Surround Vehicle Motion Prediction: Difference between revisions
(91 intermediate revisions by 48 users not shown) | |||
Line 1: | Line 1: | ||
DROCC: Surround Vehicle Motion Prediction Using LSTM-RNN for Motion Planning of Autonomous Vehicles at Multi-Lane Turn Intersections | DROCC: '''Surround Vehicle Motion Prediction Using LSTM-RNN for Motion Planning of Autonomous Vehicles at Multi-Lane Turn Intersections''' | ||
== Presented by == | == Presented by == | ||
Mushi Wang, Siyuan Qiu, Yan Yu | Mushi Wang, Siyuan Qiu, Yan Yu | ||
Line 5: | Line 5: | ||
== Introduction == | == Introduction == | ||
This paper presents a surrounding vehicle motion prediction algorithm for multi-lane turn intersections using a Long Short-Term Memory (LSTM) | This paper presents a surrounding vehicle motion prediction algorithm for multi-lane turn intersections using a Long Short-Term Memory-based (LSTM) Recurrent Neural Network (RNN). More specifically, it focused on the improvement of in-lane target recognition and achieving human-like acceleration decisions at multi-lane turn intersections by introducing the learning-based target motion predictor and prediction-based motion predictor. A data-driven approach to predict the trajectory and velocity of surrounding vehicles on urban roads at multi-lane turn intersections was described. LSTM architecture, a specific kind of RNN capable of learning long-term dependencies, is designed to manage complex vehicle motions in multi-lane turn intersections. The results show that the forecaster improves the recognition time of the leading vehicle and contributes to the improvement of prediction ability. | ||
== Previous Work == | == Previous Work == | ||
There are 3 main challenges to achieving fully autonomous driving on urban roads, which are scene awareness, inferring other drivers’ intentions, and predicting their future motions. Researchers are developing prediction algorithms that can simulate a driver’s intuition to improve safety when autonomous vehicles and human drivers drive together. To predict driver behavior on urban road, there are 3 categories for motion prediction model: (1) physics-based (2) maneuver-based; and (3) interaction-aware. Physics-based models are simple and direct, which only consider the states of prediction vehicles kinematically. The advantage is that it has minimal computational burden among the three types. However, it is impossible to consider interactions between vehicles. Maneuver-based models consider the driver’s intention and classified them. By predicting the driver maneuver, the future trajectory can be predicted. Identifying similar behaviors in driving is able to infer different drivers' intentions which are stated to improve the prediction accuracy. However, it still an assistant to improve physics-based models. Recurrent Neural Network (RNN) is a type of approach proposed to infer driver intention in this paper. Interaction-aware models can reflect interactions between surrounding vehicles, and predict future motions of detected vehicles simultaneously as a scene. While the prediction algorithm is more complex in computation which often used in offline simulations. | The autonomous vehicle trajectory approaches previously used motion models like Constant Velocity and Constant Acceleration. These models are linear and are only able to handle straight motions. There are curvilinear models such as Constant Turn Rate and Velocity and Constant Turn Rate and Acceleration which handle rotations and more complex motions. Together with these models, Kalman Filter is used to predicting the vehicle trajectory. Kalman filtering is a common technique used in sensor fusion for state estimation that allows the vehicle's state to be predicted while taking into account the uncertainty associated with inputs and measurements. However, the performance of the Kalman Filter in predicting multi-step problems is not that good. Recurrent Neural Network performs significantly better than it. | ||
There are 3 main challenges to achieving fully autonomous driving on urban roads, which are scene awareness, inferring other drivers’ intentions, and predicting their future motions. Researchers are developing prediction algorithms that can simulate a driver’s intuition to improve safety when autonomous vehicles and human drivers drive together. To predict driver behavior on an urban road, there are 3 categories for the motion prediction model: (1) physics-based; (2) maneuver-based; and (3) interaction-aware. Physics-based models are simple and direct, which only consider the states of prediction vehicles kinematically. The advantage is that it has minimal computational burden among the three types. However, it is impossible to consider the interactions between vehicles. Maneuver-based models consider the driver’s intention and classified them. By predicting the driver maneuver, the future trajectory can be predicted. Identifying similar behaviors in driving is able to infer different drivers' intentions which are stated to improve the prediction accuracy. However, it still an assistant to improve physics-based models. | |||
Recurrent Neural Network (RNN) is a type of approach proposed to infer driver intention in this paper. Interaction-aware models can reflect interactions between surrounding vehicles, and predict future motions of detected vehicles simultaneously as a scene. While the prediction algorithm is more complex in computation which is often used in offline simulations. As Schulz et al. indicate, interaction models are very difficult to create as "predicting complete trajectories at once is challenging, as one needs to account for multiple hypotheses and long-term interactions between multiple agents" [6]. | |||
== Motivation == | == Motivation == | ||
Research results indicate that | Research results indicate that little research has been dedicated on predicting the trajectory of intersections. Research regarding intersections have previously concentrated only on maneuver-level predictions of activities such as crossing, stopping or making turns. Trajectory level prediction has also been mainly focused on structured environments that enforce classifiable behavior but has not been extensively researched in multi-lane turn intersections. This is important since different drivers have varied driving behaviors as they travel through intersections. | ||
Moreover, public data sets for analyzing driver behavior at intersections are not enough, and these data sets are not easy to collect. A model is needed to predict the various movements of the target around a multi-lane turning intersection. It is very necessary to design a motion predictor that can be used for real-time traffic. | |||
<center> | |||
[[ File:intersection.png |300px]] | |||
</center> | |||
== Framework == | == Framework == | ||
The LSTM-RNN-based motion predictor comprises three parts: (1) a data encoder; (2) an LSTM-based RNN; and (3) a data decoder | The LSTM-RNN-based motion predictor comprises of three parts: (1) a data encoder; (2) an LSTM-based RNN; and (3) a data decoder depicts the architecture of the surrounding target trajectory predictor. The proposed architecture uses a perception algorithm to estimate the state of surrounding vehicles, which relies on six scanners. The output predicts the state of the surrounding vehicles and is used to determine the expected longitudinal acceleration in the actual traffic at the intersection. The following image gives a visual representation of the model. | ||
<center>[[Image:Figure1_Yan.png|800px|]]</center> | <center>[[Image:Figure1_Yan.png|800px|]]</center> | ||
== LSTM-RNN based motion predictor == | == LSTM-RNN based motion predictor == | ||
=== Sensor Outputs === | |||
The input of the target perceptions is from the output of the sensors. The data collected in this article uses 6 different sensors with feature fusion to detect traffic in the range up to 100m: 1) LiDAR system outputs: Relative position, heading, velocity, and box size in local coordinates; 2) Around-View Monitoring (AVM) and 3)GPS outputs: acquire lanes, road marker, global position; 4) Gateway engine outputs: precise global position in urban road environment; 5) Micro-Autobox II and 6) a MDPS are used to control and actuate the subject. All data are stored in an industrial PC. | |||
=== Data === | === Data === | ||
