing Paper Data=== | ===Managing Paper Data=== | ||
To construct an effective keyword dictionary using abstract data and keywords data in all of the crawled papers, the authors categorized keywords with similar meanings using a single representative keyword. The approach is called stemming, which is common in cleaning data. 1394 keyword categories are extracted, which is still too much to compute. Hence, only the top 30 keyword categories are used. | To construct an effective keyword dictionary using abstract data and keywords data in all of the crawled papers, the authors categorized keywords with similar meanings using a single representative keyword. The approach is called stemming, which is common in cleaning data, where words are reduced to their word stem. An example is "running" and "ran" would be reduced to "run". 1394 keyword categories are extracted, which is still too much to compute. Hence, only the top 30 keyword categories are used. | ||
<div align="center">[[File:table_1_kswf.JPG|700px]]</div> | <div align="center">[[File:table_1_kswf.JPG|700px]]</div> | ||
Line 62: | Line 65: | ||
Latent Dirichlet allocation (LDA) is a generative probabilistic model that views documents as random mixtures over latent topics. Each topic is a distribution over words, and the goal is to extract these topics from documents. | Latent Dirichlet allocation (LDA) is a generative probabilistic model that views documents as random mixtures over latent topics. Each topic is a distribution over words, and the goal is to extract these topics from documents. | ||
LDA estimates the topic-word distribution <math>P\left(t | z\right)</math> and the document-topic distribution <math>P\left(z | d\right)</math> using Dirichlet priors for the distributions with a fixed number of topics. For each document, obtain a feature vector: | LDA estimates the topic-word distribution <math>P\left(t | z\right)</math> (probability of word "z" having topic "t") and the document-topic distribution <math>P\left(z | d\right)</math> (probability of finding word "z" within a given document "d") using Dirichlet priors for the distributions with a fixed number of topics. For each document, obtain a feature vector: | ||
\[F = \left( P\left(z_1 | d\right), P\left(z_2 | d\right), \cdots, P\left(z_k | d\right) \right)\] | \[F = \left( P\left(z_1 | d\right), P\left(z_2 | d\right), \cdots, P\left(z_k | d\right) \right)\] | ||
Line 73: | Line 76: | ||
===LDA Intuition=== | ===LDA Intuition=== | ||
LDA uses the Dirichlet priors of the Dirichlet distribution. The following picture illustrates 2-simplex Dirichlet distributions with different alpha values, one for each corner of the triangles. | LDA uses the Dirichlet priors of the Dirichlet distribution, which allows the algorithm to model a probability distribution ''over prior probability distributions of words and topics''. The following picture illustrates 2-simplex Dirichlet distributions with different alpha values, one for each corner of the triangles. | ||
<div align="center">[[File:dirichlet_dist.png|700px]]</div> | <div align="center">[[File:dirichlet_dist.png|700px]]</div> | ||
Simplex is a generalization of the notion of a triangle. In Dirichlet distribution, each parameter will be represented by a corner in simplex, so adding additional parameters implies increasing the dimensions of simplex. As illustrated, when alphas are smaller than 1 the distribution is dense at the corners. When the alphas are greater than 1 the distribution is dense at the centers. | Simplex is a generalization of the notion of a triangle in k-1 dimension where k is the number of classes. For example, if you wish to classify essays into three groups, English, History and Math then the simplex would be a 2 dimension triangle, if you add philosophy as one of your potential class, then we would need a tetrahedron in 3 deminsion. In Dirichlet distribution, each parameter will be represented by a corner in simplex, so adding additional parameters implies increasing the dimensions of simplex. As illustrated, when alphas are smaller than 1 the distribution is dense at the corners. When the alphas are greater than 1 the distribution is dense at the centers. | ||
The following illustration shows an example LDA with 3 topics, 4 words and 7 documents. | The following illustration shows an example LDA with 3 topics, 4 words and 7 documents. | ||
Line 88: | Line 91: | ||
TF-IDF is widely used to evaluate the importance of a set of words in the fields of information retrieval and text mining. It is a combination of term frequency (TF) and inverse document frequency (IDF). The idea behind this combination is | TF-IDF is widely used to evaluate the importance of a set of words in the fields of information retrieval and text mining. It is a combination of term frequency (TF) and inverse document frequency (IDF). The idea behind this combination is | ||
It evaluates the importance of a word within a document | * It evaluates the importance of a word within a document | ||
It evaluates the importance of the word among the collection of all documents | * It evaluates the importance of the word among the collection of all documents | ||
The inverse of the document frequency accounts for the fact that term frequency will naturally increase as document frequency increases. Thus IDF is needed to counteract a word's TF to give an accurate representation of a word's importance. | |||
The TF-IDF formula has the following form: | The TF-IDF formula has the following form: | ||
Line 107: | Line 112: | ||
\[TF_{i,j} = \frac{n_{i,j} }{\sum_k n_{k,j} }\] | \[TF_{i,j} = \frac{n_{i,j} }{\sum_k n_{k,j} }\] | ||
where i stands for the <math>i^{th}</math> word, j stands for the <math>j^{th}</math> document, | where i stands for the <math>i^{th}</math> word, j stands for the <math>j^{th}</math> document, <math>n_{i,j}</math> stands for the number of times words <math>t_i</math> appear in document <math>d_j</math> and <math>\sum_k n_{k,j} </math> stands for total number of occurence of words in document <math>d_j</math>. | ||
Note that the denominator is the total number of words remaining in document j after crawling. | Note that the denominator is the total number of words remaining in document j after crawling. | ||
===Document Frequency (DF)=== | ===Document Frequency (DF)=== | ||
DF evaluates the percentage of documents that contain a given word over the entire collection of documents. Thus, the higher DF value is, the less important the word is | DF evaluates the percentage of documents that contain a given word over the entire collection of documents. Thus, the higher DF value is, the less important the word is. | ||
<math>DF_{i}</math> is the number of documents in the collection with word i divided by the total number of documents in the collection. The formula for DF has the following form: | <math>DF_{i}</math> is the number of documents in the collection with word i divided by the total number of documents in the collection. The formula for DF has the following form: | ||
