Speech2Face: Learning the Face Behind a Voice: Difference between revisions
(85 intermediate revisions by 52 users not shown) | |||
Line 3: | Line 3: | ||
== Introduction == | == Introduction == | ||
This paper presents a deep neural network architecture called Speech2Face | This paper presents a deep neural network architecture called Speech2Face which utilizes millions of Internet/Youtube videos of people speaking to learn the correlation between a voice and the respective face. The model produces facial reconstruction images that capture specific physical attributes learning the correlations between faces and voices, such as a person's age, gender, or ethnicity, through a self-supervised procedure. Namely, the model utilizes the simultaneous occurrence of faces and speech in videos and does not need to model the attributes explicitly. This model explores what types of facial information could be extracted from speech without the constraints of predefined facial characterizations. Without any prior information or accurate classifiers, the reconstructions revealed correlations between craniofacial features and voice in addition to the correlation between dominant features (gender, age, ethnicity, etc.) and voice. The model is evaluated and numerically quantifies how closely the reconstruction, done by the Speech2Face model, resembles the true face images of the respective speakers. | ||
== Ethical Considerations == | |||
The authors note that due to the potential sensitivity of facial information, they have chosen to explicitly state some ethical considerations. The first of which is privacy. The paper states that the method cannot recover the true identity of the face or produce faces of specific individuals, but rather will show average-looking faces. The paper also addresses that there are potential dataset biases that exist for the voice-face correlations, thus the faces may not accurately represent the intended population. The paper recommends that any further investigation or practical use of this technology will be tested to represent the intended population and also if the data does not reflect this, more representative data should be broadly collected. Finally, it acknowledges that the model uses demographic categories such as "White" and "Asian" that are defined by a commercial face attribute classifier. | |||
== Previous Work == | == Previous Work == | ||
With visual and audio signals being so dominant and accessible in our daily life, there has been huge interest in how visual and audio perceptions interact with each other. Arandjelovic and Zisserman [1] leveraged the existing database of mp4 files to learn a generic audio representation to classify whether a video frame and an audio clip correspond to each other. These learned audio-visual representations have been used in a variety of setting, including cross-modal retrieval, sound source localization and sound source separation. This also paved the path for specifically studying the association between faces and voices of agents in the field of computer vision. In particular, cross-modal signals extracted from faces and voices have been proposed as a binary or multi-task classification task and there have been some promising results. Studies have been able to identify active speakers of a video, | With visual and audio signals being so dominant and accessible in our daily life, there has been huge interest in how visual and audio perceptions interact with each other. Arandjelovic and Zisserman [1] leveraged the existing database of mp4 files to learn a generic audio representation to classify whether a video frame and an audio clip correspond to each other. These learned audio-visual representations have been used in a variety of setting, including cross-modal retrieval, sound source localization and sound source separation. This also paved the path for specifically studying the association between faces and voices of agents in the field of computer vision. In particular, cross-modal signals extracted from faces and voices have been proposed as a binary or multi-task classification task and there have been some promising results. Studies have been able to identify active speakers of a video, separate speech from multiple concurrent sources, predict lip motion from speech, and even learn the emotion of the agents based on their voices. Aytar et al. [6] proposed a student-teacher training procedure in which a well established visual recognition model was used to transfer the knowledge obtained in the visual modality to the sound modality, using unlabeled videos. | ||
Recently, various methods have been suggested to use various audio signals to reconstruct visual information, where the reconstructed subject is subjected to a priori. Notably, Duarte et al. [2] were able to synthesize the exact face images and expression of an agent from speech using a GAN model. This paper instead hopes to recover the dominant and generic facial structure from a speech. | Recently, various methods have been suggested to use various audio signals to reconstruct visual information, where the reconstructed subject is subjected to a priori. Notably, Duarte et al. [2] were able to synthesize the exact face images and expression of an agent from speech using a GAN model. A generative adversarial network (GAN) model is one that uses a generator to produce seemingly possible data for training and a discriminator that identifies if the training data is fabricated by the generator or if it is real [7]. This paper instead hopes to recover the dominant and generic facial structure from a speech. | ||
== Motivation == | == Motivation == | ||
It seems to be a common trait among humans to imagine what some people look like when we hear their voices before we see what they look like. There is a strong connection between speech and appearance, which is a direct result of the factors that affect speech, including age, gender, and facial bone structure. In addition, other voice-appearance correlations stem from the way we talk: language, accent, speed, pronunciations, etc. These properties of speech are often common among many different nationalities and cultures, which can, in turn, translate to common physical features among different voices. Namely, from an input audio segment of a person speaking, the method would reconstruct an image of the person’s face in a canonical form (frontal-facing, neutral expression). The goal was to study to what extent people can infer how someone else looks from the way they talk. Rather than predicting a recognizable image of the exact face, the authors are more interested in capturing the dominant facial features. | |||
== Model Architecture == | == Model Architecture == | ||
Line 17: | Line 21: | ||
'''Speech2Face model and training pipeline''' | '''Speech2Face model and training pipeline''' | ||
[[File:ModelFramework.jpg]] | [[File:ModelFramework.jpg|center]] | ||
<div style="text-align:center;"> Figure 1. '''Speech2Face model and training pipeline''' </div> | |||
The Speech2Face Model consists of two parts - a voice encoder which takes in a spectrogram of speech as input and outputs low dimensional face features, and a face decoder which takes in face features as input and outputs a normalized image of a face (neutral expression, looking forward). Figure 1 gives a visual representation of the pipeline of the entire model, from video input to a recognizable face. The combination of the voice encoder and face decoder results are combined to form an image. The variability in facial expressions, head positions and lighting conditions of the face images creates a challenge to both the design and training of the Speech2Face model. It needs a model to figure out many irrelevant variations in the data, and to implicitly extract important internal representations of faces. To avoid this problem the model is trained to first regress to a low dimensional intermediate representation of the face. | |||
'''Face Decoder''' | |||
The face decoder itself was taken from previous work The VGG-Face model by Cole et al [3] (a face recognition model that is pretrained on a largescale face database [5] is used to extract a 4069-D face feature from the penultimate layer of the network.) and will not be explored in great detail here, but in essence the facenet model is combined with a single multilayer perceptron layer, the result of which is passed through a convolutional neural network to determine the texture of the image, and a multilayer perception to determine the landmark locations. The face decoder kept the VGG-Face model's dimension and weights. The weights were also trained separately and remained fixed during the voice encoder training. | |||
