Unsupervised Neural Machine Translation: Difference between revisions
(48 intermediate revisions by 24 users not shown) | |||
Line 2: | Line 2: | ||
= Introduction = | = Introduction = | ||
The paper presents an unsupervised Neural Machine Translation(NMT) method | The paper presents an unsupervised Neural Machine Translation (NMT) method that uses monolingual corpora (single language texts) only. This contrasts with the usual supervised NMT approach which relies on parallel corpora (aligned text) from the source and target languages being available for training. This problem is important because parallel pairing for a majority of languages, e.g. for German-Russian, do not exist. Often, languages can also suffer from having poor resources for translation (e.g. Basque), which could lead to the problem of the dataset being too small (Koehn & Knowles, 2017). | ||
Other authors have recently tried to address this problem | Other authors have recently tried to address this problem using semi-supervised approaches (small set of parallel corpora). Their approaches have included pivoting or triangulation techniques [Chen et al., 2017], and semi supervised approaches [He, 2016]. However, these methods still require a strong cross-lingual signal. The proposed method eliminates the need for cross-lingual information all together and relies solely on monolingual data. The proposed method builds upon the work done recently on unsupervised cross-lingual embeddings by Artetxe et al., 2017 and Zhang et al., 2017. | ||
The general approach of the methodology is to: | The general approach of the methodology is to: | ||
# Use monolingual corpora in the source and target languages to learn | # Use monolingual corpora in the source and target languages to learn single language word embeddings for both languages separately. | ||
# Align the 2 sets of word embeddings | # Align the 2 sets of word embeddings into a single cross lingual (language independent) embedding. | ||
Then iteratively perform: | Then iteratively perform: | ||
# Train an encoder-decoder to reconstruct noisy versions of | # Train an encoder-decoder model to reconstruct noisy versions of sentences in both source and target languages separately. The model uses a single encoder and different decoders for each language. The encoder uses cross lingual word embedding. | ||
# Tune the decoder in each language by back-translating between the source and target language. | # Tune the decoder in each language by back-translating between the source and target language. | ||
Line 18: | Line 18: | ||
===Word Embedding Alignment=== | ===Word Embedding Alignment=== | ||
The paper uses word2vec [Mikolov, 2013] to convert each monolingual corpora to vector embeddings. These embeddings have been shown to contain the contextual and syntactic features independent of language, and so, in theory, there could exist a linear map that maps the embeddings from language L1 to language L2. | The paper uses word2vec [Mikolov, 2013] to convert each monolingual corpora to vector embeddings. They improve the continuous Skip-gram model for learning high-quality distributed vector representations that capture a large number of precise syntactic and semantic word relationships. These embeddings have been shown to contain the contextual and syntactic features independent of language, and so, in theory, there could exist a linear map that maps the embeddings from language L1 to language L2. | ||
Figure 1 shows an example of aligning the word embeddings in English and French. | Figure 1 shows an example of aligning the word embeddings in English and French. | ||
Line 26: | Line 26: | ||
Most cross-lingual word embedding methods use bilingual signals in the form of parallel corpora. Usually, the embedding mapping methods train the embeddings in different languages using monolingual corpora, then use a linear transformation to map them into a shared space based on a bilingual dictionary. | Most cross-lingual word embedding methods use bilingual signals in the form of parallel corpora. Usually, the embedding mapping methods train the embeddings in different languages using monolingual corpora, then use a linear transformation to map them into a shared space based on a bilingual dictionary. | ||
The paper uses the methodology proposed by [Artetxe, 2017] to do cross-lingual embedding aligning in an unsupervised manner and without parallel data. Without going into the details, the general approach of this paper is starting from a seed dictionary of numeral pairings (e.g. 1-1, 2-2, etc.), to iteratively learn the mapping between 2 language embeddings, while concurrently improving the dictionary with the learned mapping at each iteration. | The paper uses the methodology proposed by [Artetxe, 2017] to do cross-lingual embedding aligning in an unsupervised manner and without parallel data. Without going into the details, the general approach of this paper is starting from a seed dictionary of numeral pairings (e.g. 1-1, 2-2, etc.), to iteratively learn the mapping between 2 language embeddings, while concurrently improving the dictionary with the learned mapping at each iteration. This is in contrast to earlier work which used dictionaries of a few thousand words. | ||
===Other related work and inspirations=== | ===Other related work and inspirations=== | ||
==== | ====Statistical Decipherment for Machine Translation==== | ||
There has been significant work in statistical deciphering | There has been significant work in statistical deciphering techniques (decipherment is the discovery of the meaning of texts written in ancient or obscure languages or scripts) to develop a machine translation model from monolingual data (Ravi & Knight, 2011; Dou & Knight, 2012). These techniques treat the source language as ciphertext (encrypted or encoded information because it contains a form of the original plaintext that is unreadable by a human or computer without the proper cipher for decoding) and model the generation process of the ciphertext as a two-stage process, which includes the generation of the original English sequence and the probabilistic replacement of the words in it. This approach takes advantage of the incorporation of syntactic knowledge of the languages. The use of word embeddings has also shown improvements in statistical decipherment. | ||
==== | ====Low-Resource Neural Machine Translation==== | ||
There are also proposals that use techniques other than direct parallel corpora to do | There are also proposals that use techniques other than direct parallel corpora to do NMT. Some use a third intermediate language that is well connected to the source and target languages independently. For example, if we want to translate German into Russian, we can use English as an intermediate language (German-English and then English-Russian) since there are plenty of resources to connect English and other languages. Johnson et al. (2017) show that a multilingual extension of a standard NMT architecture performs reasonably well for language pairs when no parallel data for the source and target data was used during training. Firat et al. (2016) and Chen et al. (2017) showed that the use of advanced models like teacher-student framework can be used to improve over the baseline of translating using a third intermediate language. | ||
Other works use monolingual data in combination with scarce parallel corpora. | Other works use monolingual data in combination with scarce parallel corpora. A simple but effective technique is back-translation [Sennrich et al, 2016]. First, a synthetic parallel corpus in the target language is created. Translated sentence and back-translated to the source language and compared with the original sentence. | ||