Line 122: | Line 126: | ||
where <math>n_{i,k}</math> is the number of times word i appears in document k, |D| is the total number of documents in the collection. | where <math>n_{i,k}</math> is the number of times word i appears in document k, |D| is the total number of documents in the collection. | ||
Since DF and the importance of the word have an inverse relation, we use inverse document frequency (IDF) instead of DF. | |||
===Inverse Document Frequency (IDF)=== | ===Inverse Document Frequency (IDF)=== | ||
Line 134: | Line 139: | ||
\[IDF_{i} = log\left(\frac{|D|+1}{|\{d_k \in D: n_{i,k} > 0\}|+1}\right)\] | \[IDF_{i} = log\left(\frac{|D|+1}{|\{d_k \in D: n_{i,k} > 0\}|+1}\right)\] | ||
The inverse document frequency gives a measure of how rare a certain term is in a given document corpus. | |||
=Paper Classification Using K-means Clustering= | =Paper Classification Using K-means Clustering= | ||
K-means clustering is an unsupervised classification algorithm that groups similar data into the same class. It is an efficient and simple method that can be applied to different types of data attributes. It is also flexible enough to handle various kinds of noise and outliers. | |||
<br> | <br> | ||
Line 143: | Line 150: | ||
<br> | <br> | ||
Moreover, when assigning data into a cluster, the algorithm will also try to minimise the distances between the data and the centre of the cluster which the data belongs to. That is, k-means clustering will | Moreover, when assigning data into a cluster, the algorithm will also try to minimise the distances between the data and the centre of the cluster which the data belongs to. That is, k-means clustering will minimize the sum of square error: | ||
\begin{align*} | \begin{align*} | ||
Line 158: | Line 165: | ||
</ul> | </ul> | ||
<br> | <br> | ||
K-means Clustering algorithm, an unsupervised algorithm, is chosen because of its advantages to deal with different types of attributes, to run with minimal requirement of domain knowledge, to deal with noise and outliers, to realize clusters with similarities. | |||
Since the goal for this paper is to classify research papers and group papers with similar topics based on keywords, the paper uses the K-means clustering algorithm. The algorithm first computes the cluster centroid for each group of papers with a specific topic. Then, it will assign a paper into a cluster based on the Euclidean distance between the cluster centroid and the paper’s TF-IDF value. | Since the goal for this paper is to classify research papers and group papers with similar topics based on keywords, the paper uses the K-means clustering algorithm. The algorithm first computes the cluster centroid for each group of papers with a specific topic. Then, it will assign a paper into a cluster based on the Euclidean distance between the cluster centroid and the paper’s TF-IDF value. | ||
<br> | <br> | ||
However, different values of <math>k</math> (the number of clusters) will return different clustering results. Therefore, it is important to define the number of clusters before clustering. For example, in this paper, the authors choose to use the Elbow scheme to determine the value of <math>k</math>. Also, to measure the performance of clustering, the authors decide to use the Silhouette scheme. The results of clustering are validated if the Silhouette scheme returns a value greater than <math>0.5</math>. | However, different values of <math>k</math> (the number of clusters) will return different clustering results. Therefore, it is important to define the number of clusters before clustering. For example, in this paper, the authors choose to use the Elbow scheme to determine the value of <math>k</math>. The Elbow scheme is a somewhat subjective way of choosing an optimal <math>k</math> that involves plotting the average of the squared distances from the cluster centers of the respective clusters (distortion) as a function of <math>k</math> and choosing a <math>k</math> at which point the decrease in distortion is outweighed by the increase in complexity. Also, to measure the performance of clustering, the authors decide to use the Silhouette scheme. The Silhouette scheme is a measure of how well the objects lie within each cluster. Silhouette scores lie from -1 to 1. A positive score indicates that the object is well-matched with its own cluster, while a negative score indicates the opposite (Kaufman & Rousseeuw, 2005). The results of clustering are validated if the Silhouette scheme returns a value greater than <math>0.5</math>. | ||
=System Testing Results= | =System Testing Results= | ||
Line 169: | Line 179: | ||
<div align="center">[[File:table_3_tmtckd.JPG|700px]]</div> | <div align="center">[[File:table_3_tmtckd.JPG|700px]]</div> | ||
Then, the authors use the Elbow scheme to define the number of clusters for each method with different numbers of keywords before running the K-means clustering algorithm. The results are shown below: | Then, the authors use the Elbow scheme to define the number of clusters for each method with different numbers of keywords before running the K-means clustering algorithm. The results are shown below: | ||
<div align="center">[[File:table_4_nocobes.JPG|700px]]</div> | <div align="center">[[File:table_4_nocobes.JPG|700px]]</div> | ||
According to | According to Table 4, there is a positive correlation between the number of keywords and the number of clusters. In addition, method 3 combines the advantages for both method 1 and method 2; thus, method 3 requires the least clusters in total. On the other hand, the wrong keywords might be presented in papers; hence, it might not be possible to group papers with similar subjects correctly by using method 1 and so method 1 needs the most number of clusters in total. | ||
Next, the Silhouette scheme had been used for measuring the performance for clustering. The average of the Silhouette values for each method with different numbers of keywords are shown below: | Next, the Silhouette scheme had been used for measuring the performance for clustering. The average of the Silhouette values for each method with different numbers of keywords are shown below: | ||
Line 183: | Line 193: | ||
Since the clustering is validated if the Silhouette’s value is greater than 0.5, for methods with 10 and 30 keywords, the K-means clustering algorithm produces good results. | Since the clustering is validated if the Silhouette’s value is greater than 0.5, for methods with 10 and 30 keywords, the K-means clustering algorithm produces good results. | ||
To evaluate the accuracy of the classification system in this paper, the authors use the F-Score. The authors execute 5 times of experiment and use 500 randomly selected research papers for each trial. The following histogram shows the average value of F-Score for the three methods and different numbers of keywords: | To evaluate the accuracy of the classification system in this paper, the authors use the F-Score. The authors execute 5 times of experiment and use 500 randomly selected research papers for each trial. The following histogram shows the average value of F-Score for the three methods and different numbers of keywords: | ||