'''Voice Encoder Architecture''' | '''Voice Encoder Architecture''' | ||
[[File:VoiceEncoderArch.JPG]] | [[File:VoiceEncoderArch.JPG|center]] | ||
Table 1: '''Voice encoder architecture''' | <div style="text-align:center;"> Table 1: '''Voice encoder architecture''' </div> | ||
The voice encoder itself is a convolutional neural network, which transforms the input spectrogram into pseudo face features. The exact architecture is given in Table 1. The model alternates between convolution, ReLU, batch normalization layers, and layers of max-pooling. In each max-pooling layer, pooling is only done along the temporal dimension of the data. This is to ensure that the frequency, an important factor in determining vocal characteristics such as tone, is preserved. In the final pooling layer, an average pooling is applied along the temporal dimension. This allows the model to aggregate information over time | The voice encoder itself is a convolutional neural network, which transforms the input spectrogram into pseudo face features. The exact architecture is given in Table 1. The model alternates between convolution, ReLU, batch normalization layers, and layers of max-pooling. In each max-pooling layer, pooling is only done along the temporal dimension of the data. This is to ensure that the frequency, an important factor in determining vocal characteristics such as tone, is preserved. In the final pooling layer, an average pooling is applied along the temporal dimension. This allows the model to aggregate information over time and allows the model to be used for input speeches of varying lengths. Two fully connected layers at the end are used to return a 4096-dimensional facial feature output. | ||
'''Training''' | '''Training''' | ||
The | The AVSpeech dataset, a large-scale audio-visual dataset is used for the training. AVSpeech dataset is comprised of millions of video segments from Youtube with over 100,000 different people. The training data is composed of educational videos and does not provide an accurate representation of the global population, which will clearly affect the model. Also note that facial features that are irrelevant to speech, like hair color, may be predicted by the model. From each video, a 224x224 pixels image of the face was passed through the face decoder to compute a facial feature vector. Combined with a spectrogram of the audio, a training and test set of 1.7 and 0.15 million entries respectively were constructed. | ||
The voice encoder is trained in a self-supervised manner. A frame | The voice encoder is trained in a self-supervised manner. A frame that contains the face is extracted from each video and then inputted to the VGG-Face model to extract the feature vector <math>v_f</math>, the 4096-dimensional facial feature vector given by the face decoder on a single frame from the input video. This provides the supervision signal for the voice-encoder. The feature <math>v_s</math>, the 4096 dimensional facial feature vector from the voice encoder, is trained to predict <math>v_f</math>. | ||
In order to train this model, a proper loss function must be defined. The L1 norm of the difference between <math>v_s</math> and <math>v_f</math>, given by <math>||v_f - v_s||_1</math>, may seem like a suitable loss function, but in actuality results in unstable results and long training times. Figure 2, below, shows the difference in predicted facial features given by <math>||v_f - v_s||_1</math> and the following loss. Based on the work of Castrejon et al. [4], a loss function is used which penalizes the differences in the last layer of the | In order to train this model, a proper loss function must be defined. The L1 norm of the difference between <math>v_s</math> and <math>v_f</math>, given by <math>||v_f - v_s||_1</math>, may seem like a suitable loss function, but in actuality results in unstable results and long training times. Figure 2, below, shows the difference in predicted facial features given by <math>||v_f - v_s||_1</math> and the following loss. Based on the work of Castrejon et al. [4], a loss function is used which penalizes the differences in the last layer of the VGG-Face model <math>f_{VGG}</math>: <math> \mathbb{R}^{4096} \to \mathbb{R}^{2622}</math> and the first layer of face decoder <math>f_{dec}</math> : <math> \mathbb{R}^{4096} \to \mathbb{R}^{1000}</math>. The final loss function is given by: $$L_{total} = ||f_{dec}(v_f) - f_{dec}(v_s)|| + \lambda_1||\frac{v_f}{||v_f||} - \frac{v_s}{||v_s||}||^2_2 + \lambda_2 L_{distill}(f_{VGG}(v_f), f_{VGG}(v_s))$$ | ||
This loss penalizes on both the normalized Euclidean distance between the 2 facial feature vectors and the knowledge distillation loss, which is given by: $$L_{distill}(a,b) = -\sum_ip_{(i)} | This loss penalizes on both the normalized Euclidean distance between the 2 facial feature vectors and the knowledge distillation loss, which is given by: $$L_{distill}(a,b) = -\sum_ip_{(i)}(a)\text{log}p_{(i)}(b)$$ $$p_{(i)}(a) = \frac{\text{exp}(a_i/T)}{\sum_j \text{exp}(a_j/T)}$$ Knowledge distillation is used as an alternative to Cross-Entropy. By recommendation of Cole et al [3], <math> T = 2 </math> was used to ensure a smooth activation. <math>\lambda_1 = 0.025</math> and <math>\lambda_2 = 200</math> were chosen so that magnitude of the gradient of each term with respect to <math>v_s</math> are of similar scale at the <math>1000^{th}</math> iteration. | ||
<center> | <center> | ||
Line 48: | Line 55: | ||
</center> | </center> | ||
Figure 2: '''Qualitative results on the AVSpeech test set''' | <div style="text-align:center;"> Figure 2: '''Qualitative results on the AVSpeech test set''' </div> | ||
'''Implementation Details''' | |||
6 seconds of audio was used to compute the spectogram by taking a Short-time Fourier transform with Hann window of 25mm, hop length of 10ms, and 512 FFT frequenct bands. A CNN-based face detector from Dlib was used to crop the face regions from the frames. The VGG-face features are computed from the resized faces and together with the spectrogram was used for training. There were a total of 1.7 and 0.15 million spectra-feature pairs. | |||
== Results == | == Results == | ||
Line 57: | Line 68: | ||
[[File:Confusionmatrix.png| 600px]] | [[File:Confusionmatrix.png| 600px]] | ||
</center> | </center> | ||
<div style="text-align:center;"> Figure 3. '''Facial attribute evaluation''' </div> | |||
In order to determine the similarity between the generated images and the ground truth, a commercial service known as Face++ which classifies faces for distinct attributes (such as gender, ethnicity, etc) was used. Figure 3 gives a confusion matrix based on gender, ethnicity, and age. By examining these matrices, it is seen that the Speech2Face model performs very well on gender, only misclassifying 6% of the time. Similarly, the model performs fairly well on ethnicities, especially with white or Asian faces. Although the model performs worse on black and Indian faces, that can be attributed to the vastly unbalanced data, where 50% of the data represented a white face, and 80% represented a white or Asian face. | In order to determine the similarity between the generated images and the ground truth, a commercial service known as Face++ which classifies faces for distinct attributes (such as gender, ethnicity, etc) was used. Figure 3 gives a confusion matrix based on gender, ethnicity, and age. By examining these matrices, it is seen that the Speech2Face model performs very well on gender, only misclassifying 6% of the time. Similarly, the model performs fairly well on ethnicities, especially with white or Asian faces. Although the model performs worse on black and Indian faces, that can be attributed to the vastly unbalanced data, where 50% of the data represented a white face, and 80% represented a white or Asian face. | ||
Line 66: | Line 81: | ||
</center> | </center> | ||
<div style="text-align:center;"> Table 2. '''Feature similarity''' </div> | |||
Another examination of the result is the similarity of features predicted by the Speech2Face model. The cosine, L1, and L2 distance between the facial feature vector produced by the model and true facial feature vector from the face decoder were computed, and presented above | Another examination of the result is the similarity of features predicted by the Speech2Face model. The cosine, L1, and L2 distance between the facial feature vector produced by the model and the true facial feature vector from the face decoder were computed, and presented, above, in Table 2. A comparison of facial similarity was also done based on the length of audio input. From the table, it is evident that the 6-second audio produced a lower cosine, L1, and L2 distance, resulting in a facial feature vector that is closer to the ground truth. | ||