The | Multi-lane turn intersections are the target roads in this paper. The dataset was collected using a human driven Autonomous Vehicle(AV) that was equipped with sensors to track motion the vehicle's surroundings. In addition, the motion sensors they used a front camera, Around-View-Monitor and GPS to acquire the lanes, road markers and global position. The data was collected in the urban roads of Gwanak-gu, Seoul, South Korea. The training model is generated from 484 tracks collected when driving through intersections in real traffic. The previous and subsequent states of a vehicle at a particular time can be extracted. After post-processing, the collected data, a total of 16,660 data samples were generated, including 11,662 training data samples, and 4,998 evaluation data samples. | ||
=== Motion predictor === | === Motion predictor === | ||
This article | This article proposes a data-driven method to predict the future movement of surrounding vehicles based on their previous movement, which is the sequential previous motion. The motion predictor based on the LSTM-RNN architecture in this work only uses information collected from sensors on autonomous vehicles, as shown in the figure below. The contribution of the network architecture of this study is that the future state of the target vehicle is used as the input feature for predicting the field of view. | ||
Line 31: | Line 44: | ||
==== Network architecture ==== | ==== Network architecture ==== | ||
RNN is an artificial neural network | A RNN is an artificial neural network that is suitable for use with sequential data because it has recurrent connections on its hidden nodes and thus, can retain its state or memory while processing the next input or sequence of inputs. For this reason, RNNs can be used to analyze time-series data where the pattern of the data depends on the time flow. This is an impossible task for traditional artificial neural networks, which assume the inputs are independent of one another. RNNs can also contain feedback loops that allow activations to flow alternately in the loop. | ||
In line with traditional neural networks, RNNs still suffer from the problem of vanishing gradients. An LSTM avoids this by making errors flow backward without a limit on the number of virtual layers through the use of forget gates. This property prevents errors from increasing or declining over time, which can make the network train improperly. The figure below shows the various layers of the LSTM-RNN and the number of units in each layer. This structure is determined by comparing the accuracy of 72 RNNs, which consist of a combination of four input sets and 18 network configurations. | |||
<center>[[Image:Figure8_Yan.png|800px|]]</center> | <center>[[Image:Figure8_Yan.png|800px|]]</center> | ||
==== Input and output features ==== | |||
In order to apply the motion predictor to the AV in motion, the speed of the data collection vehicle is added to the input sequence. The input sequence consists of relative X/Y position, relative heading angle, speed of surrounding target vehicles, and speed of data collection vehicles. The output sequence is the same as the input sequence, such as relative position, heading, and speed. | |||
==== Encoder and decoder ==== | ==== Encoder and decoder ==== | ||
In this study, authors introduced an encoder and decoder that process the input from the sensor and the output from the RNN, respectively. The encoder normalizes each component of the input data to rescale the data to mean 0 and standard deviation 1, while the decoder denormalizes the output data to use the same parameters as in the encoder to scale it back to the actual unit. | In this study, the authors introduced an encoder and decoder that process the input from the sensor and the output from the RNN, respectively. The encoder normalizes each component of the input data to rescale the data to mean 0 and standard deviation 1, while the decoder denormalizes the output data to use the same parameters as in the encoder to scale it back to the actual unit. | ||
==== | ==== Sequence length ==== | ||
The sequence length of RNN input and output is another important factor to improve prediction performance. In this study, 5, 10, 15, 20, 25, and 30 steps of 100 millisecond sampling times were compared, and 15 steps showed relatively accurate results, even among candidates The observation time is very short. | The sequence length of RNN input and output is another important factor to improve prediction performance. In this study, 5, 10, 15, 20, 25, and 30 steps of 100 millisecond sampling times were compared, and 15 steps showed relatively accurate results, even among candidates The observation time is very short. | ||
Line 53: | Line 66: | ||
\end{split} | \end{split} | ||
\end{equation*} | \end{equation*} | ||
where <math>k</math> and <math>t</math> are the prediction step index and time index, respectively; <math>x(k|t)</math> and <math>x_{ref} (k|t)</math> are the states and reference of the MPC problem, respectively; <math>x(k|t)</math> is composed of travel distance px and longitudinal velocity vx; <math>x_{ref} (k|t)</math> consists of reference travel distance <math>p_{x,ref}</math> and reference longitudinal velocity <math>v_{x,ref}</math> ; <math>u(k|t)</math> is the control input, which is the longitudinal acceleration command; <math>N_p</math> is the prediction horizon; and Q, R, and <math>R_{\Delta \mu}</math> are the weight matrices for states, input, and input derivative, respectively, and these weight matrices were tuned to obtain control inputs from the proposed controller that were as similar as possible to those of human-driven vehicles. | where <math>k</math> and <math>t</math> are the prediction step index and time index, respectively; <math>x(k|t)</math> and <math>x_{ref} (k|t)</math> are the states and reference of the MPC problem, respectively; <math>x(k|t)</math> is composed of travel distance px and longitudinal velocity vx; <math>x_{ref} (k|t)</math> consists of reference travel distance <math>p_{x,ref}</math> and reference longitudinal velocity <math>v_{x,ref}</math> ; <math>u(k|t)</math> is the control input, which is the longitudinal acceleration command; <math>N_p</math> is the prediction horizon; and Q, R, and <math>R_{\Delta \mu}</math> are the weight matrices for states, input, and input derivative, respectively, and these weight matrices were tuned to obtain control inputs from the proposed controller that were as similar as possible to those of human-driven vehicles. The weight matrices were used for the controller, for the proposed and conventional motion predictors. The dynamic constraints for the controller were defined as follors | ||
$$\begin{bmatrix} p_{x}(k+1|t)\\ v_{x}(k+1|t)\end{bmatrix} = \begin{bmatrix}1 & dt \\0 &1-dt/\tau \end{bmatrix}\begin{bmatrix} p_{x}(k|t)\\ v_{x}(k|t)\end{bmatrix} + \begin{bmatrix} 0 & dt/\tau\end{bmatrix}u(k|t) $$ | |||
The constraints of the control input are defined as follows: | The constraints of the control input are defined as follows: | ||
\begin{equation*} | \begin{equation*} | ||
Line 61: | Line 75: | ||
\end{split} | \end{split} | ||
\end{equation*} | \end{equation*} | ||
Where <math>u_{min}</math>, <math>u_{max}</math>and S are the minimum/maximum control input and maximum slew rate of input respectively. | |||
Determine the position and speed boundary based on the predicted state: | Determine the position and speed boundary based on the predicted state: | ||
\begin{equation*} | \begin{equation*} | ||
Line 86: | Line 102: | ||
\end{split} | \end{split} | ||
\end{equation*} | \end{equation*} | ||
<center>[[Image:Figure10.1_YanYu.png|500px|]]</center> | |||
The proposed model shows | The proposed model shows significantly fewer prediction errors compare to the based algorithms in terms of mean, | ||
standard deviation(STD), and root mean square error(RMSE). Meanwhile, the proposed model exhibits a bell shaped | standard deviation(STD), and root mean square error(RMSE). Meanwhile, the proposed model exhibits a bell-shaped | ||
curve with a close to zero mean, which indicates that the proposed algorithm's prediction of human divers' | |||
intensions are relatively precise. On the other hand, <math>e_{x,T_p}</math>, <math>e_{y,T_p}</math>, <math>e_{v,T_p}</math> are bounded within | intensions are relatively precise. On the other hand, <math>e_{x,T_p}</math>, <math>e_{y,T_p}</math>, <math>e_{v,T_p}</math> are bounded within | ||
reasonable levels. For instant, the three-sigma range of <math>e_{y,T_p}</math> is within the width of a lane. Therefore, | reasonable levels. For instant, the three-sigma range of <math>e_{y,T_p}</math> is within the width of a lane. Therefore, | ||