<div align="center">[[File:fig_16_fsvotm.JPG|700px]]</div> | <div align="center">[[File:fig_16_fsvotm.JPG|700px]]</div> | ||
Note that “TFIDF” means method 1, “LDA” means method 2, and “TFIDF-LDA” means method 3. The number 10, 20, and 30 after each method is the number of keywords the method has used. | Note that “TFIDF” means method 1, “LDA” means method 2, and “TFIDF-LDA” means method 3. The number 10, 20, and 30 after each method is the number of keywords the method has used. | ||
Line 194: | Line 204: | ||
=Conclusion= | =Conclusion= | ||
This paper introduces a classification system that classifies papers into different topics by using TF-IDF and LDA scheme with K-means clustering algorithm. This system allows users to search the papers they want quickly and with the most productivity. | This paper introduces a classification system that classifies research papers into different topics by using TF-IDF and LDA scheme with K-means clustering algorithm. The experimental results showed that the proposed system could classify the papers with similar subjects according to the keywords extracted from the abstracts of papers. In particular, when a keyword dictionary with both of the keywords extracted from the abstracts and the topics extracted by the LDA scheme is used, the classification system has a better clustering performance and higher F-Score values. The authors emphasized that the system can be implemented efficiently on high-performance computing infrastructure, using industry-standard technologies. This system allows users to search for the papers they want quickly and with the most productivity. | ||
Furthermore, this classification system might be | Furthermore, this classification system might also be used in different types of texts (e.g., documents, tweets, etc.) instead of only classifying research papers. | ||
=Critique= | =Critique= | ||
In this paper, DF values are calculated within each partition. This results that for each partition, DF value for a given word will vary and may have an inconsistent result for different partition methods. As mentioned above, there might be a divide by zero problem since some partitions do not have documents containing a given word, but this can be solved by introducing a dummy document as the authors did. Another method that might be better at solving inconsistent results and the divide by zero problems is to have all partitions to communicate with their DF value. Then pass the merged DF value to all partitions to do the final IDF and TF-IDF value. Having all partitions to communicate with the DF value will guarantee a consistent DF value across all partitions and helps avoid a divide by zero problem as words in the keyword dictionary must appear in some documents in the whole collection. | In this paper, DF values are calculated within each partition. This results that for each partition, DF value for a given word will vary and may have an inconsistent result for different partition methods. As mentioned above, there might be a divide by zero problem since some partitions do not have documents containing a given word, but this can be solved by introducing a dummy document as the authors did. Another method that might be better at solving inconsistent results and the divide by zero problems is to have all partitions to communicate with their DF value. Then pass the merged DF value to all partitions to do the final IDF and TF-IDF value. Having all partitions to communicate with the DF value will guarantee a consistent DF value across all partitions and helps avoid a divide by zero problem as words in the keyword dictionary must appear in some documents in the whole collection. | ||
This paper treated the words in the different parts of a document equivalently, it might perform better if it gives different weights to the same word in different parts. For example, if a word appears in the title of the document, it usually shows it's a main topic of this document so we can put more weight on it to categorize. | |||
When discussing the potential processing advantages of this classification system for other types of text samples, has the effect of processing mixed samples (text and image or text and video) taken into consideration? IF not, in terms of text classification only, does it have an overwhelming advantage over traditional classification models? | |||
The preprocessing should also include <math>n</math>-gram tokenization for topic modelling because some topics are inherently two words, such as machine learning where if it is seen separately, it implies different topics. | |||
This system is very compute-intensive due to the large volumes of dictionaries that can be generated by processing large volumes of data. It would be nice to see how much data HDFS had to process and similarly how much time was saved by using Hadoop for data processing as opposed to centralized approach. | |||
This system can be improved further in terms of computation times by utilizing other big data framework MapReduce, that can also use HDFS, by parallelizing their computation across multiple nodes for K-means clustering as discussed in (Jin, et al) [5]. | |||
It's not exactly clear what method 3 (TFIDF-LDA) is doing, how is it performing TF-IDF on the topics? Also it seems like the preprocessing step only keeps 10/20/30 top words? This seems like an extremely low number especially in comparison with the LDA which has 10/20/30 topics - what is the reason for so strongly limiting the number of words? It would also be interesting to see if both key words and topics are necessary - an ablation study showing the significance of both would be interesting. | |||
It is better if the paper has an example with some topics on some research papers. Also it is better if we can visualize the distance between each research paper and the topic names | |||
I am interested in the first step of the general framework, which is the Paper Crawling step. Many conferences actually require the authors to indicate several key words that best describe a paper. For example, a database paper may have keywords such as "large-scale database management", "information retrieval", and "relational table mining". So in addition to crawling text from abstract, it may be more effective to crawl these keywords directly. Not only does this require less time, these keywords may also lead to better performance than the nouns extracted from the abstract section. I am also slightly concerned about the claim made in the paper that "Our methodologies can be applied to text outside of research papers". Research papers are usually carefully revised and well-structured. Extending the algorithm described in the paper to any kind of free-text could be difficult in practice. | |||