The most important contribution to the problem of training an NMT model with monolingual data was from [He, 2016], which trains two agents to translate in opposite directions (e.g. French → English and English → French) and teach each other through reinforcement learning. However, this approach still required a large parallel corpus for a warm start, while | The most important contribution to the problem of training an NMT model with monolingual data was from [He, 2016], which trains two agents to translate in opposite directions (e.g. French → English and English → French) and teach each other through reinforcement learning. However, this approach still required a large parallel corpus for a warm start (about 1.2 million sentences), while this paper does not use parallel data. | ||
= Related Works = | |||
=== 2.1 UNSUPERVISED CROSS-LINGUAL EMBEDDINGS === | |||
A majority of methods for learning cross-lingual word embeddings depend on some bilingual signal at the document level. Embedding mapping methods independently train the embeddings in different languages using monolingual corpora and subsequently learn a linear transformation that maps them to a shared space based on a bilingual dictionary. While the dictionary used in these earlier work typically contains a few thousands entries, Artetxe et al. (2017) propose a simple self-learning extension that gives comparable results with an automatically generated list of numerals, which is used as a shortcut for practical unsupervised learning. | |||
=== 2.2 STATISTICAL DECIPHERMENT FOR MACHINE TRANSLATION === | |||
A considerable body of work in statistical decipherment techniques treat the source language as ciphertext and model the process by which this ciphertext is generated as a two-stage process involving the generation of the original English sequence and the probabilistic replacement of the words in it. The English generative process is modeled using a standard n-gram language model, and the channel model parameters are estimated using either expectation maximization or Bayesian inference. This approach was shown to benefit from the incorporation of syntactic knowledge of the languages involved (Dou & Knight, 2013; Dou et al., 2015). More in line with our proposal, the use of word embeddings has also been shown to bring significant improvements in statistical decipherment for machine translation (Dou et al., 2015). Another newly developed method is using a relatively new deep architecture called Sum-Product network to do machine translation. Hoifung Poon, Pedro Domingos[2011] It is a hybrid model that combines the probabilistic modeling and deep architectures. The main advantage of this model is that it has clear semantics and provide great interoperability, and like many other deep architectures, it can be trained using gradient descent. Sum-product network can be applied in the machine translation field, where one can model the language translation in the following one P(English | French) = p(French / English) * p(English) / p(French), where P(English / French) is the probability that an English text corresponds to a given French text, and P(French/ English) is vice versa. We can use Sum-product network to model each of the above probability and thus doing machine translation. | |||
=== 2.3 LOW-RESOURCE NEURAL MACHINE TRANSLATION === | |||
There have been several proposals to exploit resources other than direct parallel corpora to train NMT systems. This is often necessary when two languages have minimal parallel similarity, but can be connected through a third language (e.g. poor resources for German-Russian connections, but sufficient resource for German-English and English-Russian). A simple approach trains the source language to a middle pivot language, which is then trained to the target language we wish to translate. Beyond this naive approach, various methods such as teacher-student frameworks which generalize to multiple language teaching models has shown significant improvements over the naive baseline benchmarks (Firat et al., 2016b; Chen et al., 2017). | |||
A simple yet effective approach is to create a synthetic parallel corpus by back-translating a monolingual corpus in the target language (Sennrich et al., 2016a). At the same time, Currey et al. (2017) showed that training an NMT system to directly copy target language text is also helpful and complementary with back-translation. Finally, Ramachandran et al. (2017) pre-train the encoder and the decoder in language modeling. Another method trains two agents to translate in opposite directions (e.g. French → English and English → French), and make them teach each other through a reinforcement learning process. This approach still requires a parallel corpus of a considerable size for a good start. | |||
= Methodology = | = Methodology = | ||
The corpora data is first | The corpora data is first preprocessed in a standard way to tokenize and case the words. The authors also experimented with an alternate way of tokenizing words by using Byte-Pair Encoding (BPE) [Sennrich, 2016] (Byte pair encoding or digram coding is a simple form of data compression in which the most common pair of consecutive bytes of data is replaced with a byte that does not occur within that data). BPE has been shown to improve embeddings of rare-words. The vocabulary was limited to the most frequent 50,000 tokens (BPE tokens or words). | ||
The | The tokens were then converted to word embeddings using word2vec with 300 dimensions and then aligned between languages using the method proposed by [Artetxe, 2017]. The alignment method proposed by [Artetxe, 2017] is also used as a baseline to evaluate this model as discussed later in Results. | ||
The translation model uses a standard encoder-decoder model with attention. The encoder is a 2-layer bidirectional RNN, and the decoder is a 2 layer RNN. All RNNs use GRU cells with 600 hidden units | The translation model uses a standard encoder-decoder model with attention. The encoder is a 2-layer bidirectional RNN, and the decoder is a 2 layer RNN. All RNNs use GRU cells with 600 hidden units. The encoder is shared by the source and target language, while the decoder is different for each language. | ||
Although the architecture uses standard models, the proposed system differs from the standard NMT through 3 aspects: | Although the architecture uses standard models, the proposed system differs from the standard NMT through 3 aspects: | ||
Line 51: | Line 67: | ||
#Dual structure: NMT usually are built for one direction translations English<math>\rightarrow</math>French or French<math>\rightarrow</math>English, whereas the proposed model trains both directions at the same time translating English<math>\leftrightarrow</math>French. | #Dual structure: NMT usually are built for one direction translations English<math>\rightarrow</math>French or French<math>\rightarrow</math>English, whereas the proposed model trains both directions at the same time translating English<math>\leftrightarrow</math>French. | ||
#Shared encoder: one encoder is shared for both source and target languages in order to produce a representation in the latent space independent of language, and each decoder learns to transform the representation back to its corresponding language. | #Shared encoder: one encoder is shared for both source and target languages in order to produce a representation in the latent space independent of language, and each decoder learns to transform the representation back to its corresponding language. | ||