Line 97: | Line 114: | ||
==== Case study of a multi-lane left turn scenario ==== | ==== Case study of a multi-lane left turn scenario ==== | ||
The proposed method mimics a human driver better, by simulating a human driver's decision-making process. | The proposed method mimics a human driver better, by simulating a human driver's decision-making process. | ||
In a multi-lane left turn scenario, the proposed algorithm correctly predicted the trajectory of a target | In a multi-lane left turn scenario, the proposed algorithm correctly predicted the trajectory of a target vehicle, even when the target vehicle was not following the intersection guideline. | ||
vehicle, even when the target vehicle was not following the intersection guideline. | |||
==== Statistical analysis of motion planning application results ==== | ==== Statistical analysis of motion planning application results ==== | ||
Line 106: | Line 122: | ||
the surrounding target vehicles first appeared beyond the sensors’ region of interest boundaries. This means | the surrounding target vehicles first appeared beyond the sensors’ region of interest boundaries. This means | ||
that these cases took place sufficiently beyond the safety distance, and had little influence on determining | that these cases took place sufficiently beyond the safety distance, and had little influence on determining | ||
the | the behaviour of the subject vehicle. | ||
In order to compare the similarities between the results | <center>[[Image:Figure11_YanYu.png|500px|]]</center> | ||
and <math>a_{x, cmd}</math> are the human | In order to compare the similarities between the results from the proposed algorithm and human driving decisions, | ||
respectively. The proposed algorithm showed more similar results to human drivers’ decisions than | this article introduced another type of error, acceleration error <math>a_{x, error} = a_{x, human} - a_{x, cmd}</math>. where <math>a_{x, human}</math> | ||
and <math>a_{x, cmd}</math> are the human drivers' acceleration history and the command from the proposed algorithm, | |||
possesses limited ability to respond to different in-lane target | respectively. The proposed algorithm showed more similar results to human drivers’ decisions than the base | ||
model is efficient and | algorithm. <math>91.97\%</math> of the acceleration error lies in the region <math>\pm 1 m/s^2</math>. Moreover, the base algorithm | ||
possesses a limited ability to respond to different in-lane target behaviours in traffic flow. Hence, the proposed | |||
model is efficient and safe. | |||
== Conclusion == | == Conclusion == | ||
A surrounding vehicle motion predictor based on | A surrounding vehicle motion predictor based on an LSTM-RNN at multi-lane turn intersections was developed and its application in an autonomous vehicle was evaluated. The model was trained by using the data captured on the urban road in Seoul in MPC. The evaluation results showed precise prediction accuracy and so the algorithm is safe to be applied on an autonomous vehicle. Also, the comparison with the other three base algorithms (CV/Path, V_flow/Path, and CTRV) revealed the superiority of the proposed algorithm. The evaluation results showed precise prediction accuracy. In addition, the time-to-recognize in-lane targets within the intersection improved significantly over the performance of the base algorithms. The proposed algorithm was compared with human driving data, and it showed similar longitudinal acceleration. The motion predictor can be applied to path planners when AVs travel in unconstructed environments, such as multi-lane turn intersections. | ||
== Future works == | |||
This paper has identified several venues for future research, which include: | |||
1.Developing trajectory prediction algorithms using other machine learning algorithms, such as attention-aware neural networks. | 1.Developing trajectory prediction algorithms using other machine learning algorithms, such as attention-aware neural networks. | ||
Line 130: | Line 149: | ||
== Critiques == | == Critiques == | ||
The literature review is not sufficient. It should focus more on LSTM, RNN, and the study in different types of | The literature review is not sufficient. It should focus more on LSTM, RNN, and the study in different types of roads. Why the LSTM-RNN is used, and the background of the method is not stated clearly. There is a lack of concept so that it is difficult to distinguish between LSTM-RNN based motion predictor and motion planning. | ||
This is an interesting topic to discuss. This is a major topic for some famous vehicle companies such as Tesla, which now already has a good service called Autopilot to give self-driving and Motion Prediction. This summary can include more diagrams in architecture in the model to give readers a whole view of how the model looks like. Since it is using LSTM-RNN, include some pictures of the LSTM-RNN will be great. I think it will be interesting to discuss more applications by using this method, such as Airplane, boats. | |||
Autonomous driving is a very hot topic, and training the model with LSTM-RNN is also a meaningful topic to discuss. By the way, it would be an interesting approach to compare the performance of different algorithms or some other traditional motion planning algorithms like KF. | |||
There are some papers that discussed the accuracy of different models in vehicle predictions, such as Deep Kinematic Models for Kinematically Feasible Vehicle Trajectory Predictions[https://arxiv.org/pdf/1908.00219.pdf.] The LSTM didn't show good performance. They increased the accuracy by combing LSTM with an unconstrained model(UM) by adding an additional LSTM layer of size 128 that is used to recursively output positions instead of simultaneously outputting positions for all horizons. | |||
It may be better to provide the results of experiments to support the efficiency of LSTM-RNN, talk about the prediction of training and test sets, and compared it with other autonomous driving systems that exist in the world. | |||
The topic of surround vehicle motion prediction is analogous to the topic of autonomous vehicles. An example of an application of these frameworks would be the transportation services industry. Many companies, such as Lyft and Uber, have started testing their own commercial autonomous vehicles. | |||
It would be really helpful if some visualization or data summary can be provided to understand the content, such as the track of the car movement. | |||
The model should have been tested in other regions besides just Seoul, as driving behaviors can vary drastically from region to region. | |||
Understandably, a supervised learning problem should be evaluated on some test dataset. However, supervised learning techniques are inherently ill-suited for general planning problems. The test dataset was obtained from human driving data which is known to be extremely noisy as well as unpredictable when it comes to motion planning. It would be crucial to determine the successes of this paper based on the state-of-the-art reinforcement learning techniques. | |||
It would be better if the authors compared their method against other SOTA methods. Also one of the reasons motion planning is done using interpretable methods rather than black boxes (such as this model) is because it is hard to see where things go wrong and fix problems with the black box when they occur - this is something the authors should have also discussed. | |||
A future area of study is to combine other source of information such as signals from Lidar or car side cameras to make a better prediction model. | |||
It might be interesting and helpful to conduct some training and testing under different weather/environmental conditions, as it could provide more generalization to real-life driving scenarios. For example, foggy weather and evening (low light) conditions might affect the performance of sensors, and rainy weather might require a longer braking distance. | |||
This paper proposes an interesting, novel model prediction algorithm, using LSTM_RNN. However, since motion prediction in autonomous driving has great real-life impacts, I do believe that the evaluations of the algorithm should be more thorough. For example, more traditional motion planning algorithms such as multi-modal estimation and Kalman filters should be used as benchmarks. Moreover, the experiment results are based on Korean driving conditions only. Eastern and Western drivers can have very different driving patterns, so that should be addressed in the discussion section of the paper as well. | |||