The paper has very meaningful motivation, since the association of research topics and finding all the relevant previous work is indeed a challenging task at the initials stage of the research. It is often easy to miss a relevant paper published years ago which might be crucial to your own work. However, the classification task that the author tested in this work is almost useless, as the classification is too high-level. The overall scheme of classifying paper between categories like "cloud bigdata" or "IoT privacy" is too general to be meaningful. It is simply classifying the primary field computer science into its direct subfield, while most researchers only work on a niche much narrower than the subfield. Most online paper database, including arxiv, takes care of the subfield and even subsubfield classifcation during the stage of submission, which leaves the author's system with limited applicabilty. What we truly need is an algorithm able to classify and cluster papers based on detailed research topics and methodology. | |||
It would be better if the author could provide some application or example of the research algorithm in the real world. It would be helpful for the readers to understand the algorithm. | |||
The summary clearly goes through the model framework well, starting from data-preprocessing, prediction, and testing. It can be enhanced by applying this model to other similar use-cases and how well the prediction goes. | |||
It will be better if their is a comparison on the BM25 algorithm v.s. TF-IDF, which is usually get compared in IR papers | |||
The paper misses the details on subjects of research papers used to perform classifications. If the majority of research papers were about one subject, it could potentially produce biased results. | |||
The paper omits the details of the reason why Method 3 for constructing the Keyword dictionaries requires the least number of k-clusters as method 3 is a combination of methods 1 and 2. It would be of interest to investigate why Method 3 uses so little clusters (in comparison) as it seemed to be the most accurate of the 3 methods. (Also the graph comparing the results could be improved by using a variety of different hues of colours as it is difficult to distinguish some scores such as TFIDF_30 and TFIDF-LDA_30) | |||
The TF-IDF is interesting as it provides a normalized method to extract the most frequent term contained in the paper, while this method still has spaces of improvements. For example, in some machine learning papers, where special operations have to be done on the datasets, the name of dataset may appear multiple times within the paper. In fact, the main theme of the paper is on the novel machine learning algorithm, which may only be mentioned once. In that case, mis-predictions may occur, and a possible improvement here is to add weights to keywords appearing in each section. i.e the most frequent word in Abstract will have more weights than the most frequent word in Introduction. | |||
In my opinion, the paper glosses over a few technicalities. First, how does the proposed algorithm deal with subgroups and nested groups. The paper is assuming only one level of sorting, which may work for a sufficiently unique set of paper, but since the problem is meant to be generalized, many papers will have to have multi-level sorts. For example, the category 'machine learning' can be further divided into 'supervised' and 'unsupervised'. Is the algorithm able to handle this or would it create 2 groups (i.e. ML-supervised and ML-unsupervised)? Second, a popular LDA model is available through the gensim package which utilizes relevancy and saliency metrics - how does that factor into the quality of the topics? Third, what is the motivation in using TF-IDF scores for clustering? In my experience, using Word2Vec and BERT has been the industry standard for obtaining vectors to perform clustering on text. | |||
When working with a larger data set, Spark might be more efficient than Hadoop. Working with natural language, the preprocessing is very important which might significantly determine the accuracy of the results. Therefore, different preprocessing techniques should be used to have a comparison. Lastly, PCA T-SNE might be a good way to visualize the result data. | |||
The paper illustrated and interpreted a lot of plots, but this summary is not plot heavy. There is a plot showing different elbow methods when method 3 are used. With different number of keywords and topics, the relationship between elbow and number of clusters is changing, the plot looks a lot steeper to be specific. Also, another thing I would like to mention is that, there may be computer science audience reading the summary, it is a good idea to put the graph that talks about map-reduce algorithm in the summary. This way the algorithm is more clearly explained, and people can get an idea of how the parameters function in the algorithm. | |||
=References= | =References= | ||
Blei DM, el. (2003). Latent Dirichlet allocation. J Mach Learn Res 3:993–1022 | [1] Blei DM, el. (2003). Latent Dirichlet allocation. J Mach Learn Res 3:993–1022 | ||
[2] Gil, JM, Kim, SW. (2019). Research paper classification systems based on TF-IDF and LDA schemes. ''Human-centric Computing and Information Sciences'', 9, 30. https://doi.org/10.1186/s13673-019-0192-7 | |||
[3] Liu, S. (2019, January 11). Dirichlet distribution Motivating LDA. Retrieved November 2020, from https://towardsdatascience.com/dirichlet-distribution-a82ab942a879 | |||
[4] Serrano, L. (Director). (2020, March 18). Latent Dirichlet Allocation (Part 1 of 2) [Video file]. Retrieved 2020, from https://www.youtube.com/watch?v=T05t-SqKArY | |||
[5] Jin, Cui, Yu. (2016). A New Parallelization Method for K-means. https://arxiv.org/ftp/arxiv/papers/1608/1608.06347.pdf | |||
[6] Kaufman, L., & Rousseeuw, P. J. (2005). Graphical Output Concerning Each Clustering. In Finding groups in data : An introduction to cluster analysis (pp. 84-85). Hoboken, New Jersey: John Wiley & Sons. doi:10.1002/9780470316801 |
Latest revision as of 04:18, 15 December 2020
Presented by
Jill Wang, Junyi (Jay) Yang, Yu Min (Chris) Wu, Chun Kit (Calvin) Li
Introduction
With the increasing advance of computer science and information technology, an increasingly overwhelming number of papers have been published. Due to the great volume, it has become incredibly hard to find and categorize papers. Typically this is a very time consuming activity. This paper introduces a paper classification system that utilizes the Term Frequency-Inverse Document Frequency (TF-IDF), Latent Dirichlet Allocation (LDA), and K-means clustering. The most important technology the system used to process big data is the Hadoop Distributed File System (HDFS). The system is highly fault-tolerant and can be deployed on low-cost hardware. It can handle quantitatively complex research paper classification problems efficiently and accurately.