#Fixed embeddings in the encoder: Most NMT systems initialize the embeddings and update them during training, whereas the proposed system trains the embeddings in the beginning and keeps these fixed throughout training, so the encoder receives language-independent representations of the words. This requires existing unsupervised methods to create embeddings using monolingual corpora as discussed in the background. | #Fixed embeddings in the encoder: Most NMT systems initialize the embeddings and update them during training, whereas the proposed system trains the embeddings in the beginning and keeps these fixed throughout training, so the encoder receives language-independent representations of the words. This approach ensures that the encoder only learns how to compose the language independent representations to build representations of the larger phrases. This requires existing unsupervised methods to create embeddings using monolingual corpora as discussed in the background. In the proposed method, even though the embeddings used are cross-lingual, the vocabulary used for each language is different. This way if the same word occurs in two different languages and has a different meaning in the respective languages then each word would get a different vector in the respective languages despite being in the same vector space. | ||
[[File:Figure2_lwali.png|600px|center]] | [[File:Figure2_lwali.png|600px|center]] | ||
The translation model iteratively improves the encoder and decoder by performing 2 tasks: Denoising, and Back-translation. | The translation model iteratively improves the encoder and decoder by performing 2 tasks: Denoising, and Back-translation. | ||
'''Note on the need for alignment:''' To train the decoders (in an admittedly “supervised” manner) we make the assumption that they decode from the same latent space. Thus, given a sentence in either language, it needs to represent it in the same latent space to allow training. However, during the back-translation training, the shared encoder stays fixed. This implies that the encoder needs to be set beforehand. For this reason, the process of embedding and alignment is needed. | |||
===Denoising=== | ===Denoising=== | ||
Random noise is added to the input sentences in order to allow the model to learn some structure of languages. Without noise, the model would simply learn to copy the input word by word. Noise also allows the shared encoder to compose the embeddings of both languages in a language-independent fashion, and then be decoded by the language dependent decoder. | |||
Denoising works by reconstructing a noisy version of a sentence back into the original sentence in the same language. In mathematical form, if <math>x</math> is a sentence in language L1: | |||
Denoising works | |||
# Construct <math>C(x)</math>, noisy version of <math>x</math>, | # Construct <math>C(x)</math>, noisy version of <math>x</math>. In the proposed model, <math>C(x)</math> is constructed by randomly swapping contiguous words. If the length of the input sequence <math>x</math> is <math>N</math>, then a total of <math>\frac{N}{2}</math> such swaps are made. | ||
# Input <math>C(x)</math> into the current iteration of the shared encoder and use decoder for L1 to get reconstructed <math>\hat{x}</math>. | # Input <math>C(x)</math> into the current iteration of the shared encoder and use decoder for L1 to get reconstructed <math>\hat{x}</math>. | ||
Line 71: | Line 87: | ||
In other words, the whole system is optimized to take an input sentence in a given language, encode it using the shared encoder, and reconstruct the original sentence using the decoder of that language. | In other words, the whole system is optimized to take an input sentence in a given language, encode it using the shared encoder, and reconstruct the original sentence using the decoder of that language. | ||
The proposed noise function is to perform <math>N/2</math> random swaps of words that are | The proposed noise function is to perform <math>N/2</math> random swaps of words that are contiguous, where <math>N</math> is the number of words in the sentence. This noise model also helps reduce the reliance of the model on the order of words in a sentence which may be different in the source and target languages. The system will also need to correctly learn the internal structure of a language to decode the sentence into the correct order. Thus at the same time, by discouraging the system to rely too much on the word order of input sequence, better account for the actual order across different languages can be done. | ||
===Back-Translation=== | ===Back-Translation=== | ||
With only denoising, the system doesn't have a goal to improve the actual translation. Back-translation works by using the decoder of the target language to create a translation, then encoding this translation and decoding again using the source decoder to reconstruct | With only denoising, the system doesn't have a goal to improve the actual translation. Back-translation works by using the decoder of the target language to create a translation, then encoding this translation and decoding again using the source decoder to reconstruct the original sentence. In mathematical form, if <math>C(x)</math> is a noisy version of sentence <math>x</math> in language L1: | ||
# Input <math>C(x)</math> into the current iteration of shared encoder and the decoder in L2 to construct translation <math>y</math> in | # Input <math>C(x)</math> into the current iteration of shared encoder and the decoder in L2 to construct translation <math>y</math> in L2, | ||
# Construct <math>C(y)</math>, noisy version of translation <math>y</math>, | # Construct <math>C(y)</math>, noisy version of translation <math>y</math>, | ||
# Input <math>C(y)</math> into the current iteration of shared encoder and the decoder in L1 to reconstruct <math>\hat{x}</math> in L1. | # Input <math>C(y)</math> into the current iteration of shared encoder and the decoder in L1 to reconstruct <math>\hat{x}</math> in L1. | ||
Line 83: | Line 99: | ||
The training objective is to minimize the cross entropy loss between <math>{x}</math> and <math>\hat{x}</math>. | The training objective is to minimize the cross entropy loss between <math>{x}</math> and <math>\hat{x}</math>. | ||
Contrary to standard back-translation that uses an independent model to back-translate the entire corpus at | This approach alleviates issues that would have resulted from the training procedure only dealing with a single language at a time. The corpus of a language is converted to a synthetic translation, and trained to predict the original sentence from this translation. | ||
Contrary to standard back-translation that uses an independent model to back-translate the entire corpus at once, the system uses mini-batches and the dual architecture to generate pseudo-translations and then train the model with the translation, improving the model iteratively as the training progresses. | |||
===Training=== | ===Training=== | ||
Line 90: | Line 108: | ||
During decoding, greedy decoding was used at training time for back-translation, but actual inference at test time was done using beam-search with a beam size of 12. | During decoding, greedy decoding was used at training time for back-translation, but actual inference at test time was done using beam-search with a beam size of 12. | ||