''' | '''S2F -> Face retrieval performance''' | ||
<center> | <center> | ||
Line 76: | Line 93: | ||
</center> | </center> | ||
The performance of the model was also examined on how well it could produce the original image. The R@K metric, also known as retrieval performance by recall at K, | <div style="text-align:center;"> Table 3. '''S2F -> Face retrieval performance''' </div> | ||
The performance of the model was also examined on how well it could produce the original image. The R@K metric, also known as retrieval performance by recall at K, measures the probability that the K closest images to the model output includes the correct image of the speaker's face. A higher R@K score indicates better performance. From Table 3, above, we see that both the 3-second and 6-second audio showed significant improvement over random chance, with the 6-second audio performing slightly better. | |||
'''Additional Observations''' | |||
Ablation studies were carried out to test the effect of audio duration and batch normalization. It was found that the duration of input audio during the training stage had little effect on convergence speed (comparing 3 and 6-second speech segments), while in the test stage longer input speech yields improvement in reconstruction quality. With respect to batch normalization (BN), it was found that without BN reconstructed faces would converge to an average face, while the inclusion of BN led to results which contained much richer facial features. | |||
== Conclusion == | == Conclusion == | ||
The report presented a novel study of face reconstruction from audio recordings of a person speaking. The model was demonstrated to be able to predict plausible face reconstructions with similar facial features to real images of the person speaking. The problem was addressed by learning to align the feature space of speech to that of a pretrained face decoder. The model was trained on millions of videos of people speaking from YouTube. The model was then evaluated by comparing the reconstructed faces with a commercial facial detection service. The authors believe that facial reconstruction allows a more comprehensive view of voice-face correlation compared to predicting individual features, which may lead to new research opportunities and applications. | The report presented a novel study of face reconstruction from audio recordings of a person speaking. The model was demonstrated to be able to predict plausible face reconstructions with similar facial features to real images of the person speaking. We have proved that our method can predict the attributes that the real face of the face is consistent with the real image. By directly reconstructing the face from this cross-modal feature space, we visually verify the existence of cross-mode biometrics. The problem was addressed by learning to align the feature space of speech to that of a pretrained face decoder. The model was trained on millions of videos of people speaking from YouTube. The model was then evaluated by comparing the reconstructed faces with a commercial facial detection service. The authors believe that facial reconstruction allows a more comprehensive view of voice-face correlation compared to predicting individual features, which may lead to new research opportunities and applications. | ||
== Discussion and Critiques == | == Discussion and Critiques == | ||
There is evidence that the results of the model may be heavily influenced by external factors: | |||
1. Their method of sampling random YouTube videos resulted in an unbalanced sample in terms of ethnicity. Over half of the samples were white. We also saw a large bias in the model's prediction of ethnicity towards white. The bias in the results shows that the model may be overfitting the training data and puts into question what the performance of the model would be when trained and tested on a balanced dataset. Figure (11) highlights this shortcoming: The same man heard speaking in either English or Chinese was predicted to have a "white" appearance or an "asian" appearance respectively. | |||
2. The model was shown to infer different face features based on language. This puts into question how heavily the model depends on the spoken language. The paper mentioned the quality of face reconstruction may be affected by uncommon languages, where English is the most popular language on Youtube(training set). Testing a more controlled sample where all speech recording was of the same language may help address this concern to determine the model's reliance on spoken language. | |||
3. The evaluation of the result is also highly dependent on the Face++ classifiers. Since they compare the age, gender, and ethnicity by running the Face++ classifiers on the original images and the reconstructions to evaluate their model, the model that they create can only be as good as the one they are using to evaluate it. Therefore, any limitations of the Face++ classifier may become a limitation of Speech2Face and may result in a compounding effect on the miss-classification rate. | |||
4. Figure 4.b shows the AVSpeech dataset statistics. However, it doesn't show the statistics about speakers' ethnicity and the language of the video. If we train the model with a more comprehensive dataset that includes enough Asian/Indian English speakers and native language speakers will this increase the accuracy? | |||
5. One concern about the source of the training data, i.e. the Youtube videos, is that resolution varies a lot since the videos are randomly selected. That may be the reason why the proposed model performs badly on some certain features. For example, it is hard to tell the age when the resolution is bad because the wrinkles on the face are neglected. | |||
6. The topic of this project is very interesting, but I highly doubt this model will be practical in real-world problems. Because there are many factors to affect a person's sound in a real-world environment. Sounds such as phone clock, TV, car horn and so on. These sounds will decrease the accuracy of the predicted result of the model. | |||
7. A lot of information can be obtained from someone's voice, this can potentially be useful for detective work and crime scene investigation. In our world of increasing surveillance, public voice recording is quite common and we can reconstruct images of potential suspects based on their voice. In order for this to be achieved, the model has to be thoroughly trained and tested to avoid false positives as it could have a highly destructive outcome for a falsely convicted suspect. | |||
8. This is a very interesting topic, and this summary has a good structure for readers. Since this model uses Youtube to train model, but I think one problem is that most of the YouTubers are adult, and many additional reasons make this dataset highly unbalanced. What is more, some people may have a baby voice, this also could affect the performance of the model. But overall, this is a meaningful topic, it might help police to locate the suspects. So it might be interesting to apply this to the police. | |||
9. In addition, it seems very unlikely that any results coming from this model would ever be held in regard even remotely close to being admissible in court to identify a person of interest until the results are improved and the model can be shown to work in real-world applications. Otherwise, there seems to be very little use for such technology and it could have negative impacts on people if they were to be depicted in an unflattering way by the model based on their voice. | |||
10. Using voice as a factor of constructing the face is a good idea, but it seems like the data they have will have lots of noise and bias. The voice of a video might not come from the person in the video. There are so many YouTubers adjusting their voices before uploading their video and it's really hard to know whether they adjust their voice. Also, most YouTubers are adults so the model cannot have enough training samples about teenagers and kids. | |||
11. It would be interesting to see how the performance changes with different face encoding sizes (instead of just 4096-D) and also difference face models (encoder/decoders) to see if better performance can be achieved. Also given that the dataset used was unbalanced, was the dataset used to train the face model the same dataset? or was a different dataset used (the model was pretrained). This could affect the performance of the model as well. | |||