The paper mentions that in the future, this research plans to learn the real life behaviour of automated vehicles. Seeing a possible improvement in road safety due to this research will be very interesting. | |||
This predictor is also possible to be applied in the traffic control system. | |||
This prediction model should consider various conditions that could happen in an intersection. However, normal prediction may not work when there is a traffic jam or in some crowded time periods like rush hours. | |||
It would be better that the author could provide more comparison between the LSTN-RNN algorithm and other traditional algorithm such as RNN or just LSTM. | |||
The paper has really good results for what they aimed to achieve. However for the future work it would also be nice to have various climates/weathers to be included in the Seoul dataset. I think it's also important to consider it as different climates/weather (such as snowy roads, or rain) would introduce more noisier data (camera's image processing) and the human drivers behaviour would change as well to adapt to the new environment. | |||
It would be good to have a future work section to discusses shortage of current algorithms and the possible improvement. | |||
The summary explains the whole process well, but is missing the small details among the steps. It would be better to explain concepts such as RNN, modelling procedure for first time users. | |||
This paper presents a nice method, but does not seem particularly well developed. I would have liked to see some more ablations on this particular choice of RNN, as there are more efficient variants such as GRU which show similar performance in other tasks while being more amenable to real-time inference. Furthermore, the multi-model aspect seems slightly ad-hoc, it would have been nice to see a more rigorous formulation similar to seen in some recent work by Zeng et al. from Uber ATG: https://arxiv.org/pdf/2008.06041.pdf. | |||
The data used for this paper contains driver information exclusively to the urban roads of Gwanak-gu Seoul, hence the data may contain an inherited bias as drivers around the rest of the country, let alone the rest of the world, will have different habits based on different environments. It would be interesting to see if this model can be applied to other cities around the world and exhibit similar results or would there be a need to tune it based off geographic location. | |||
Since the data is based on urban roads, It would be better to include the details on performance of the model on high traffic area vs low traffic urban area. It would also be interesting to see the performance of the model with many pedestrians. | |||
While it would be nice to read more on why the authors chose LSTM-RNN, the paper exhibits a potential way to improve autonomous vehicle performance. It would be interesting to see how an army of robots would behave when this paper's method is applied in robotics, since robots' motions also follow a trajectory. | |||
An interesting topic, but the paper and accompanying summary are missing some details that would improve understandability. With respect to the background of the topic, a more detailed explanation of trajectories in the case of driving would help to better motivate the research. The addition of benchmarks and comparisons to current industry standards (if they are published publicly) would help to contextualize the results of the LSTM-RNN. An area of further study is applying these techniques to different weather situations and driving patterns in different countries. How does this model perform in regions where driver's very loosely follow the laws of the road? Further, could the research be generalized for controlled and uncontrolled turn lanes, especially on roads with higher speed limits? | |||
In the past, LSTM is a very popular model in natural language. It is interesting to see how it is used for motion prediction. Although the result is not good enough, it is still a good start. In the future, more language models can be used to see how well they can perform to do a comparison such as BiLSTM, Transformer, and Bert. | |||
LSTM-RNNs are really incredibly useful for sequential learning. The RNN should be able to predict, not only when a vehicle in front of the motion sensors, but it's likelihood of staying in motion vs coming to a sudden stop. There is no details in this review on the running time of the algorithm. In a field where split seconds could be the difference between an accident and a brake, running time cannot be stressed enough. Whether or not it is truly capable of dealing with real time traffic cannot be judged without relevant data and results being shown. | |||
The prediction could be more flexible and be trained and tested with more complex cases. In real-world, unpredicted situations could always happen, and usual predictions might not be aware of those cases. | |||
We must notice that the model is not presenting the time data of training and prediction and adaptation to road conditions as it learns the history of conditions. This is an inherently flowed experiment as time is extremely important to how well a model is to predict and assess the movement of a moving vehicle. Even though a desired time gap is given, it is sufficient for a vehicle at moving speed. It does not give what does the model do as it realizes the prediction was wrong and the steps following after as sensors detect new information. | |||
This is rather late, but I find there is an important concept missing in section 8 of the summary. In the original article, although not explicitly stated, the author compared the difference in recognition timing between two different predictors. It was revealed later that the proposed algorithm only recognized cases later than the base algorithm did when the surrounding target vehicles first appeared beyond the sensors’ region of interest boundaries, which was illustrated using a plot. Although it is not as important as other contents contained in the same section, the plot is still a good evidence to show how the proposed algorithm's performance can be affected by the target vehicles' motion. | |||
== Reference == | == Reference == | ||
Line 144: | Line 217: | ||
[4] E. Strigel, D. Meissner, F. Seeliger, B. Wilking, and K. Dietmayer, “The Ko-PER intersection laserscanner and video dataset,” in Proc. 17th Int. IEEE Conf. Intell. Transp. Syst. (ITSC), Qingdao, China, 2014, pp. 1900–1901. | [4] E. Strigel, D. Meissner, F. Seeliger, B. Wilking, and K. Dietmayer, “The Ko-PER intersection laserscanner and video dataset,” in Proc. 17th Int. IEEE Conf. Intell. Transp. Syst. (ITSC), Qingdao, China, 2014, pp. 1900–1901. | ||
[5] Henggang Cui, Thi Nguyen, Fang-Chieh Chou, Tsung-Han Lin, Jeff Schneider, David Bradley, Nemanja Djuric: “Deep Kinematic Models for Kinematically Feasible Vehicle Trajectory Predictions”, 2019; [http://arxiv.org/abs/1908.00219 arXiv:1908.00219]. | |||
[6]Schulz, Jens & Hubmann, Constantin & Morin, Nikolai & Löchner, Julian & Burschka, Darius. (2019). Learning Interaction-Aware Probabilistic Driver Behavior Models from Urban Scenarios. 10.1109/IVS.2019.8814080. |
Latest revision as of 22:12, 14 December 2020
DROCC: Surround Vehicle Motion Prediction Using LSTM-RNN for Motion Planning of Autonomous Vehicles at Multi-Lane Turn Intersections
Presented by
Mushi Wang, Siyuan Qiu, Yan Yu
Introduction
This paper presents a surrounding vehicle motion prediction algorithm for multi-lane turn intersections using a Long Short-Term Memory-based (LSTM) Recurrent Neural Network (RNN). More specifically, it focused on the improvement of in-lane target recognition and achieving human-like acceleration decisions at multi-lane turn intersections by introducing the learning-based target motion predictor and prediction-based motion predictor. A data-driven approach to predict the trajectory and velocity of surrounding vehicles on urban roads at multi-lane turn intersections was described. LSTM architecture, a specific kind of RNN capable of learning long-term dependencies, is designed to manage complex vehicle motions in multi-lane turn intersections. The results show that the forecaster improves the recognition time of the leading vehicle and contributes to the improvement of prediction ability.