General Framework
The paper classification system classifies research papers based on the abstracts given that the core of most papers is presented in the abstracts.
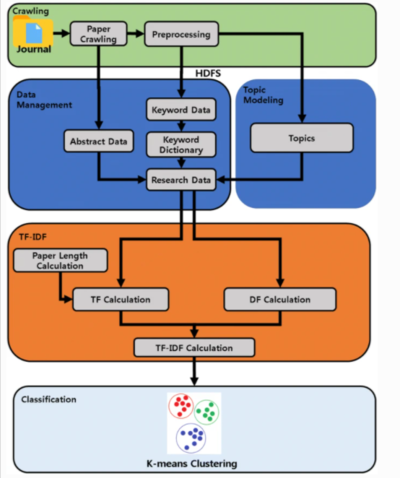
- Paper Crawling
Collects abstracts and keywords from research papers published during a given period
- Preprocessing
- Removes stop words in the papers crawled, in which only nouns are extracted from the papers
- generates a keyword dictionary, keeping only the top-N keywords with the highest frequencies
- Topic Modelling
Use the LDA to group the keywords into topics
- Paper Length Calculation
Calculates the total number of occurrences of words to prevent an unbalanced TF values caused by the various length of abstracts using the map-reduce algorithm
- Word Frequency Calculation
Calculates the Term Frequency (TF) values which represent the frequency of keywords in a research paper
- Document Frequency Calculation
Calculates the Document Frequency (DF) values which represents the frequency of keywords in a collection of research papers. The higher the DF value, the lower the importance of a keyword.
- Term frequency-inverse document frequency (TF-IDF) calculation
Calculates the inverse of the DF which represents the importance of a keyword.
- Paper Classification
Classify papers by topics and similar subjects using the K-means clustering algorithm.
Technologies
The HDFS with a Hadoop cluster composed of one master node, one sub-node, and four data nodes is what is used to process the massive paper data. Hadoop-2.6.5 version in Java is what is used to perform the TF-IDF calculation. Spark MLlib is what is used to perform the LDA. The Scikit-learn library is what is used to perform the K-means clustering.
HDFS
Hadoop Distributed File System (HDFS) was used to process big data in this system. HFDS has been shown to process big data rapidly and stably with high scalability which makes it a perfect choice for this problem. What Hadoop does is to break a big collection of data into different partitions and pass each partition to one individual processor. Each processor will only have information about the partition of data it has received.
In this summary, we are going to focus on introducing the main algorithms of what this system uses, namely LDA, TF-IDF, and K-Means.
Data Preprocessing
Crawling of Abstract Data
Under the assumption that audiences tend to first read the abstract of a paper to gain an overall understanding of the material, it is reasonable to assume the abstract section includes “core words” that can be used to effectively classify a paper's subject.
An abstract is crawled to have its stop words removed. Stop words are words that are usually ignored by search engines, such as “the”, “a”, and etc. Afterward, nouns are extracted, as a more condensed representation for efficient analysis.
This is managed on HDFS. The TF-IDF value of each paper is calculated through map-reduce, an easy-to-use programming model and implementation for processing and generating large data sets. The user must specify (i) a map procedure, that filters and sorts the input data to produce a set of intermediate key/value pairs and (ii) a reduce function, which performs a summary operation on the intermediate values with the same key and returns a smaller set of output key/value pairs. The MapReduce interface enables this process by grouping the intermediate values with the same key and passing them as input to the reduce function. For example, one could count the number of times various words appear in a large number of documents by setting your map procedure to count the number of occurrences of each word in a single document, and your reduce function to sum all counts of a given word [1].
Managing Paper Data
To construct an effective keyword dictionary using abstract data and keywords data in all of the crawled papers, the authors categorized keywords with similar meanings using a single representative keyword. The approach is called stemming, which is common in cleaning data, where words are reduced to their word stem. An example is "running" and "ran" would be reduced to "run". 1394 keyword categories are extracted, which is still too much to compute. Hence, only the top 30 keyword categories are used.
Topic Modeling Using LDA
Latent Dirichlet allocation (LDA) is a generative probabilistic model that views documents as random mixtures over latent topics. Each topic is a distribution over words, and the goal is to extract these topics from documents.
LDA estimates the topic-word distribution [math]\displaystyle{ P\left(t | z\right) }[/math] (probability of word "z" having topic "t") and the document-topic distribution [math]\displaystyle{ P\left(z | d\right) }[/math] (probability of finding word "z" within a given document "d") using Dirichlet priors for the distributions with a fixed number of topics. For each document, obtain a feature vector:
\[F = \left( P\left(z_1 | d\right), P\left(z_2 | d\right), \cdots, P\left(z_k | d\right) \right)\]
In the paper, authors extract topics from preprocessed paper to generate three kinds of topic sets, each with 10, 20, and 30 topics respectively. The following is a table of the 10 topic sets of highest frequency keywords.
LDA Intuition
LDA uses the Dirichlet priors of the Dirichlet distribution, which allows the algorithm to model a probability distribution over prior probability distributions of words and topics. The following picture illustrates 2-simplex Dirichlet distributions with different alpha values, one for each corner of the triangles.
Simplex is a generalization of the notion of a triangle in k-1 dimension where k is the number of classes. For example, if you wish to classify essays into three groups, English, History and Math then the simplex would be a 2 dimension triangle, if you add philosophy as one of your potential class, then we would need a tetrahedron in 3 deminsion. In Dirichlet distribution, each parameter will be represented by a corner in simplex, so adding additional parameters implies increasing the dimensions of simplex. As illustrated, when alphas are smaller than 1 the distribution is dense at the corners. When the alphas are greater than 1 the distribution is dense at the centers.