12. The audio input is transformed into a spectrogram before being used for training. They use STFT with a Hann window of 25 mm, a hop length of 10 ms, and 512 FFT frequency bands. They cite this method from a paper that focuses on speech separation, not speech classification. So, it would be interesting to see if there is a better way to do STFT, possibly with different hyperparameters (eg. different windowing, different number of bands), or if another type of transform (eg. wavelet transform) would have better results. | |||
13. A easy way to get somewhat balanced data is to duplicate the data that are fewer. | |||
14. This problem is interesting but is hard to generalize. This algorithm didn't account for other genders and mixed-race. In addition, the face recognition software Face++ introduces bias which can carry forward to Speech2Face algorithm. Face recognition algorithms are known to have higher error rates classifying darker-skinned individuals. Thus, it'll be tough to apply it to real-life scenarios like identifying suspects. | |||
15. This experiment raises a lot of ethical complications when it comes to possible applications in the real world. Even if this model was highly accurate, the implications of being able to discern a person's racial ethnicity, skin tone, etc. based solely on there voice could play in to inherent biases in the application user and this may end up being an issue that needs to be combatted in future research in this area. Another possible issue is that many people will change their intonation or vocal features based on the context (I'll likely have a different voice pattern in a job interview in terms of projection, intonation, etc. than if I was casually chatting/mumbling with a friend while playing video games for example). | |||
16. Overall a very interesting topic. I want to talk about the technical challenged raised by using the AVSSpeech dataset for training. The paper acknowledges that the AVSSpeech is unbalanced, and 80% of the data are white and Asians. It also says in the results section that "Our model does not perform on other races due to the imbalance in data". There does not seem to be any effort made in balancing the data. I think that there are definitely some data processing techniques that can be used (filtering, data augmentation, etc) to address the class imbalance problem. Not seeing any of these in the paper is a bit disappointing. Another issue I have noticed is that the model aims to predict an average-looking face from certain gender/racial group from voice input, due to ethical considerations. If we cannot reveal the identify of a person, why don't we predict the gender and race directly? Giving an average-looking face does not seem to be the most helpful. | |||
17. Very interesting research paper to be studied and the main objective was also interesting. This research leads to open question which can be applied to another application such as predicting person's face using voice and can be used in more advanced way. The only risk is how the data is obtained from YouTube where data is not consistent. | |||
18. The essay uses millions of natural videos of people speaking to find the correlation between face and voice. Since face and voice are commonly used as the identity of a person, there are many possible research opportunities and applications about improving voice and face unlock. | |||
19. It would be better to have a future work section to discuss the current shortage and explore the possible improvement and applications in the future. | |||
20. While the idea behind Speech2Face is interesting, ethnic profiling is a huge concern and it can further lead to racial discrimination, racism etc. Developers must put more care and thought into applying Speech2Face in tech before deploying the products. | |||
21. It would be helpful if the author could explore the different applications of this project in real life. Speech2face can be helpful during criminal investigation and essentially in scenarios when someone's picture is missing and only voice is available. It would also be helpful if the author could state the importance and need of such kind project in the society. | |||
22. The authors mention that they use the AVSpeech dataset for both training and testing but do not talk about how they split the data. It is possible that the same speakers were used in the training and testing data and so the model is able to recreate a face simply by matching the observed face to the observed audio. This would explain the striking example images shown in the paper. | |||
23. Another interesting application of this research is automated speech or facial animation at scale or in multiple languages. The cutting-edge automated facial animation solution provided by JALI Research Inc is applied in Cyberpunk 2077. | |||
24. It would be interesting to know the model can predict a similar face when one is speaking different languages. A person who is speaking multiple languages can have different tones and accents depending on a language that they speak. | |||
25. The results are actually amazing for the introduction of Speech2Face. As others have mentioned, the researchers might have used a biased dataset of YouTube videos favoring certain ethnicities and their accents and dialects. Thus, it would be nice to also see the data distribution. Additionally it would be nice to see how their model reacts to people who are able to speak multiple languages and see how well Speech2Face generalizes different language pronunciations of one person. | |||
26. The paper introduces Speech2Face and it definitely is one of the major areas of researches in the future. In the paper, the confusion matrix indicates that the model tends to misclassify based on the age of the speaking person. Specifically, the model tends to misclassify between 40-70. It would be interesting to see if the model could improve on its bottleneck by training on more speeches by the age group 40-70. | |||
27. An interesting topic, and as others have mentioned, has many ethical considerations and implications. Particularly in regions where call-recording is permitted, there is dangerous potential to for the technology to be misused to identify and target individuals. It would also be interesting to get a more in depth exploration into how the language spoken and accents have a bias. For example, if a person speaks with a strong British accent, are they classified as white? Particularly for Spanish-speakers, they vary greatly with respect to their skin colour and features, how well does the algorithm work on these individuals. A last nit-pick is the labelling used (i.e. Asian, White, Indian, Black) as this is not accurate since Indians, and moreover South Asians, fall under Asian as well. | |||
28. This topic is quite interesting and it could have great contribution in terms of criminal fight. But as the result, the accuracy is essential. There is still the space for much improvements since to tell a person's face by his/her voice is pretty hard since there are many factors such as oral structure, the language environment and even personality. Great bias could be resulted from these unpredictable factors. | |||
29. This is an interesting topic and could have great use in terms of finding criminals or people when having their voice recorded. However, the voice recording might be noisy and some might include voices of multiple people. It could consider ways to eliminate those factors that might effect the accuracy of the face generation. | |||
30. Most contents described in the paper are very useful. However, YouTube might not be a good enough data source since there are fewer labels to classify. Perhaps, after generating the model, the transfer learning could be done based on Facebook's videos in order to solve the imbalanced problem. | |||
31. This topic is really incredibly interesting and the writers should commend themselves on a job well done. However, Youtube, not only is it an ethnically skewed dataset, but has a non-negligible number of creators who use voice modifiers, auto tune, or a number of other things to change the pitch of their voices, which may lead to the significantly more errors in practical applications. A better dataset to be used could be Skype video calls, or a class room study. Also, judging from the way the model does it's prediction, it seems very prone to overfitting on the dataset, and will not generalize well, since pitch and sound are both incredibly variable across humans. | |||