Previous Work
The autonomous vehicle trajectory approaches previously used motion models like Constant Velocity and Constant Acceleration. These models are linear and are only able to handle straight motions. There are curvilinear models such as Constant Turn Rate and Velocity and Constant Turn Rate and Acceleration which handle rotations and more complex motions. Together with these models, Kalman Filter is used to predicting the vehicle trajectory. Kalman filtering is a common technique used in sensor fusion for state estimation that allows the vehicle's state to be predicted while taking into account the uncertainty associated with inputs and measurements. However, the performance of the Kalman Filter in predicting multi-step problems is not that good. Recurrent Neural Network performs significantly better than it.
There are 3 main challenges to achieving fully autonomous driving on urban roads, which are scene awareness, inferring other drivers’ intentions, and predicting their future motions. Researchers are developing prediction algorithms that can simulate a driver’s intuition to improve safety when autonomous vehicles and human drivers drive together. To predict driver behavior on an urban road, there are 3 categories for the motion prediction model: (1) physics-based; (2) maneuver-based; and (3) interaction-aware. Physics-based models are simple and direct, which only consider the states of prediction vehicles kinematically. The advantage is that it has minimal computational burden among the three types. However, it is impossible to consider the interactions between vehicles. Maneuver-based models consider the driver’s intention and classified them. By predicting the driver maneuver, the future trajectory can be predicted. Identifying similar behaviors in driving is able to infer different drivers' intentions which are stated to improve the prediction accuracy. However, it still an assistant to improve physics-based models.
Recurrent Neural Network (RNN) is a type of approach proposed to infer driver intention in this paper. Interaction-aware models can reflect interactions between surrounding vehicles, and predict future motions of detected vehicles simultaneously as a scene. While the prediction algorithm is more complex in computation which is often used in offline simulations. As Schulz et al. indicate, interaction models are very difficult to create as "predicting complete trajectories at once is challenging, as one needs to account for multiple hypotheses and long-term interactions between multiple agents" [6].
Motivation
Research results indicate that little research has been dedicated on predicting the trajectory of intersections. Research regarding intersections have previously concentrated only on maneuver-level predictions of activities such as crossing, stopping or making turns. Trajectory level prediction has also been mainly focused on structured environments that enforce classifiable behavior but has not been extensively researched in multi-lane turn intersections. This is important since different drivers have varied driving behaviors as they travel through intersections. Moreover, public data sets for analyzing driver behavior at intersections are not enough, and these data sets are not easy to collect. A model is needed to predict the various movements of the target around a multi-lane turning intersection. It is very necessary to design a motion predictor that can be used for real-time traffic.
Framework
The LSTM-RNN-based motion predictor comprises of three parts: (1) a data encoder; (2) an LSTM-based RNN; and (3) a data decoder depicts the architecture of the surrounding target trajectory predictor. The proposed architecture uses a perception algorithm to estimate the state of surrounding vehicles, which relies on six scanners. The output predicts the state of the surrounding vehicles and is used to determine the expected longitudinal acceleration in the actual traffic at the intersection. The following image gives a visual representation of the model.
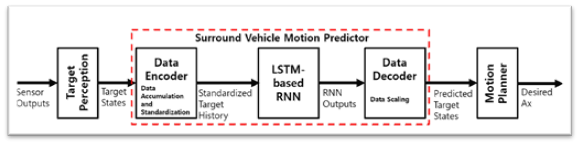
LSTM-RNN based motion predictor
Sensor Outputs
The input of the target perceptions is from the output of the sensors. The data collected in this article uses 6 different sensors with feature fusion to detect traffic in the range up to 100m: 1) LiDAR system outputs: Relative position, heading, velocity, and box size in local coordinates; 2) Around-View Monitoring (AVM) and 3)GPS outputs: acquire lanes, road marker, global position; 4) Gateway engine outputs: precise global position in urban road environment; 5) Micro-Autobox II and 6) a MDPS are used to control and actuate the subject. All data are stored in an industrial PC.
Data
Multi-lane turn intersections are the target roads in this paper. The dataset was collected using a human driven Autonomous Vehicle(AV) that was equipped with sensors to track motion the vehicle's surroundings. In addition, the motion sensors they used a front camera, Around-View-Monitor and GPS to acquire the lanes, road markers and global position. The data was collected in the urban roads of Gwanak-gu, Seoul, South Korea. The training model is generated from 484 tracks collected when driving through intersections in real traffic. The previous and subsequent states of a vehicle at a particular time can be extracted. After post-processing, the collected data, a total of 16,660 data samples were generated, including 11,662 training data samples, and 4,998 evaluation data samples.
Motion predictor
This article proposes a data-driven method to predict the future movement of surrounding vehicles based on their previous movement, which is the sequential previous motion. The motion predictor based on the LSTM-RNN architecture in this work only uses information collected from sensors on autonomous vehicles, as shown in the figure below. The contribution of the network architecture of this study is that the future state of the target vehicle is used as the input feature for predicting the field of view.