The following illustration shows an example LDA with 3 topics, 4 words and 7 documents.
In the left diagram, there are three topics, hence it is a 2-simplex. In the right diagram there are four words, hence it is a 3-simplex. LDA essentially adjusts parameters in Dirichlet distributions and multinomial distributions (represented by the points), such that, in the left diagram, all the yellow points representing documents and, in the right diagram, all the points representing topics, are as close to a corner as possible. In other words, LDA finds topics for documents and also finds words for topics. At the end topic-word distribution [math]\displaystyle{ P\left(t | z\right) }[/math] and the document-topic distribution [math]\displaystyle{ P\left(z | d\right) }[/math] are produced.
Term Frequency Inverse Document Frequency (TF-IDF) Calculation
TF-IDF is widely used to evaluate the importance of a set of words in the fields of information retrieval and text mining. It is a combination of term frequency (TF) and inverse document frequency (IDF). The idea behind this combination is
- It evaluates the importance of a word within a document
- It evaluates the importance of the word among the collection of all documents
The inverse of the document frequency accounts for the fact that term frequency will naturally increase as document frequency increases. Thus IDF is needed to counteract a word's TF to give an accurate representation of a word's importance.
The TF-IDF formula has the following form:
\[TF-IDF_{i,j} = TF_{i,j} \times IDF_{i}\]
where i stands for the [math]\displaystyle{ i^{th} }[/math] word and j stands for the [math]\displaystyle{ j^{th} }[/math] document.
Term Frequency (TF)
TF evaluates the percentage of a given word in a document. Thus, TF value indicates the importance of a word. The TF has a positive relation with the importance.
In this paper, we only calculate TF for words in the keyword dictionary obtained. For a given keyword i, [math]\displaystyle{ TF_{i,j} }[/math] is the number of times word i appears in document j divided by the total number of words in document j.
The formula for TF has the following form:
\[TF_{i,j} = \frac{n_{i,j} }{\sum_k n_{k,j} }\]
where i stands for the [math]\displaystyle{ i^{th} }[/math] word, j stands for the [math]\displaystyle{ j^{th} }[/math] document, [math]\displaystyle{ n_{i,j} }[/math] stands for the number of times words [math]\displaystyle{ t_i }[/math] appear in document [math]\displaystyle{ d_j }[/math] and [math]\displaystyle{ \sum_k n_{k,j} }[/math] stands for total number of occurence of words in document [math]\displaystyle{ d_j }[/math].
Note that the denominator is the total number of words remaining in document j after crawling.
Document Frequency (DF)
DF evaluates the percentage of documents that contain a given word over the entire collection of documents. Thus, the higher DF value is, the less important the word is.
[math]\displaystyle{ DF_{i} }[/math] is the number of documents in the collection with word i divided by the total number of documents in the collection. The formula for DF has the following form:
\[DF_{i} = \frac{|d_k \in D: n_{i,k} > 0|}{|D|}\]
where [math]\displaystyle{ n_{i,k} }[/math] is the number of times word i appears in document k, |D| is the total number of documents in the collection.
Since DF and the importance of the word have an inverse relation, we use inverse document frequency (IDF) instead of DF.
Inverse Document Frequency (IDF)
In this paper, IDF is calculated in a log scale. Since we will receive a large number of documents, i.e, we will have a large |D|
The formula for IDF has the following form:
\[IDF_{i} = log\left(\frac{|D|}{|\{d_k \in D: n_{i,k} > 0\}|}\right)\]
As mentioned before, we will use HDFS. The actual formula applied is:
\[IDF_{i} = log\left(\frac{|D|+1}{|\{d_k \in D: n_{i,k} > 0\}|+1}\right)\]
The inverse document frequency gives a measure of how rare a certain term is in a given document corpus.
Paper Classification Using K-means Clustering
K-means clustering is an unsupervised classification algorithm that groups similar data into the same class. It is an efficient and simple method that can be applied to different types of data attributes. It is also flexible enough to handle various kinds of noise and outliers.
Given a set of [math]\displaystyle{ d }[/math] by [math]\displaystyle{ n }[/math] dataset [math]\displaystyle{ \mathbf{X} = \left[ \mathbf{x}_1 \cdots \mathbf{x}_n \right] }[/math], the algorithm will assign each [math]\displaystyle{ \mathbf{x}_j }[/math] into [math]\displaystyle{ k }[/math] different clusters based on the characteristics of [math]\displaystyle{ \mathbf{x}_j }[/math] itself.
Moreover, when assigning data into a cluster, the algorithm will also try to minimise the distances between the data and the centre of the cluster which the data belongs to. That is, k-means clustering will minimize the sum of square error:
\begin{align*} min \sum_{i=1}^{k} \sum_{j \in C_i} ||x_j - \mu_i||^2 \end{align*}
where
- [math]\displaystyle{ k }[/math]: the number of clusters
- [math]\displaystyle{ C_i }[/math]: the [math]\displaystyle{ i^th }[/math] cluster
- [math]\displaystyle{ x_j }[/math]: the [math]\displaystyle{ j^th }[/math] data in the [math]\displaystyle{ C_i }[/math]
- [math]\displaystyle{ mu_i }[/math]: the centroid of [math]\displaystyle{ C_i }[/math]
- [math]\displaystyle{ ||x_j - \mu_i||^2 }[/math]: the Euclidean distance between [math]\displaystyle{ x_j }[/math] and [math]\displaystyle{ \mu_i }[/math]
K-means Clustering algorithm, an unsupervised algorithm, is chosen because of its advantages to deal with different types of attributes, to run with minimal requirement of domain knowledge, to deal with noise and outliers, to realize clusters with similarities.