32. One thing to notice is that the training data used to train the model is downloaded from Youtube, which may be a good site to retrieve a large amount of data. While it allows the possibility that the voices retrieved does not match with the people who made those sounds, claimed by the video. If that is the case, those records will become dirty data, and needs to be cleaned before training the model. Otherwise, there will be some huge misclassifications because some of the training data is not making sense. One way I can think of to improve this problem is that we may train multiple models on different subsets of the original dataset, and combine the results of all the models by taking weighted average. | |||
33. Predicting appearance with sound is a very imaginative research direction. But the author did not explain how to exclude environmental factors in data preprocessing, such as light intensity, facial dress, facial wounds, etc. In the training data set, different sound and image resolutions also affect the effectiveness of the model. The author needs more robustness tests to exclude these factors. | |||
34. The origin of data is primarily Western sourced. Even if taken into account the other ethnicities being selected, the resolution is decompression/compression quality is not maintained for an uploaded platform such as YouTube. Furthermore, since ethnicities are a factor in the model, people not speaking in their mother tongue might provide a very difficult sample for them to match since people tend to sound a certain way (volume, indentation, confidence, tone etc) which can all affect the quality of a recording. | |||
35. With this model's predictions, can it be used by law enforcement to make preemptive identification (with facial recognition) to capture/identify criminals (on surveillance mission)? | |||
36. Interesting article. I wonder how it will result if a participant speaks with a higher/lower pitch, or tries to imitate someone else intentionally. | |||
37. The result section can be expanded a lot more, the original paper studied something really interesting. For example, Craniofacial features were utilized to capture ratios and distances in the face, and how to regenerate a cartoon version of a particular face base on existing face images etc. | |||
== References == | == References == | ||
Line 99: | Line 198: | ||
[5] O. M. Parkhi, A. Vedaldi, and A. Zisserman. Deep face recognition. In British Machine Vision Conference (BMVC), 2015. | [5] O. M. Parkhi, A. Vedaldi, and A. Zisserman. Deep face recognition. In British Machine Vision Conference (BMVC), 2015. | ||
[6] “Overview of GAN Structure | Generative Adversarial Networks,” ''Google Developers'', 2019. [Online]. Available: https://developers.google.com/machine-learning/gan/gan_structure. [Accessed: 02-Dec-2020]. |
Latest revision as of 02:36, 15 December 2020
Presented by
Ian Cheung, Russell Parco, Scholar Sun, Jacky Yao, Daniel Zhang
Introduction
This paper presents a deep neural network architecture called Speech2Face which utilizes millions of Internet/Youtube videos of people speaking to learn the correlation between a voice and the respective face. The model produces facial reconstruction images that capture specific physical attributes learning the correlations between faces and voices, such as a person's age, gender, or ethnicity, through a self-supervised procedure. Namely, the model utilizes the simultaneous occurrence of faces and speech in videos and does not need to model the attributes explicitly. This model explores what types of facial information could be extracted from speech without the constraints of predefined facial characterizations. Without any prior information or accurate classifiers, the reconstructions revealed correlations between craniofacial features and voice in addition to the correlation between dominant features (gender, age, ethnicity, etc.) and voice. The model is evaluated and numerically quantifies how closely the reconstruction, done by the Speech2Face model, resembles the true face images of the respective speakers.
Ethical Considerations
The authors note that due to the potential sensitivity of facial information, they have chosen to explicitly state some ethical considerations. The first of which is privacy. The paper states that the method cannot recover the true identity of the face or produce faces of specific individuals, but rather will show average-looking faces. The paper also addresses that there are potential dataset biases that exist for the voice-face correlations, thus the faces may not accurately represent the intended population. The paper recommends that any further investigation or practical use of this technology will be tested to represent the intended population and also if the data does not reflect this, more representative data should be broadly collected. Finally, it acknowledges that the model uses demographic categories such as "White" and "Asian" that are defined by a commercial face attribute classifier.
Previous Work
With visual and audio signals being so dominant and accessible in our daily life, there has been huge interest in how visual and audio perceptions interact with each other. Arandjelovic and Zisserman [1] leveraged the existing database of mp4 files to learn a generic audio representation to classify whether a video frame and an audio clip correspond to each other. These learned audio-visual representations have been used in a variety of setting, including cross-modal retrieval, sound source localization and sound source separation. This also paved the path for specifically studying the association between faces and voices of agents in the field of computer vision. In particular, cross-modal signals extracted from faces and voices have been proposed as a binary or multi-task classification task and there have been some promising results. Studies have been able to identify active speakers of a video, separate speech from multiple concurrent sources, predict lip motion from speech, and even learn the emotion of the agents based on their voices. Aytar et al. [6] proposed a student-teacher training procedure in which a well established visual recognition model was used to transfer the knowledge obtained in the visual modality to the sound modality, using unlabeled videos.
Recently, various methods have been suggested to use various audio signals to reconstruct visual information, where the reconstructed subject is subjected to a priori. Notably, Duarte et al. [2] were able to synthesize the exact face images and expression of an agent from speech using a GAN model. A generative adversarial network (GAN) model is one that uses a generator to produce seemingly possible data for training and a discriminator that identifies if the training data is fabricated by the generator or if it is real [7]. This paper instead hopes to recover the dominant and generic facial structure from a speech.
Motivation
It seems to be a common trait among humans to imagine what some people look like when we hear their voices before we see what they look like. There is a strong connection between speech and appearance, which is a direct result of the factors that affect speech, including age, gender, and facial bone structure. In addition, other voice-appearance correlations stem from the way we talk: language, accent, speed, pronunciations, etc. These properties of speech are often common among many different nationalities and cultures, which can, in turn, translate to common physical features among different voices. Namely, from an input audio segment of a person speaking, the method would reconstruct an image of the person’s face in a canonical form (frontal-facing, neutral expression). The goal was to study to what extent people can infer how someone else looks from the way they talk. Rather than predicting a recognizable image of the exact face, the authors are more interested in capturing the dominant facial features.
Model Architecture
Speech2Face model and training pipeline
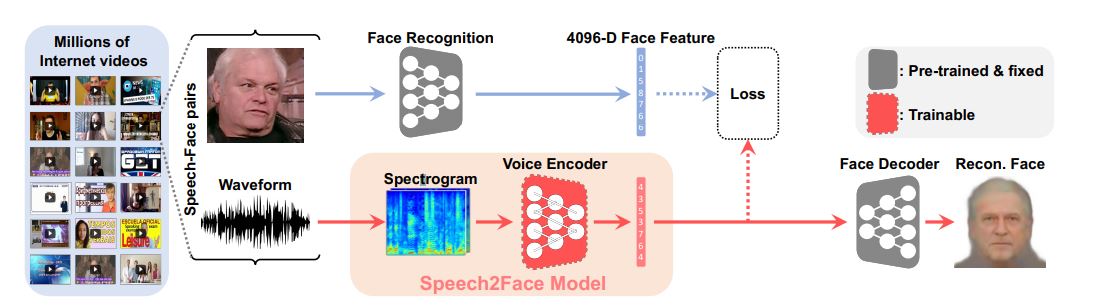
The Speech2Face Model consists of two parts - a voice encoder which takes in a spectrogram of speech as input and outputs low dimensional face features, and a face decoder which takes in face features as input and outputs a normalized image of a face (neutral expression, looking forward). Figure 1 gives a visual representation of the pipeline of the entire model, from video input to a recognizable face. The combination of the voice encoder and face decoder results are combined to form an image. The variability in facial expressions, head positions and lighting conditions of the face images creates a challenge to both the design and training of the Speech2Face model. It needs a model to figure out many irrelevant variations in the data, and to implicitly extract important internal representations of faces. To avoid this problem the model is trained to first regress to a low dimensional intermediate representation of the face.