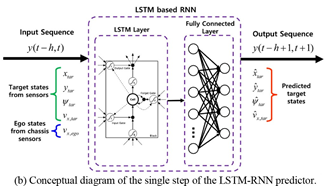
Network architecture
A RNN is an artificial neural network that is suitable for use with sequential data because it has recurrent connections on its hidden nodes and thus, can retain its state or memory while processing the next input or sequence of inputs. For this reason, RNNs can be used to analyze time-series data where the pattern of the data depends on the time flow. This is an impossible task for traditional artificial neural networks, which assume the inputs are independent of one another. RNNs can also contain feedback loops that allow activations to flow alternately in the loop.
In line with traditional neural networks, RNNs still suffer from the problem of vanishing gradients. An LSTM avoids this by making errors flow backward without a limit on the number of virtual layers through the use of forget gates. This property prevents errors from increasing or declining over time, which can make the network train improperly. The figure below shows the various layers of the LSTM-RNN and the number of units in each layer. This structure is determined by comparing the accuracy of 72 RNNs, which consist of a combination of four input sets and 18 network configurations.

Input and output features
In order to apply the motion predictor to the AV in motion, the speed of the data collection vehicle is added to the input sequence. The input sequence consists of relative X/Y position, relative heading angle, speed of surrounding target vehicles, and speed of data collection vehicles. The output sequence is the same as the input sequence, such as relative position, heading, and speed.
Encoder and decoder
In this study, the authors introduced an encoder and decoder that process the input from the sensor and the output from the RNN, respectively. The encoder normalizes each component of the input data to rescale the data to mean 0 and standard deviation 1, while the decoder denormalizes the output data to use the same parameters as in the encoder to scale it back to the actual unit.
Sequence length
The sequence length of RNN input and output is another important factor to improve prediction performance. In this study, 5, 10, 15, 20, 25, and 30 steps of 100 millisecond sampling times were compared, and 15 steps showed relatively accurate results, even among candidates The observation time is very short.
Motion planning based on surrounding vehicle motion prediction
In daily driving, experienced drivers will predict possible risks based on observations of surrounding vehicles, and ensure safety by changing behaviors before the risks occur. In order to achieve a human-like motion plan, based on the model predictive control (MPC) method, a prediction-based motion planner for autonomous vehicles is designed, which takes into account the driver’s future behavior. The cost function of the motion planner is determined as follows: \begin{equation*} \begin{split} J = & \sum_{k=1}^{N_p} (x(k|t) - x_{ref}(k|t)^T) Q(x(k|t) - x_{ref}(k|t)) +\\ & R \sum_{k=0}^{N_p-1} u(k|t)^2 + R_{\Delta \mu}\sum_{k=0}^{N_p-2} (u(k+1|t) - u(k|t))^2 \end{split} \end{equation*} where [math]\displaystyle{ k }[/math] and [math]\displaystyle{ t }[/math] are the prediction step index and time index, respectively; [math]\displaystyle{ x(k|t) }[/math] and [math]\displaystyle{ x_{ref} (k|t) }[/math] are the states and reference of the MPC problem, respectively; [math]\displaystyle{ x(k|t) }[/math] is composed of travel distance px and longitudinal velocity vx; [math]\displaystyle{ x_{ref} (k|t) }[/math] consists of reference travel distance [math]\displaystyle{ p_{x,ref} }[/math] and reference longitudinal velocity [math]\displaystyle{ v_{x,ref} }[/math] ; [math]\displaystyle{ u(k|t) }[/math] is the control input, which is the longitudinal acceleration command; [math]\displaystyle{ N_p }[/math] is the prediction horizon; and Q, R, and [math]\displaystyle{ R_{\Delta \mu} }[/math] are the weight matrices for states, input, and input derivative, respectively, and these weight matrices were tuned to obtain control inputs from the proposed controller that were as similar as possible to those of human-driven vehicles. The weight matrices were used for the controller, for the proposed and conventional motion predictors. The dynamic constraints for the controller were defined as follors $$\begin{bmatrix} p_{x}(k+1|t)\\ v_{x}(k+1|t)\end{bmatrix} = \begin{bmatrix}1 & dt \\0 &1-dt/\tau \end{bmatrix}\begin{bmatrix} p_{x}(k|t)\\ v_{x}(k|t)\end{bmatrix} + \begin{bmatrix} 0 & dt/\tau\end{bmatrix}u(k|t) $$ The constraints of the control input are defined as follows: \begin{equation*} \begin{split} &\mu_{min} \leq \mu(k|t) \leq \mu_{max} \\ &||\mu(k+1|t) - \mu(k|t)|| \leq S \end{split} \end{equation*} Where [math]\displaystyle{ u_{min} }[/math], [math]\displaystyle{ u_{max} }[/math]and S are the minimum/maximum control input and maximum slew rate of input respectively.
Determine the position and speed boundary based on the predicted state: \begin{equation*} \begin{split} & p_{x,max}(k|t) = p_{x,tar}(k|t) - c_{des}(k|t) \quad p_{x,min}(k|t) = 0 \\ & v_{x,max}(k|t) = min(v_{x,ret}(k|t), v_{x,limit}) \quad v_{x,min}(k|t) = 0 \end{split} \end{equation*} Where [math]\displaystyle{ v_{x, limit} }[/math] are the speed limits of the target vehicle.
Prediction performance analysis and application to motion planning
Accuracy analysis
The proposed algorithm was compared with the results from three base algorithms, a path-following model with constant velocity, a path-following model with traffic flow and a CTRV model.
We compare those algorithms according to four sorts of errors, The [math]\displaystyle{ x }[/math] position error [math]\displaystyle{ e_{x,T_p} }[/math], [math]\displaystyle{ y }[/math] position error [math]\displaystyle{ e_{y,T_p} }[/math], heading error [math]\displaystyle{ e_{\theta,T_p} }[/math], and velocity error [math]\displaystyle{ e_{v,T_p} }[/math] where [math]\displaystyle{ T_p }[/math] denotes time [math]\displaystyle{ p }[/math]. These four errors are defined as follows:
\begin{equation*} \begin{split} e_{x,Tp}=& p_{x,Tp} -\hat {p}_{x,Tp}\\ e_{y,Tp}=& p_{y,Tp} -\hat {p}_{y,Tp}\\ e_{\theta,Tp}=& \theta _{Tp} -\hat {\theta }_{Tp}\\ e_{v,Tp}=& v_{Tp} -\hat {v}_{Tp} \end{split} \end{equation*}
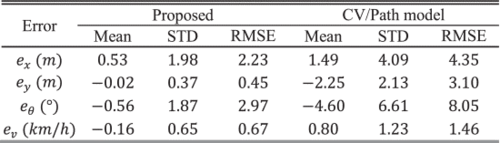
The proposed model shows significantly fewer prediction errors compare to the based algorithms in terms of mean, standard deviation(STD), and root mean square error(RMSE). Meanwhile, the proposed model exhibits a bell-shaped curve with a close to zero mean, which indicates that the proposed algorithm's prediction of human divers' intensions are relatively precise. On the other hand, [math]\displaystyle{ e_{x,T_p} }[/math], [math]\displaystyle{ e_{y,T_p} }[/math], [math]\displaystyle{ e_{v,T_p} }[/math] are bounded within reasonable levels. For instant, the three-sigma range of [math]\displaystyle{ e_{y,T_p} }[/math] is within the width of a lane. Therefore, the proposed algorithm can be precise and maintain safety simultaneously.