The authors use Adam as their optimizer with a learning rate of α = 0.0002 (Kingma & Ba, 2015). During training, dropout regularization is implemented with a drop probability p = 0.3. Given that no parallel data is used for development purposes, the authors perform a fixed number of iterations (300,000) to train each variant. | |||
Considering recently demonstrated weaker convergence of Adam (compared to SGD), repeating the experiments with other optimizers might provide better results. | |||
=Experiments and Results= | =Experiments and Results= | ||
The model | The model was evaluated using the Bilingual Evaluation Understudy (BLEU) Score, which is typically used to evaluate the quality of the translation, using a reference (ground-truth) translation. | ||
The paper | The paper trained translation model under 3 different settings to compare the performance (Table 1). All training and testing data used was from a standard NMT dataset, WMT'14. | ||
[[File:Table1_lwali.png|600px|center]] | [[File:Table1_lwali.png|600px|center]] | ||
The results exhibit that for the proposed system to work properly, back-translation is necessary. The denoising technique alone is below the baseline while big improvements appear when introducing back-translation. | |||
===Unsupervised=== | ===Unsupervised=== | ||
Line 104: | Line 126: | ||
The model only has access to monolingual corpora, using the News Crawl corpus with articles from 2007 to 2013. The baseline for unsupervised is the method proposed by [Artetxe, 2017], which was the unsupervised word vector alignment method discussed in the Background section. | The model only has access to monolingual corpora, using the News Crawl corpus with articles from 2007 to 2013. The baseline for unsupervised is the method proposed by [Artetxe, 2017], which was the unsupervised word vector alignment method discussed in the Background section. | ||
The paper adds each component piece-wise when doing an evaluation to test the impact each piece has on the final score. As shown in | The paper adds each component piece-wise when doing an evaluation to test the impact each piece has on the final score. As shown in Table 1, Unsupervised results compared to the baseline of word-by-word results are strong, with improvement between 40% to 140%. Results also show that back-translation is essential. Denoising doesn't show a big improvement however it is required for back-translation, because otherwise, back-translation would translate nonsensical sentences. The addition of back-translation, however, does show large improvement on all tested cases. | ||
For the BPE experiment, results show it helps in some language pairs but detract in some other language pairs. This is because while BPE helped to translate some rare words, it increased the error rates in other words. | For the BPE experiment, results show it helps in some language pairs but detract in some other language pairs. This is because while BPE helped to translate some rare words, it increased the error rates in other words. It also did not perform well when translating named entities which occur infrequently. | ||
===Semi-supervised=== | ===Semi-supervised=== | ||
Line 112: | Line 134: | ||
Since there is often some small parallel data but not enough to train a Neural Machine Translation system, the authors test a semi-supervised setting with the same monolingual data from the unsupervised settings together with either 10,000 or 100,000 random sentence pairs from the News Commentary parallel corpus. The supervision is included to improve the model during the back-translation stage to directly predict sentences that are in the parallel corpus. | Since there is often some small parallel data but not enough to train a Neural Machine Translation system, the authors test a semi-supervised setting with the same monolingual data from the unsupervised settings together with either 10,000 or 100,000 random sentence pairs from the News Commentary parallel corpus. The supervision is included to improve the model during the back-translation stage to directly predict sentences that are in the parallel corpus. | ||
Table 1 shows that the model can greatly benefit from the addition of a small parallel corpus to the monolingual corpora. It is surprising that semi-supervised in row 6 outperforms supervised in row 7, one possible explanation is that both the semi-supervised training set and the test set belong to the news domain, whereas the supervised training set is all domains of corpora. | |||
===Supervised=== | ===Supervised=== | ||
Line 118: | Line 140: | ||
This setting provides an upper bound to the unsupervised proposed system. The data used was the combination of all parallel corpora provided at WMT 2014, which includes Europarl, Common Crawl and News Commentary for both language pairs plus the UN and the Gigaword corpus for French- English. Moreover, the authors use the same subsets of News Commentary alone to run the separate experiments in order to compare with the semi-supervised scenario. | This setting provides an upper bound to the unsupervised proposed system. The data used was the combination of all parallel corpora provided at WMT 2014, which includes Europarl, Common Crawl and News Commentary for both language pairs plus the UN and the Gigaword corpus for French- English. Moreover, the authors use the same subsets of News Commentary alone to run the separate experiments in order to compare with the semi-supervised scenario. | ||
The Comparable NMT was trained using the same proposed model except it does not use monolingual corpora, and consequently, it was trained without denoising and back-translation. The proposed model under a supervised setting does much worse than the state of the NMT in row 10, which suggests that adding the additional constraints to enable unsupervised learning also limits the potential performance. To improve these results, the authors also suggest | The Comparable NMT was trained using the same proposed model except it does not use monolingual corpora, and consequently, it was trained without denoising and back-translation. The proposed model under a supervised setting does much worse than the state of the NMT in row 10, which suggests that adding the additional constraints to enable unsupervised learning also limits the potential performance. To improve these results, the authors also suggest using larger models, longer training times, and incorporating several well-known NMT techniques. | ||
===Qualitative Analysis=== | ===Qualitative Analysis=== | ||
Line 124: | Line 146: | ||
[[File:Table2_lwali.png|600px|center]] | [[File:Table2_lwali.png|600px|center]] | ||
Table 2 shows 4 examples of French to English translations, which shows that the high-quality translations are | Table 2 shows 4 examples of French to English translations, which shows that the high-quality translations are produced by the proposed system, and this system adequately models non-trivial translation relations. Example 1 and 2 show that the model is able to not only go beyond a literal word-by-word substitution but also model structural differences in the languages (ex.e, it correctly translates "l’aeroport international de Los Angeles" as "Los Angeles International Airport", and it is capable of producing high-quality translations of long and more complex sentences. However, in Example 3 and 4, the system failed to translate the months and numbers correctly and having difficulty with comprehending odd sentence structures, which means that the proposed system has limitations. Especially, the authors point that the proposed model has difficulties to preserve some concrete details from source sentences. Results also show, the proposed model's translation quality often lags behind that of a standard supervised NMT system and also there are also some cases where there are both fluency and adequacy problems that severely hinders understanding the original message from the proposed translation, suggesting that there is still room for improvement and possible future work. | ||