Face Decoder The face decoder itself was taken from previous work The VGG-Face model by Cole et al [3] (a face recognition model that is pretrained on a largescale face database [5] is used to extract a 4069-D face feature from the penultimate layer of the network.) and will not be explored in great detail here, but in essence the facenet model is combined with a single multilayer perceptron layer, the result of which is passed through a convolutional neural network to determine the texture of the image, and a multilayer perception to determine the landmark locations. The face decoder kept the VGG-Face model's dimension and weights. The weights were also trained separately and remained fixed during the voice encoder training.
Voice Encoder Architecture
The voice encoder itself is a convolutional neural network, which transforms the input spectrogram into pseudo face features. The exact architecture is given in Table 1. The model alternates between convolution, ReLU, batch normalization layers, and layers of max-pooling. In each max-pooling layer, pooling is only done along the temporal dimension of the data. This is to ensure that the frequency, an important factor in determining vocal characteristics such as tone, is preserved. In the final pooling layer, an average pooling is applied along the temporal dimension. This allows the model to aggregate information over time and allows the model to be used for input speeches of varying lengths. Two fully connected layers at the end are used to return a 4096-dimensional facial feature output.
Training
The AVSpeech dataset, a large-scale audio-visual dataset is used for the training. AVSpeech dataset is comprised of millions of video segments from Youtube with over 100,000 different people. The training data is composed of educational videos and does not provide an accurate representation of the global population, which will clearly affect the model. Also note that facial features that are irrelevant to speech, like hair color, may be predicted by the model. From each video, a 224x224 pixels image of the face was passed through the face decoder to compute a facial feature vector. Combined with a spectrogram of the audio, a training and test set of 1.7 and 0.15 million entries respectively were constructed.
The voice encoder is trained in a self-supervised manner. A frame that contains the face is extracted from each video and then inputted to the VGG-Face model to extract the feature vector [math]\displaystyle{ v_f }[/math], the 4096-dimensional facial feature vector given by the face decoder on a single frame from the input video. This provides the supervision signal for the voice-encoder. The feature [math]\displaystyle{ v_s }[/math], the 4096 dimensional facial feature vector from the voice encoder, is trained to predict [math]\displaystyle{ v_f }[/math].
In order to train this model, a proper loss function must be defined. The L1 norm of the difference between [math]\displaystyle{ v_s }[/math] and [math]\displaystyle{ v_f }[/math], given by [math]\displaystyle{ ||v_f - v_s||_1 }[/math], may seem like a suitable loss function, but in actuality results in unstable results and long training times. Figure 2, below, shows the difference in predicted facial features given by [math]\displaystyle{ ||v_f - v_s||_1 }[/math] and the following loss. Based on the work of Castrejon et al. [4], a loss function is used which penalizes the differences in the last layer of the VGG-Face model [math]\displaystyle{ f_{VGG} }[/math]: [math]\displaystyle{ \mathbb{R}^{4096} \to \mathbb{R}^{2622} }[/math] and the first layer of face decoder [math]\displaystyle{ f_{dec} }[/math] : [math]\displaystyle{ \mathbb{R}^{4096} \to \mathbb{R}^{1000} }[/math]. The final loss function is given by: $$L_{total} = ||f_{dec}(v_f) - f_{dec}(v_s)|| + \lambda_1||\frac{v_f}{||v_f||} - \frac{v_s}{||v_s||}||^2_2 + \lambda_2 L_{distill}(f_{VGG}(v_f), f_{VGG}(v_s))$$ This loss penalizes on both the normalized Euclidean distance between the 2 facial feature vectors and the knowledge distillation loss, which is given by: $$L_{distill}(a,b) = -\sum_ip_{(i)}(a)\text{log}p_{(i)}(b)$$ $$p_{(i)}(a) = \frac{\text{exp}(a_i/T)}{\sum_j \text{exp}(a_j/T)}$$ Knowledge distillation is used as an alternative to Cross-Entropy. By recommendation of Cole et al [3], [math]\displaystyle{ T = 2 }[/math] was used to ensure a smooth activation. [math]\displaystyle{ \lambda_1 = 0.025 }[/math] and [math]\displaystyle{ \lambda_2 = 200 }[/math] were chosen so that magnitude of the gradient of each term with respect to [math]\displaystyle{ v_s }[/math] are of similar scale at the [math]\displaystyle{ 1000^{th} }[/math] iteration.
Implementation Details
6 seconds of audio was used to compute the spectogram by taking a Short-time Fourier transform with Hann window of 25mm, hop length of 10ms, and 512 FFT frequenct bands. A CNN-based face detector from Dlib was used to crop the face regions from the frames. The VGG-face features are computed from the resized faces and together with the spectrogram was used for training. There were a total of 1.7 and 0.15 million spectra-feature pairs.
Results
Confusion Matrix and Dataset statistics
In order to determine the similarity between the generated images and the ground truth, a commercial service known as Face++ which classifies faces for distinct attributes (such as gender, ethnicity, etc) was used. Figure 3 gives a confusion matrix based on gender, ethnicity, and age. By examining these matrices, it is seen that the Speech2Face model performs very well on gender, only misclassifying 6% of the time. Similarly, the model performs fairly well on ethnicities, especially with white or Asian faces. Although the model performs worse on black and Indian faces, that can be attributed to the vastly unbalanced data, where 50% of the data represented a white face, and 80% represented a white or Asian face.
Feature Similarity
Another examination of the result is the similarity of features predicted by the Speech2Face model. The cosine, L1, and L2 distance between the facial feature vector produced by the model and the true facial feature vector from the face decoder were computed, and presented, above, in Table 2. A comparison of facial similarity was also done based on the length of audio input. From the table, it is evident that the 6-second audio produced a lower cosine, L1, and L2 distance, resulting in a facial feature vector that is closer to the ground truth.
S2F -> Face retrieval performance
The performance of the model was also examined on how well it could produce the original image. The R@K metric, also known as retrieval performance by recall at K, measures the probability that the K closest images to the model output includes the correct image of the speaker's face. A higher R@K score indicates better performance. From Table 3, above, we see that both the 3-second and 6-second audio showed significant improvement over random chance, with the 6-second audio performing slightly better.
Additional Observations
Ablation studies were carried out to test the effect of audio duration and batch normalization. It was found that the duration of input audio during the training stage had little effect on convergence speed (comparing 3 and 6-second speech segments), while in the test stage longer input speech yields improvement in reconstruction quality. With respect to batch normalization (BN), it was found that without BN reconstructed faces would converge to an average face, while the inclusion of BN led to results which contained much richer facial features.