Motion planning application
Case study of a multi-lane left turn scenario
The proposed method mimics a human driver better, by simulating a human driver's decision-making process. In a multi-lane left turn scenario, the proposed algorithm correctly predicted the trajectory of a target vehicle, even when the target vehicle was not following the intersection guideline.
Statistical analysis of motion planning application results
The data is analyzed from two perspectives, the time to recognize the in-lane target and the similarity to human driver commands. In most of cases, the proposed algorithm detects the in-line target no late than based algorithm. In addition, the proposed algorithm only recognized cases later than the base algorithm did when the surrounding target vehicles first appeared beyond the sensors’ region of interest boundaries. This means that these cases took place sufficiently beyond the safety distance, and had little influence on determining the behaviour of the subject vehicle.
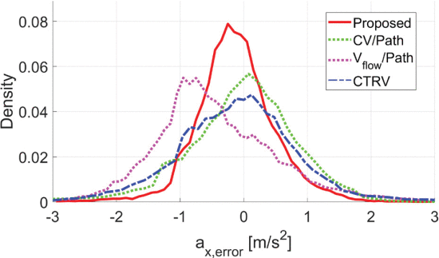
In order to compare the similarities between the results from the proposed algorithm and human driving decisions, this article introduced another type of error, acceleration error [math]\displaystyle{ a_{x, error} = a_{x, human} - a_{x, cmd} }[/math]. where [math]\displaystyle{ a_{x, human} }[/math] and [math]\displaystyle{ a_{x, cmd} }[/math] are the human drivers' acceleration history and the command from the proposed algorithm, respectively. The proposed algorithm showed more similar results to human drivers’ decisions than the base algorithm. [math]\displaystyle{ 91.97\% }[/math] of the acceleration error lies in the region [math]\displaystyle{ \pm 1 m/s^2 }[/math]. Moreover, the base algorithm possesses a limited ability to respond to different in-lane target behaviours in traffic flow. Hence, the proposed model is efficient and safe.
Conclusion
A surrounding vehicle motion predictor based on an LSTM-RNN at multi-lane turn intersections was developed and its application in an autonomous vehicle was evaluated. The model was trained by using the data captured on the urban road in Seoul in MPC. The evaluation results showed precise prediction accuracy and so the algorithm is safe to be applied on an autonomous vehicle. Also, the comparison with the other three base algorithms (CV/Path, V_flow/Path, and CTRV) revealed the superiority of the proposed algorithm. The evaluation results showed precise prediction accuracy. In addition, the time-to-recognize in-lane targets within the intersection improved significantly over the performance of the base algorithms. The proposed algorithm was compared with human driving data, and it showed similar longitudinal acceleration. The motion predictor can be applied to path planners when AVs travel in unconstructed environments, such as multi-lane turn intersections.
Future works
This paper has identified several venues for future research, which include:
1.Developing trajectory prediction algorithms using other machine learning algorithms, such as attention-aware neural networks.
2.Applying the machine learning-based approach to infer lane change intention at motorways and main roads of urban environments.
3.Extending the target road of the trajectory predictor, such as roundabouts or uncontrolled intersections, to infer yield intention.
4.Learning the behavior of surrounding vehicles in real time while automated vehicles drive with real traffic.
Critiques
The literature review is not sufficient. It should focus more on LSTM, RNN, and the study in different types of roads. Why the LSTM-RNN is used, and the background of the method is not stated clearly. There is a lack of concept so that it is difficult to distinguish between LSTM-RNN based motion predictor and motion planning.
This is an interesting topic to discuss. This is a major topic for some famous vehicle companies such as Tesla, which now already has a good service called Autopilot to give self-driving and Motion Prediction. This summary can include more diagrams in architecture in the model to give readers a whole view of how the model looks like. Since it is using LSTM-RNN, include some pictures of the LSTM-RNN will be great. I think it will be interesting to discuss more applications by using this method, such as Airplane, boats.
Autonomous driving is a very hot topic, and training the model with LSTM-RNN is also a meaningful topic to discuss. By the way, it would be an interesting approach to compare the performance of different algorithms or some other traditional motion planning algorithms like KF.
There are some papers that discussed the accuracy of different models in vehicle predictions, such as Deep Kinematic Models for Kinematically Feasible Vehicle Trajectory Predictions[1] The LSTM didn't show good performance. They increased the accuracy by combing LSTM with an unconstrained model(UM) by adding an additional LSTM layer of size 128 that is used to recursively output positions instead of simultaneously outputting positions for all horizons.
It may be better to provide the results of experiments to support the efficiency of LSTM-RNN, talk about the prediction of training and test sets, and compared it with other autonomous driving systems that exist in the world.
The topic of surround vehicle motion prediction is analogous to the topic of autonomous vehicles. An example of an application of these frameworks would be the transportation services industry. Many companies, such as Lyft and Uber, have started testing their own commercial autonomous vehicles.
It would be really helpful if some visualization or data summary can be provided to understand the content, such as the track of the car movement.
The model should have been tested in other regions besides just Seoul, as driving behaviors can vary drastically from region to region.
Understandably, a supervised learning problem should be evaluated on some test dataset. However, supervised learning techniques are inherently ill-suited for general planning problems. The test dataset was obtained from human driving data which is known to be extremely noisy as well as unpredictable when it comes to motion planning. It would be crucial to determine the successes of this paper based on the state-of-the-art reinforcement learning techniques.
It would be better if the authors compared their method against other SOTA methods. Also one of the reasons motion planning is done using interpretable methods rather than black boxes (such as this model) is because it is hard to see where things go wrong and fix problems with the black box when they occur - this is something the authors should have also discussed.
A future area of study is to combine other source of information such as signals from Lidar or car side cameras to make a better prediction model.
It might be interesting and helpful to conduct some training and testing under different weather/environmental conditions, as it could provide more generalization to real-life driving scenarios. For example, foggy weather and evening (low light) conditions might affect the performance of sensors, and rainy weather might require a longer braking distance.