=Conclusions and Future Work= | =Conclusions and Future Work= | ||
Line 130: | Line 152: | ||
The paper presented an unsupervised model to perform translations with monolingual corpora by using an attention-based encoder-decoder system and training using denoise and back-translation. | The paper presented an unsupervised model to perform translations with monolingual corpora by using an attention-based encoder-decoder system and training using denoise and back-translation. | ||
Although experimental results show that the proposed model is effective as an unsupervised approach, there is significant room for improvement when using the model in a supervised way, suggesting the model is limited by the architectural modifications. Some ideas for future improvement include: | Although experimental results show that the proposed model is effective as an unsupervised approach, there is significant room for improvement when using the model in a supervised way, combining the proposed method with a small parallel corpus, suggesting the model is limited by the architectural modifications. Some ideas for future improvement include: | ||
*Instead of using fixed cross-lingual word embeddings at the beginning which forces the encoder to learn a common representation for both languages, progressively update the weight of the embeddings as training progresses. | *Instead of using fixed cross-lingual word embeddings at the beginning which forces the encoder to learn a common representation for both languages, progressively update the weight of the embeddings as training progresses. | ||
*Decouple the shared encoder into 2 independent encoders at some point during training | *Decouple the shared encoder into 2 independent encoders at some point during training | ||
Line 140: | Line 162: | ||
While the idea is interesting and the results are impressive for an unsupervised approach, much of the model had actually already been proposed by other papers that are referenced. The paper doesn't add a lot of new ideas but only builds on existing techniques and combines them in a different way to achieve good experimental results. The paper is not a significant algorithmic contribution. | While the idea is interesting and the results are impressive for an unsupervised approach, much of the model had actually already been proposed by other papers that are referenced. The paper doesn't add a lot of new ideas but only builds on existing techniques and combines them in a different way to achieve good experimental results. The paper is not a significant algorithmic contribution. | ||
As pointed out, in order to critically analyze the effect of the algorithm, we need to formulate the algorithm in terms of mathematics. | |||
The results showed that the proposed system performed far worse than the state of the art when used in a supervised setting, which is concerning and shows that the techniques used creates a limitation and a ceiling for performance. | The results showed that the proposed system performed far worse than the state of the art when used in a supervised setting, which is concerning and shows that the techniques used creates a limitation and a ceiling for performance. | ||
Line 149: | Line 173: | ||
The results comparison could have shown how the semi-supervised version of the model scores compared to other semi-supervised approaches as touched on in the other works section. | The results comparison could have shown how the semi-supervised version of the model scores compared to other semi-supervised approaches as touched on in the other works section. | ||
Their qualitative analysis just checks whether their proposed unsupervised NMT generates sensible translation. It is limited and it needs further detailed analysis regarding the characteristics and properties of translation which is generated by unsupervised NMT. | As the authors didnot try practises like, coverage penalty , these approaches might improve their results. | ||
Their qualitative analysis just checks whether their proposed unsupervised NMT generates a sensible translation. It is limited and it needs further detailed analysis regarding the characteristics and properties of translation which is generated by unsupervised NMT. | |||
One interesting research direction might explore the performance of the proposed approach over different semantic contexts. In other words, as a parallel work, a quantitative comparison could be made between the translation of texts from areas such as novels, news, technical reports, among others. The reason is people speaking different languages might use similar phrases for technical writing but very different for expressing emotions or feelings. | |||
* (As pointed out by an | * (As pointed out by an anonymous reviewer [https://openreview.net/forum?id=Sy2ogebAW])Future work is vague: “we would like to detect and mitigate the specific causes…” “We also think that a better handling of rare words…” That’s great, but how will you do these things? Do you have specific reasons to think this, or ideas on how to approach them? Otherwise, this is just hand-waving. | ||
= References = | = References = | ||
Line 162: | Line 190: | ||
#'''[Dou & Knight, 2012]''' Qing Dou and Kevin Knight, "Large scale decipherment for out-of-domain machine translation." | #'''[Dou & Knight, 2012]''' Qing Dou and Kevin Knight, "Large scale decipherment for out-of-domain machine translation." | ||
#'''[Johnson et al. 2017]''' Melvin Johnson,et al, "Google’s multilingual neural machine translation system: Enabling zero-shot translation." | #'''[Johnson et al. 2017]''' Melvin Johnson,et al, "Google’s multilingual neural machine translation system: Enabling zero-shot translation." | ||
#'''[Zhang et al. 2017]''' Meng Zhang, Yang Liu, Huanbo Luan, and Maosong Sun. "Adversarial training for unsupervised bilingual lexicon induction" | |||
#'''[ Koehn & Knowles, 2017]''' Philipp Koehn and Rebecca Knowles. Six challenges for neural machine translation. | |||
#'''[Chen et al., 2017]''' Yun Chen, Yang Liu, Yong Cheng, and Victor O.K. Li. A teacher-student framework for zero-resource neural machine translation. |
Latest revision as of 00:28, 17 December 2018
This paper was published in ICLR 2018, authored by Mikel Artetxe, Gorka Labaka, Eneko Agirre, and Kyunghyun Cho. Open source implementation of this paper is available here
Introduction
The paper presents an unsupervised Neural Machine Translation (NMT) method that uses monolingual corpora (single language texts) only. This contrasts with the usual supervised NMT approach which relies on parallel corpora (aligned text) from the source and target languages being available for training. This problem is important because parallel pairing for a majority of languages, e.g. for German-Russian, do not exist. Often, languages can also suffer from having poor resources for translation (e.g. Basque), which could lead to the problem of the dataset being too small (Koehn & Knowles, 2017).