Conclusion
The report presented a novel study of face reconstruction from audio recordings of a person speaking. The model was demonstrated to be able to predict plausible face reconstructions with similar facial features to real images of the person speaking. We have proved that our method can predict the attributes that the real face of the face is consistent with the real image. By directly reconstructing the face from this cross-modal feature space, we visually verify the existence of cross-mode biometrics. The problem was addressed by learning to align the feature space of speech to that of a pretrained face decoder. The model was trained on millions of videos of people speaking from YouTube. The model was then evaluated by comparing the reconstructed faces with a commercial facial detection service. The authors believe that facial reconstruction allows a more comprehensive view of voice-face correlation compared to predicting individual features, which may lead to new research opportunities and applications.
Discussion and Critiques
There is evidence that the results of the model may be heavily influenced by external factors:
1. Their method of sampling random YouTube videos resulted in an unbalanced sample in terms of ethnicity. Over half of the samples were white. We also saw a large bias in the model's prediction of ethnicity towards white. The bias in the results shows that the model may be overfitting the training data and puts into question what the performance of the model would be when trained and tested on a balanced dataset. Figure (11) highlights this shortcoming: The same man heard speaking in either English or Chinese was predicted to have a "white" appearance or an "asian" appearance respectively.
2. The model was shown to infer different face features based on language. This puts into question how heavily the model depends on the spoken language. The paper mentioned the quality of face reconstruction may be affected by uncommon languages, where English is the most popular language on Youtube(training set). Testing a more controlled sample where all speech recording was of the same language may help address this concern to determine the model's reliance on spoken language.
3. The evaluation of the result is also highly dependent on the Face++ classifiers. Since they compare the age, gender, and ethnicity by running the Face++ classifiers on the original images and the reconstructions to evaluate their model, the model that they create can only be as good as the one they are using to evaluate it. Therefore, any limitations of the Face++ classifier may become a limitation of Speech2Face and may result in a compounding effect on the miss-classification rate.
4. Figure 4.b shows the AVSpeech dataset statistics. However, it doesn't show the statistics about speakers' ethnicity and the language of the video. If we train the model with a more comprehensive dataset that includes enough Asian/Indian English speakers and native language speakers will this increase the accuracy?
5. One concern about the source of the training data, i.e. the Youtube videos, is that resolution varies a lot since the videos are randomly selected. That may be the reason why the proposed model performs badly on some certain features. For example, it is hard to tell the age when the resolution is bad because the wrinkles on the face are neglected.
6. The topic of this project is very interesting, but I highly doubt this model will be practical in real-world problems. Because there are many factors to affect a person's sound in a real-world environment. Sounds such as phone clock, TV, car horn and so on. These sounds will decrease the accuracy of the predicted result of the model.
7. A lot of information can be obtained from someone's voice, this can potentially be useful for detective work and crime scene investigation. In our world of increasing surveillance, public voice recording is quite common and we can reconstruct images of potential suspects based on their voice. In order for this to be achieved, the model has to be thoroughly trained and tested to avoid false positives as it could have a highly destructive outcome for a falsely convicted suspect.
8. This is a very interesting topic, and this summary has a good structure for readers. Since this model uses Youtube to train model, but I think one problem is that most of the YouTubers are adult, and many additional reasons make this dataset highly unbalanced. What is more, some people may have a baby voice, this also could affect the performance of the model. But overall, this is a meaningful topic, it might help police to locate the suspects. So it might be interesting to apply this to the police.
9. In addition, it seems very unlikely that any results coming from this model would ever be held in regard even remotely close to being admissible in court to identify a person of interest until the results are improved and the model can be shown to work in real-world applications. Otherwise, there seems to be very little use for such technology and it could have negative impacts on people if they were to be depicted in an unflattering way by the model based on their voice.
10. Using voice as a factor of constructing the face is a good idea, but it seems like the data they have will have lots of noise and bias. The voice of a video might not come from the person in the video. There are so many YouTubers adjusting their voices before uploading their video and it's really hard to know whether they adjust their voice. Also, most YouTubers are adults so the model cannot have enough training samples about teenagers and kids.
11. It would be interesting to see how the performance changes with different face encoding sizes (instead of just 4096-D) and also difference face models (encoder/decoders) to see if better performance can be achieved. Also given that the dataset used was unbalanced, was the dataset used to train the face model the same dataset? or was a different dataset used (the model was pretrained). This could affect the performance of the model as well.
12. The audio input is transformed into a spectrogram before being used for training. They use STFT with a Hann window of 25 mm, a hop length of 10 ms, and 512 FFT frequency bands. They cite this method from a paper that focuses on speech separation, not speech classification. So, it would be interesting to see if there is a better way to do STFT, possibly with different hyperparameters (eg. different windowing, different number of bands), or if another type of transform (eg. wavelet transform) would have better results.
13. A easy way to get somewhat balanced data is to duplicate the data that are fewer.
14. This problem is interesting but is hard to generalize. This algorithm didn't account for other genders and mixed-race. In addition, the face recognition software Face++ introduces bias which can carry forward to Speech2Face algorithm. Face recognition algorithms are known to have higher error rates classifying darker-skinned individuals. Thus, it'll be tough to apply it to real-life scenarios like identifying suspects.
15. This experiment raises a lot of ethical complications when it comes to possible applications in the real world. Even if this model was highly accurate, the implications of being able to discern a person's racial ethnicity, skin tone, etc. based solely on there voice could play in to inherent biases in the application user and this may end up being an issue that needs to be combatted in future research in this area. Another possible issue is that many people will change their intonation or vocal features based on the context (I'll likely have a different voice pattern in a job interview in terms of projection, intonation, etc. than if I was casually chatting/mumbling with a friend while playing video games for example).
16. Overall a very interesting topic. I want to talk about the technical challenged raised by using the AVSSpeech dataset for training. The paper acknowledges that the AVSSpeech is unbalanced, and 80% of the data are white and Asians. It also says in the results section that "Our model does not perform on other races due to the imbalance in data". There does not seem to be any effort made in balancing the data. I think that there are definitely some data processing techniques that can be used (filtering, data augmentation, etc) to address the class imbalance problem. Not seeing any of these in the paper is a bit disappointing. Another issue I have noticed is that the model aims to predict an average-looking face from certain gender/racial group from voice input, due to ethical considerations. If we cannot reveal the identify of a person, why don't we predict the gender and race directly? Giving an average-looking face does not seem to be the most helpful.
17. Very interesting research paper to be studied and the main objective was also interesting. This research leads to open question which can be applied to another application such as predicting person's face using voice and can be used in more advanced way. The only risk is how the data is obtained from YouTube where data is not consistent.