Since the goal for this paper is to classify research papers and group papers with similar topics based on keywords, the paper uses the K-means clustering algorithm. The algorithm first computes the cluster centroid for each group of papers with a specific topic. Then, it will assign a paper into a cluster based on the Euclidean distance between the cluster centroid and the paper’s TF-IDF value.
However, different values of [math]\displaystyle{ k }[/math] (the number of clusters) will return different clustering results. Therefore, it is important to define the number of clusters before clustering. For example, in this paper, the authors choose to use the Elbow scheme to determine the value of [math]\displaystyle{ k }[/math]. The Elbow scheme is a somewhat subjective way of choosing an optimal [math]\displaystyle{ k }[/math] that involves plotting the average of the squared distances from the cluster centers of the respective clusters (distortion) as a function of [math]\displaystyle{ k }[/math] and choosing a [math]\displaystyle{ k }[/math] at which point the decrease in distortion is outweighed by the increase in complexity. Also, to measure the performance of clustering, the authors decide to use the Silhouette scheme. The Silhouette scheme is a measure of how well the objects lie within each cluster. Silhouette scores lie from -1 to 1. A positive score indicates that the object is well-matched with its own cluster, while a negative score indicates the opposite (Kaufman & Rousseeuw, 2005). The results of clustering are validated if the Silhouette scheme returns a value greater than [math]\displaystyle{ 0.5 }[/math].
System Testing Results
In this paper, the dataset has 3264 research papers from the Future Generation Computer System (FGCS) journal between 1984 and 2017. For constructing keyword dictionaries for each paper, the authors have introduced three methods as shown below:
Then, the authors use the Elbow scheme to define the number of clusters for each method with different numbers of keywords before running the K-means clustering algorithm. The results are shown below:
According to Table 4, there is a positive correlation between the number of keywords and the number of clusters. In addition, method 3 combines the advantages for both method 1 and method 2; thus, method 3 requires the least clusters in total. On the other hand, the wrong keywords might be presented in papers; hence, it might not be possible to group papers with similar subjects correctly by using method 1 and so method 1 needs the most number of clusters in total.
Next, the Silhouette scheme had been used for measuring the performance for clustering. The average of the Silhouette values for each method with different numbers of keywords are shown below:
Since the clustering is validated if the Silhouette’s value is greater than 0.5, for methods with 10 and 30 keywords, the K-means clustering algorithm produces good results.
To evaluate the accuracy of the classification system in this paper, the authors use the F-Score. The authors execute 5 times of experiment and use 500 randomly selected research papers for each trial. The following histogram shows the average value of F-Score for the three methods and different numbers of keywords:
Note that “TFIDF” means method 1, “LDA” means method 2, and “TFIDF-LDA” means method 3. The number 10, 20, and 30 after each method is the number of keywords the method has used. According to the histogram above, method 3 has the highest F-Score values than the other two methods with different numbers of keywords. Therefore, the classification system is most accurate when using method 3 as it combines the advantages for both method 1 and method 2.
Conclusion
This paper introduces a classification system that classifies research papers into different topics by using TF-IDF and LDA scheme with K-means clustering algorithm. The experimental results showed that the proposed system could classify the papers with similar subjects according to the keywords extracted from the abstracts of papers. In particular, when a keyword dictionary with both of the keywords extracted from the abstracts and the topics extracted by the LDA scheme is used, the classification system has a better clustering performance and higher F-Score values. The authors emphasized that the system can be implemented efficiently on high-performance computing infrastructure, using industry-standard technologies. This system allows users to search for the papers they want quickly and with the most productivity.
Furthermore, this classification system might also be used in different types of texts (e.g., documents, tweets, etc.) instead of only classifying research papers.
Critique
In this paper, DF values are calculated within each partition. This results that for each partition, DF value for a given word will vary and may have an inconsistent result for different partition methods. As mentioned above, there might be a divide by zero problem since some partitions do not have documents containing a given word, but this can be solved by introducing a dummy document as the authors did. Another method that might be better at solving inconsistent results and the divide by zero problems is to have all partitions to communicate with their DF value. Then pass the merged DF value to all partitions to do the final IDF and TF-IDF value. Having all partitions to communicate with the DF value will guarantee a consistent DF value across all partitions and helps avoid a divide by zero problem as words in the keyword dictionary must appear in some documents in the whole collection.
This paper treated the words in the different parts of a document equivalently, it might perform better if it gives different weights to the same word in different parts. For example, if a word appears in the title of the document, it usually shows it's a main topic of this document so we can put more weight on it to categorize.
When discussing the potential processing advantages of this classification system for other types of text samples, has the effect of processing mixed samples (text and image or text and video) taken into consideration? IF not, in terms of text classification only, does it have an overwhelming advantage over traditional classification models?
The preprocessing should also include [math]\displaystyle{ n }[/math]-gram tokenization for topic modelling because some topics are inherently two words, such as machine learning where if it is seen separately, it implies different topics.
This system is very compute-intensive due to the large volumes of dictionaries that can be generated by processing large volumes of data. It would be nice to see how much data HDFS had to process and similarly how much time was saved by using Hadoop for data processing as opposed to centralized approach.
This system can be improved further in terms of computation times by utilizing other big data framework MapReduce, that can also use HDFS, by parallelizing their computation across multiple nodes for K-means clustering as discussed in (Jin, et al) [5].
It's not exactly clear what method 3 (TFIDF-LDA) is doing, how is it performing TF-IDF on the topics? Also it seems like the preprocessing step only keeps 10/20/30 top words? This seems like an extremely low number especially in comparison with the LDA which has 10/20/30 topics - what is the reason for so strongly limiting the number of words? It would also be interesting to see if both key words and topics are necessary - an ablation study showing the significance of both would be interesting.