Other authors have recently tried to address this problem using semi-supervised approaches (small set of parallel corpora). Their approaches have included pivoting or triangulation techniques [Chen et al., 2017], and semi supervised approaches [He, 2016]. However, these methods still require a strong cross-lingual signal. The proposed method eliminates the need for cross-lingual information all together and relies solely on monolingual data. The proposed method builds upon the work done recently on unsupervised cross-lingual embeddings by Artetxe et al., 2017 and Zhang et al., 2017.
The general approach of the methodology is to:
- Use monolingual corpora in the source and target languages to learn single language word embeddings for both languages separately.
- Align the 2 sets of word embeddings into a single cross lingual (language independent) embedding.
Then iteratively perform:
- Train an encoder-decoder model to reconstruct noisy versions of sentences in both source and target languages separately. The model uses a single encoder and different decoders for each language. The encoder uses cross lingual word embedding.
- Tune the decoder in each language by back-translating between the source and target language.
Background
Word Embedding Alignment
The paper uses word2vec [Mikolov, 2013] to convert each monolingual corpora to vector embeddings. They improve the continuous Skip-gram model for learning high-quality distributed vector representations that capture a large number of precise syntactic and semantic word relationships. These embeddings have been shown to contain the contextual and syntactic features independent of language, and so, in theory, there could exist a linear map that maps the embeddings from language L1 to language L2.
Figure 1 shows an example of aligning the word embeddings in English and French.
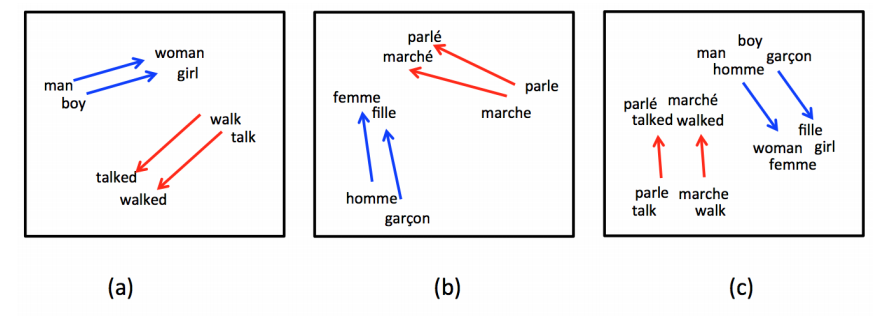
Most cross-lingual word embedding methods use bilingual signals in the form of parallel corpora. Usually, the embedding mapping methods train the embeddings in different languages using monolingual corpora, then use a linear transformation to map them into a shared space based on a bilingual dictionary.
The paper uses the methodology proposed by [Artetxe, 2017] to do cross-lingual embedding aligning in an unsupervised manner and without parallel data. Without going into the details, the general approach of this paper is starting from a seed dictionary of numeral pairings (e.g. 1-1, 2-2, etc.), to iteratively learn the mapping between 2 language embeddings, while concurrently improving the dictionary with the learned mapping at each iteration. This is in contrast to earlier work which used dictionaries of a few thousand words.
Statistical Decipherment for Machine Translation
There has been significant work in statistical deciphering techniques (decipherment is the discovery of the meaning of texts written in ancient or obscure languages or scripts) to develop a machine translation model from monolingual data (Ravi & Knight, 2011; Dou & Knight, 2012). These techniques treat the source language as ciphertext (encrypted or encoded information because it contains a form of the original plaintext that is unreadable by a human or computer without the proper cipher for decoding) and model the generation process of the ciphertext as a two-stage process, which includes the generation of the original English sequence and the probabilistic replacement of the words in it. This approach takes advantage of the incorporation of syntactic knowledge of the languages. The use of word embeddings has also shown improvements in statistical decipherment.
Low-Resource Neural Machine Translation
There are also proposals that use techniques other than direct parallel corpora to do NMT. Some use a third intermediate language that is well connected to the source and target languages independently. For example, if we want to translate German into Russian, we can use English as an intermediate language (German-English and then English-Russian) since there are plenty of resources to connect English and other languages. Johnson et al. (2017) show that a multilingual extension of a standard NMT architecture performs reasonably well for language pairs when no parallel data for the source and target data was used during training. Firat et al. (2016) and Chen et al. (2017) showed that the use of advanced models like teacher-student framework can be used to improve over the baseline of translating using a third intermediate language.
Other works use monolingual data in combination with scarce parallel corpora. A simple but effective technique is back-translation [Sennrich et al, 2016]. First, a synthetic parallel corpus in the target language is created. Translated sentence and back-translated to the source language and compared with the original sentence.
The most important contribution to the problem of training an NMT model with monolingual data was from [He, 2016], which trains two agents to translate in opposite directions (e.g. French → English and English → French) and teach each other through reinforcement learning. However, this approach still required a large parallel corpus for a warm start (about 1.2 million sentences), while this paper does not use parallel data.
Related Works
2.1 UNSUPERVISED CROSS-LINGUAL EMBEDDINGS
A majority of methods for learning cross-lingual word embeddings depend on some bilingual signal at the document level. Embedding mapping methods independently train the embeddings in different languages using monolingual corpora and subsequently learn a linear transformation that maps them to a shared space based on a bilingual dictionary. While the dictionary used in these earlier work typically contains a few thousands entries, Artetxe et al. (2017) propose a simple self-learning extension that gives comparable results with an automatically generated list of numerals, which is used as a shortcut for practical unsupervised learning.
2.2 STATISTICAL DECIPHERMENT FOR MACHINE TRANSLATION
A considerable body of work in statistical decipherment techniques treat the source language as ciphertext and model the process by which this ciphertext is generated as a two-stage process involving the generation of the original English sequence and the probabilistic replacement of the words in it. The English generative process is modeled using a standard n-gram language model, and the channel model parameters are estimated using either expectation maximization or Bayesian inference. This approach was shown to benefit from the incorporation of syntactic knowledge of the languages involved (Dou & Knight, 2013; Dou et al., 2015). More in line with our proposal, the use of word embeddings has also been shown to bring significant improvements in statistical decipherment for machine translation (Dou et al., 2015). Another newly developed method is using a relatively new deep architecture called Sum-Product network to do machine translation. Hoifung Poon, Pedro Domingos[2011] It is a hybrid model that combines the probabilistic modeling and deep architectures. The main advantage of this model is that it has clear semantics and provide great interoperability, and like many other deep architectures, it can be trained using gradient descent. Sum-product network can be applied in the machine translation field, where one can model the language translation in the following one P(English | French) = p(French / English) * p(English) / p(French), where P(English / French) is the probability that an English text corresponds to a given French text, and P(French/ English) is vice versa. We can use Sum-product network to model each of the above probability and thus doing machine translation.