This paper proposes an interesting, novel model prediction algorithm, using LSTM_RNN. However, since motion prediction in autonomous driving has great real-life impacts, I do believe that the evaluations of the algorithm should be more thorough. For example, more traditional motion planning algorithms such as multi-modal estimation and Kalman filters should be used as benchmarks. Moreover, the experiment results are based on Korean driving conditions only. Eastern and Western drivers can have very different driving patterns, so that should be addressed in the discussion section of the paper as well.
The paper mentions that in the future, this research plans to learn the real life behaviour of automated vehicles. Seeing a possible improvement in road safety due to this research will be very interesting.
This predictor is also possible to be applied in the traffic control system.
This prediction model should consider various conditions that could happen in an intersection. However, normal prediction may not work when there is a traffic jam or in some crowded time periods like rush hours.
It would be better that the author could provide more comparison between the LSTN-RNN algorithm and other traditional algorithm such as RNN or just LSTM.
The paper has really good results for what they aimed to achieve. However for the future work it would also be nice to have various climates/weathers to be included in the Seoul dataset. I think it's also important to consider it as different climates/weather (such as snowy roads, or rain) would introduce more noisier data (camera's image processing) and the human drivers behaviour would change as well to adapt to the new environment.
It would be good to have a future work section to discusses shortage of current algorithms and the possible improvement.
The summary explains the whole process well, but is missing the small details among the steps. It would be better to explain concepts such as RNN, modelling procedure for first time users.
This paper presents a nice method, but does not seem particularly well developed. I would have liked to see some more ablations on this particular choice of RNN, as there are more efficient variants such as GRU which show similar performance in other tasks while being more amenable to real-time inference. Furthermore, the multi-model aspect seems slightly ad-hoc, it would have been nice to see a more rigorous formulation similar to seen in some recent work by Zeng et al. from Uber ATG: https://arxiv.org/pdf/2008.06041.pdf.
The data used for this paper contains driver information exclusively to the urban roads of Gwanak-gu Seoul, hence the data may contain an inherited bias as drivers around the rest of the country, let alone the rest of the world, will have different habits based on different environments. It would be interesting to see if this model can be applied to other cities around the world and exhibit similar results or would there be a need to tune it based off geographic location.
Since the data is based on urban roads, It would be better to include the details on performance of the model on high traffic area vs low traffic urban area. It would also be interesting to see the performance of the model with many pedestrians.
While it would be nice to read more on why the authors chose LSTM-RNN, the paper exhibits a potential way to improve autonomous vehicle performance. It would be interesting to see how an army of robots would behave when this paper's method is applied in robotics, since robots' motions also follow a trajectory.
An interesting topic, but the paper and accompanying summary are missing some details that would improve understandability. With respect to the background of the topic, a more detailed explanation of trajectories in the case of driving would help to better motivate the research. The addition of benchmarks and comparisons to current industry standards (if they are published publicly) would help to contextualize the results of the LSTM-RNN. An area of further study is applying these techniques to different weather situations and driving patterns in different countries. How does this model perform in regions where driver's very loosely follow the laws of the road? Further, could the research be generalized for controlled and uncontrolled turn lanes, especially on roads with higher speed limits?
In the past, LSTM is a very popular model in natural language. It is interesting to see how it is used for motion prediction. Although the result is not good enough, it is still a good start. In the future, more language models can be used to see how well they can perform to do a comparison such as BiLSTM, Transformer, and Bert.
LSTM-RNNs are really incredibly useful for sequential learning. The RNN should be able to predict, not only when a vehicle in front of the motion sensors, but it's likelihood of staying in motion vs coming to a sudden stop. There is no details in this review on the running time of the algorithm. In a field where split seconds could be the difference between an accident and a brake, running time cannot be stressed enough. Whether or not it is truly capable of dealing with real time traffic cannot be judged without relevant data and results being shown.
The prediction could be more flexible and be trained and tested with more complex cases. In real-world, unpredicted situations could always happen, and usual predictions might not be aware of those cases.
We must notice that the model is not presenting the time data of training and prediction and adaptation to road conditions as it learns the history of conditions. This is an inherently flowed experiment as time is extremely important to how well a model is to predict and assess the movement of a moving vehicle. Even though a desired time gap is given, it is sufficient for a vehicle at moving speed. It does not give what does the model do as it realizes the prediction was wrong and the steps following after as sensors detect new information.
This is rather late, but I find there is an important concept missing in section 8 of the summary. In the original article, although not explicitly stated, the author compared the difference in recognition timing between two different predictors. It was revealed later that the proposed algorithm only recognized cases later than the base algorithm did when the surrounding target vehicles first appeared beyond the sensors’ region of interest boundaries, which was illustrated using a plot. Although it is not as important as other contents contained in the same section, the plot is still a good evidence to show how the proposed algorithm's performance can be affected by the target vehicles' motion.
Reference
[1] E. Choi, Crash Factors in Intersection-Related Crashes: An On-Scene Perspective (No. Dot HS 811 366), U.S. DOT Nat. Highway Traffic Safety Admin., Washington, DC, USA, 2010.
[2] D. J. Phillips, T. A. Wheeler, and M. J. Kochenderfer, “Generalizable intention prediction of human drivers at intersections,” in Proc. IEEE Intell. Veh. Symp. (IV), Los Angeles, CA, USA, 2017, pp. 1665–1670.
[3] B. Kim, C. M. Kang, J. Kim, S. H. Lee, C. C. Chung, and J. W. Choi, “Probabilistic vehicle trajectory prediction over occupancy grid map via recurrent neural network,” in Proc. IEEE 20th Int. Conf. Intell. Transp. Syst. (ITSC), Yokohama, Japan, 2017, pp. 399–404.
[4] E. Strigel, D. Meissner, F. Seeliger, B. Wilking, and K. Dietmayer, “The Ko-PER intersection laserscanner and video dataset,” in Proc. 17th Int. IEEE Conf. Intell. Transp. Syst. (ITSC), Qingdao, China, 2014, pp. 1900–1901.
[5] Henggang Cui, Thi Nguyen, Fang-Chieh Chou, Tsung-Han Lin, Jeff Schneider, David Bradley, Nemanja Djuric: “Deep Kinematic Models for Kinematically Feasible Vehicle Trajectory Predictions”, 2019; arXiv:1908.00219.
[6]Schulz, Jens & Hubmann, Constantin & Morin, Nikolai & Löchner, Julian & Burschka, Darius. (2019). Learning Interaction-Aware Probabilistic Driver Behavior Models from Urban Scenarios. 10.1109/IVS.2019.8814080.