It is better if the paper has an example with some topics on some research papers. Also it is better if we can visualize the distance between each research paper and the topic names
I am interested in the first step of the general framework, which is the Paper Crawling step. Many conferences actually require the authors to indicate several key words that best describe a paper. For example, a database paper may have keywords such as "large-scale database management", "information retrieval", and "relational table mining". So in addition to crawling text from abstract, it may be more effective to crawl these keywords directly. Not only does this require less time, these keywords may also lead to better performance than the nouns extracted from the abstract section. I am also slightly concerned about the claim made in the paper that "Our methodologies can be applied to text outside of research papers". Research papers are usually carefully revised and well-structured. Extending the algorithm described in the paper to any kind of free-text could be difficult in practice.
The paper has very meaningful motivation, since the association of research topics and finding all the relevant previous work is indeed a challenging task at the initials stage of the research. It is often easy to miss a relevant paper published years ago which might be crucial to your own work. However, the classification task that the author tested in this work is almost useless, as the classification is too high-level. The overall scheme of classifying paper between categories like "cloud bigdata" or "IoT privacy" is too general to be meaningful. It is simply classifying the primary field computer science into its direct subfield, while most researchers only work on a niche much narrower than the subfield. Most online paper database, including arxiv, takes care of the subfield and even subsubfield classifcation during the stage of submission, which leaves the author's system with limited applicabilty. What we truly need is an algorithm able to classify and cluster papers based on detailed research topics and methodology.
It would be better if the author could provide some application or example of the research algorithm in the real world. It would be helpful for the readers to understand the algorithm.
The summary clearly goes through the model framework well, starting from data-preprocessing, prediction, and testing. It can be enhanced by applying this model to other similar use-cases and how well the prediction goes.
It will be better if their is a comparison on the BM25 algorithm v.s. TF-IDF, which is usually get compared in IR papers
The paper misses the details on subjects of research papers used to perform classifications. If the majority of research papers were about one subject, it could potentially produce biased results.
The paper omits the details of the reason why Method 3 for constructing the Keyword dictionaries requires the least number of k-clusters as method 3 is a combination of methods 1 and 2. It would be of interest to investigate why Method 3 uses so little clusters (in comparison) as it seemed to be the most accurate of the 3 methods. (Also the graph comparing the results could be improved by using a variety of different hues of colours as it is difficult to distinguish some scores such as TFIDF_30 and TFIDF-LDA_30)
The TF-IDF is interesting as it provides a normalized method to extract the most frequent term contained in the paper, while this method still has spaces of improvements. For example, in some machine learning papers, where special operations have to be done on the datasets, the name of dataset may appear multiple times within the paper. In fact, the main theme of the paper is on the novel machine learning algorithm, which may only be mentioned once. In that case, mis-predictions may occur, and a possible improvement here is to add weights to keywords appearing in each section. i.e the most frequent word in Abstract will have more weights than the most frequent word in Introduction.
In my opinion, the paper glosses over a few technicalities. First, how does the proposed algorithm deal with subgroups and nested groups. The paper is assuming only one level of sorting, which may work for a sufficiently unique set of paper, but since the problem is meant to be generalized, many papers will have to have multi-level sorts. For example, the category 'machine learning' can be further divided into 'supervised' and 'unsupervised'. Is the algorithm able to handle this or would it create 2 groups (i.e. ML-supervised and ML-unsupervised)? Second, a popular LDA model is available through the gensim package which utilizes relevancy and saliency metrics - how does that factor into the quality of the topics? Third, what is the motivation in using TF-IDF scores for clustering? In my experience, using Word2Vec and BERT has been the industry standard for obtaining vectors to perform clustering on text.
When working with a larger data set, Spark might be more efficient than Hadoop. Working with natural language, the preprocessing is very important which might significantly determine the accuracy of the results. Therefore, different preprocessing techniques should be used to have a comparison. Lastly, PCA T-SNE might be a good way to visualize the result data.
The paper illustrated and interpreted a lot of plots, but this summary is not plot heavy. There is a plot showing different elbow methods when method 3 are used. With different number of keywords and topics, the relationship between elbow and number of clusters is changing, the plot looks a lot steeper to be specific. Also, another thing I would like to mention is that, there may be computer science audience reading the summary, it is a good idea to put the graph that talks about map-reduce algorithm in the summary. This way the algorithm is more clearly explained, and people can get an idea of how the parameters function in the algorithm.
References
[1] Blei DM, el. (2003). Latent Dirichlet allocation. J Mach Learn Res 3:993–1022
[2] Gil, JM, Kim, SW. (2019). Research paper classification systems based on TF-IDF and LDA schemes. Human-centric Computing and Information Sciences, 9, 30. https://doi.org/10.1186/s13673-019-0192-7
[3] Liu, S. (2019, January 11). Dirichlet distribution Motivating LDA. Retrieved November 2020, from https://towardsdatascience.com/dirichlet-distribution-a82ab942a879
[4] Serrano, L. (Director). (2020, March 18). Latent Dirichlet Allocation (Part 1 of 2) [Video file]. Retrieved 2020, from https://www.youtube.com/watch?v=T05t-SqKArY
[5] Jin, Cui, Yu. (2016). A New Parallelization Method for K-means. https://arxiv.org/ftp/arxiv/papers/1608/1608.06347.pdf
[6] Kaufman, L., & Rousseeuw, P. J. (2005). Graphical Output Concerning Each Clustering. In Finding groups in data : An introduction to cluster analysis (pp. 84-85). Hoboken, New Jersey: John Wiley & Sons. doi:10.1002/9780470316801