2.3 LOW-RESOURCE NEURAL MACHINE TRANSLATION
There have been several proposals to exploit resources other than direct parallel corpora to train NMT systems. This is often necessary when two languages have minimal parallel similarity, but can be connected through a third language (e.g. poor resources for German-Russian connections, but sufficient resource for German-English and English-Russian). A simple approach trains the source language to a middle pivot language, which is then trained to the target language we wish to translate. Beyond this naive approach, various methods such as teacher-student frameworks which generalize to multiple language teaching models has shown significant improvements over the naive baseline benchmarks (Firat et al., 2016b; Chen et al., 2017).
A simple yet effective approach is to create a synthetic parallel corpus by back-translating a monolingual corpus in the target language (Sennrich et al., 2016a). At the same time, Currey et al. (2017) showed that training an NMT system to directly copy target language text is also helpful and complementary with back-translation. Finally, Ramachandran et al. (2017) pre-train the encoder and the decoder in language modeling. Another method trains two agents to translate in opposite directions (e.g. French → English and English → French), and make them teach each other through a reinforcement learning process. This approach still requires a parallel corpus of a considerable size for a good start.
Methodology
The corpora data is first preprocessed in a standard way to tokenize and case the words. The authors also experimented with an alternate way of tokenizing words by using Byte-Pair Encoding (BPE) [Sennrich, 2016] (Byte pair encoding or digram coding is a simple form of data compression in which the most common pair of consecutive bytes of data is replaced with a byte that does not occur within that data). BPE has been shown to improve embeddings of rare-words. The vocabulary was limited to the most frequent 50,000 tokens (BPE tokens or words).
The tokens were then converted to word embeddings using word2vec with 300 dimensions and then aligned between languages using the method proposed by [Artetxe, 2017]. The alignment method proposed by [Artetxe, 2017] is also used as a baseline to evaluate this model as discussed later in Results.
The translation model uses a standard encoder-decoder model with attention. The encoder is a 2-layer bidirectional RNN, and the decoder is a 2 layer RNN. All RNNs use GRU cells with 600 hidden units. The encoder is shared by the source and target language, while the decoder is different for each language.
Although the architecture uses standard models, the proposed system differs from the standard NMT through 3 aspects:
- Dual structure: NMT usually are built for one direction translations English[math]\displaystyle{ \rightarrow }[/math]French or French[math]\displaystyle{ \rightarrow }[/math]English, whereas the proposed model trains both directions at the same time translating English[math]\displaystyle{ \leftrightarrow }[/math]French.
- Shared encoder: one encoder is shared for both source and target languages in order to produce a representation in the latent space independent of language, and each decoder learns to transform the representation back to its corresponding language.
- Fixed embeddings in the encoder: Most NMT systems initialize the embeddings and update them during training, whereas the proposed system trains the embeddings in the beginning and keeps these fixed throughout training, so the encoder receives language-independent representations of the words. This approach ensures that the encoder only learns how to compose the language independent representations to build representations of the larger phrases. This requires existing unsupervised methods to create embeddings using monolingual corpora as discussed in the background. In the proposed method, even though the embeddings used are cross-lingual, the vocabulary used for each language is different. This way if the same word occurs in two different languages and has a different meaning in the respective languages then each word would get a different vector in the respective languages despite being in the same vector space.
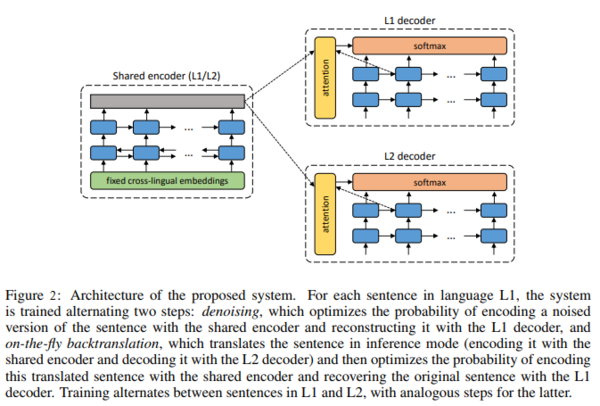
The translation model iteratively improves the encoder and decoder by performing 2 tasks: Denoising, and Back-translation.
Note on the need for alignment: To train the decoders (in an admittedly “supervised” manner) we make the assumption that they decode from the same latent space. Thus, given a sentence in either language, it needs to represent it in the same latent space to allow training. However, during the back-translation training, the shared encoder stays fixed. This implies that the encoder needs to be set beforehand. For this reason, the process of embedding and alignment is needed.
Denoising
Random noise is added to the input sentences in order to allow the model to learn some structure of languages. Without noise, the model would simply learn to copy the input word by word. Noise also allows the shared encoder to compose the embeddings of both languages in a language-independent fashion, and then be decoded by the language dependent decoder.
Denoising works by reconstructing a noisy version of a sentence back into the original sentence in the same language. In mathematical form, if [math]\displaystyle{ x }[/math] is a sentence in language L1:
- Construct [math]\displaystyle{ C(x) }[/math], noisy version of [math]\displaystyle{ x }[/math]. In the proposed model, [math]\displaystyle{ C(x) }[/math] is constructed by randomly swapping contiguous words. If the length of the input sequence [math]\displaystyle{ x }[/math] is [math]\displaystyle{ N }[/math], then a total of [math]\displaystyle{ \frac{N}{2} }[/math] such swaps are made.