18. The essay uses millions of natural videos of people speaking to find the correlation between face and voice. Since face and voice are commonly used as the identity of a person, there are many possible research opportunities and applications about improving voice and face unlock.
19. It would be better to have a future work section to discuss the current shortage and explore the possible improvement and applications in the future.
20. While the idea behind Speech2Face is interesting, ethnic profiling is a huge concern and it can further lead to racial discrimination, racism etc. Developers must put more care and thought into applying Speech2Face in tech before deploying the products.
21. It would be helpful if the author could explore the different applications of this project in real life. Speech2face can be helpful during criminal investigation and essentially in scenarios when someone's picture is missing and only voice is available. It would also be helpful if the author could state the importance and need of such kind project in the society.
22. The authors mention that they use the AVSpeech dataset for both training and testing but do not talk about how they split the data. It is possible that the same speakers were used in the training and testing data and so the model is able to recreate a face simply by matching the observed face to the observed audio. This would explain the striking example images shown in the paper.
23. Another interesting application of this research is automated speech or facial animation at scale or in multiple languages. The cutting-edge automated facial animation solution provided by JALI Research Inc is applied in Cyberpunk 2077.
24. It would be interesting to know the model can predict a similar face when one is speaking different languages. A person who is speaking multiple languages can have different tones and accents depending on a language that they speak.
25. The results are actually amazing for the introduction of Speech2Face. As others have mentioned, the researchers might have used a biased dataset of YouTube videos favoring certain ethnicities and their accents and dialects. Thus, it would be nice to also see the data distribution. Additionally it would be nice to see how their model reacts to people who are able to speak multiple languages and see how well Speech2Face generalizes different language pronunciations of one person.
26. The paper introduces Speech2Face and it definitely is one of the major areas of researches in the future. In the paper, the confusion matrix indicates that the model tends to misclassify based on the age of the speaking person. Specifically, the model tends to misclassify between 40-70. It would be interesting to see if the model could improve on its bottleneck by training on more speeches by the age group 40-70.
27. An interesting topic, and as others have mentioned, has many ethical considerations and implications. Particularly in regions where call-recording is permitted, there is dangerous potential to for the technology to be misused to identify and target individuals. It would also be interesting to get a more in depth exploration into how the language spoken and accents have a bias. For example, if a person speaks with a strong British accent, are they classified as white? Particularly for Spanish-speakers, they vary greatly with respect to their skin colour and features, how well does the algorithm work on these individuals. A last nit-pick is the labelling used (i.e. Asian, White, Indian, Black) as this is not accurate since Indians, and moreover South Asians, fall under Asian as well.
28. This topic is quite interesting and it could have great contribution in terms of criminal fight. But as the result, the accuracy is essential. There is still the space for much improvements since to tell a person's face by his/her voice is pretty hard since there are many factors such as oral structure, the language environment and even personality. Great bias could be resulted from these unpredictable factors.
29. This is an interesting topic and could have great use in terms of finding criminals or people when having their voice recorded. However, the voice recording might be noisy and some might include voices of multiple people. It could consider ways to eliminate those factors that might effect the accuracy of the face generation.
30. Most contents described in the paper are very useful. However, YouTube might not be a good enough data source since there are fewer labels to classify. Perhaps, after generating the model, the transfer learning could be done based on Facebook's videos in order to solve the imbalanced problem.
31. This topic is really incredibly interesting and the writers should commend themselves on a job well done. However, Youtube, not only is it an ethnically skewed dataset, but has a non-negligible number of creators who use voice modifiers, auto tune, or a number of other things to change the pitch of their voices, which may lead to the significantly more errors in practical applications. A better dataset to be used could be Skype video calls, or a class room study. Also, judging from the way the model does it's prediction, it seems very prone to overfitting on the dataset, and will not generalize well, since pitch and sound are both incredibly variable across humans.
32. One thing to notice is that the training data used to train the model is downloaded from Youtube, which may be a good site to retrieve a large amount of data. While it allows the possibility that the voices retrieved does not match with the people who made those sounds, claimed by the video. If that is the case, those records will become dirty data, and needs to be cleaned before training the model. Otherwise, there will be some huge misclassifications because some of the training data is not making sense. One way I can think of to improve this problem is that we may train multiple models on different subsets of the original dataset, and combine the results of all the models by taking weighted average.
33. Predicting appearance with sound is a very imaginative research direction. But the author did not explain how to exclude environmental factors in data preprocessing, such as light intensity, facial dress, facial wounds, etc. In the training data set, different sound and image resolutions also affect the effectiveness of the model. The author needs more robustness tests to exclude these factors.
34. The origin of data is primarily Western sourced. Even if taken into account the other ethnicities being selected, the resolution is decompression/compression quality is not maintained for an uploaded platform such as YouTube. Furthermore, since ethnicities are a factor in the model, people not speaking in their mother tongue might provide a very difficult sample for them to match since people tend to sound a certain way (volume, indentation, confidence, tone etc) which can all affect the quality of a recording.
35. With this model's predictions, can it be used by law enforcement to make preemptive identification (with facial recognition) to capture/identify criminals (on surveillance mission)?
36. Interesting article. I wonder how it will result if a participant speaks with a higher/lower pitch, or tries to imitate someone else intentionally.
37. The result section can be expanded a lot more, the original paper studied something really interesting. For example, Craniofacial features were utilized to capture ratios and distances in the face, and how to regenerate a cartoon version of a particular face base on existing face images etc.
References
[1] R. Arandjelovic and A. Zisserman. Look, listen and learn. In IEEE International Conference on Computer Vision (ICCV), 2017.
[2] A. Duarte, F. Roldan, M. Tubau, J. Escur, S. Pascual, A. Salvador, E. Mohedano, K. McGuinness, J. Torres, and X. Giroi-Nieto. Wav2Pix: speech-conditioned face generation using generative adversarial networks. In IEEE International Conference on Acoustics, Speech and Signal Processing (ICASSP), 2019.
[3] F. Cole, D. Belanger, D. Krishnan, A. Sarna, I. Mosseri, and W. T. Freeman. Synthesizing normalized faces from facial identity features. In IEEE Conference on Computer Vision and Pattern Recognition (CVPR), 2017.
[4] L. Castrejon, Y. Aytar, C. Vondrick, H. Pirsiavash, and A. Torralba. Learning aligned cross-modal representations from weakly aligned data. In IEEE Conference on Computer Vision and Pattern Recognition (CVPR), 2016.
[5] O. M. Parkhi, A. Vedaldi, and A. Zisserman. Deep face recognition. In British Machine Vision Conference (BMVC), 2015.
[6] “Overview of GAN Structure | Generative Adversarial Networks,” Google Developers, 2019. [Online]. Available: https://developers.google.com/machine-learning/gan/gan_structure. [Accessed: 02-Dec-2020].