- Input [math]\displaystyle{ C(x) }[/math] into the current iteration of the shared encoder and use decoder for L1 to get reconstructed [math]\displaystyle{ \hat{x} }[/math].
The training objective is to minimize the cross entropy loss between [math]\displaystyle{ {x} }[/math] and [math]\displaystyle{ \hat{x} }[/math].
In other words, the whole system is optimized to take an input sentence in a given language, encode it using the shared encoder, and reconstruct the original sentence using the decoder of that language.
The proposed noise function is to perform [math]\displaystyle{ N/2 }[/math] random swaps of words that are contiguous, where [math]\displaystyle{ N }[/math] is the number of words in the sentence. This noise model also helps reduce the reliance of the model on the order of words in a sentence which may be different in the source and target languages. The system will also need to correctly learn the internal structure of a language to decode the sentence into the correct order. Thus at the same time, by discouraging the system to rely too much on the word order of input sequence, better account for the actual order across different languages can be done.
Back-Translation
With only denoising, the system doesn't have a goal to improve the actual translation. Back-translation works by using the decoder of the target language to create a translation, then encoding this translation and decoding again using the source decoder to reconstruct the original sentence. In mathematical form, if [math]\displaystyle{ C(x) }[/math] is a noisy version of sentence [math]\displaystyle{ x }[/math] in language L1:
- Input [math]\displaystyle{ C(x) }[/math] into the current iteration of shared encoder and the decoder in L2 to construct translation [math]\displaystyle{ y }[/math] in L2,
- Construct [math]\displaystyle{ C(y) }[/math], noisy version of translation [math]\displaystyle{ y }[/math],
- Input [math]\displaystyle{ C(y) }[/math] into the current iteration of shared encoder and the decoder in L1 to reconstruct [math]\displaystyle{ \hat{x} }[/math] in L1.
The training objective is to minimize the cross entropy loss between [math]\displaystyle{ {x} }[/math] and [math]\displaystyle{ \hat{x} }[/math].
This approach alleviates issues that would have resulted from the training procedure only dealing with a single language at a time. The corpus of a language is converted to a synthetic translation, and trained to predict the original sentence from this translation.
Contrary to standard back-translation that uses an independent model to back-translate the entire corpus at once, the system uses mini-batches and the dual architecture to generate pseudo-translations and then train the model with the translation, improving the model iteratively as the training progresses.
Training
Training is done by alternating these 2 objectives from mini-batch to mini-batch. Each iteration would perform one mini-batch of denoising for L1, another one for L2, one mini-batch of back-translation from L1 to L2, and another one from L2 to L1. The procedure is repeated until convergence. During decoding, greedy decoding was used at training time for back-translation, but actual inference at test time was done using beam-search with a beam size of 12.
The authors use Adam as their optimizer with a learning rate of α = 0.0002 (Kingma & Ba, 2015). During training, dropout regularization is implemented with a drop probability p = 0.3. Given that no parallel data is used for development purposes, the authors perform a fixed number of iterations (300,000) to train each variant.
Considering recently demonstrated weaker convergence of Adam (compared to SGD), repeating the experiments with other optimizers might provide better results.
Experiments and Results
The model was evaluated using the Bilingual Evaluation Understudy (BLEU) Score, which is typically used to evaluate the quality of the translation, using a reference (ground-truth) translation.
The paper trained translation model under 3 different settings to compare the performance (Table 1). All training and testing data used was from a standard NMT dataset, WMT'14.
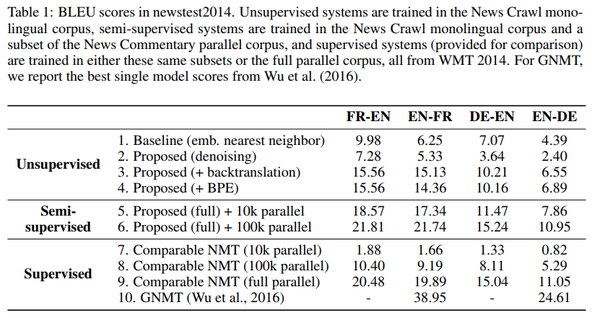
The results exhibit that for the proposed system to work properly, back-translation is necessary. The denoising technique alone is below the baseline while big improvements appear when introducing back-translation.
Unsupervised
The model only has access to monolingual corpora, using the News Crawl corpus with articles from 2007 to 2013. The baseline for unsupervised is the method proposed by [Artetxe, 2017], which was the unsupervised word vector alignment method discussed in the Background section.
The paper adds each component piece-wise when doing an evaluation to test the impact each piece has on the final score. As shown in Table 1, Unsupervised results compared to the baseline of word-by-word results are strong, with improvement between 40% to 140%. Results also show that back-translation is essential. Denoising doesn't show a big improvement however it is required for back-translation, because otherwise, back-translation would translate nonsensical sentences. The addition of back-translation, however, does show large improvement on all tested cases.
For the BPE experiment, results show it helps in some language pairs but detract in some other language pairs. This is because while BPE helped to translate some rare words, it increased the error rates in other words. It also did not perform well when translating named entities which occur infrequently.
Semi-supervised
Since there is often some small parallel data but not enough to train a Neural Machine Translation system, the authors test a semi-supervised setting with the same monolingual data from the unsupervised settings together with either 10,000 or 100,000 random sentence pairs from the News Commentary parallel corpus. The supervision is included to improve the model during the back-translation stage to directly predict sentences that are in the parallel corpus.
Table 1 shows that the model can greatly benefit from the addition of a small parallel corpus to the monolingual corpora. It is surprising that semi-supervised in row 6 outperforms supervised in row 7, one possible explanation is that both the semi-supervised training set and the test set belong to the news domain, whereas the supervised training set is all domains of corpora.
Supervised
This setting provides an upper bound to the unsupervised proposed system. The data used was the combination of all parallel corpora provided at WMT 2014, which includes Europarl, Common Crawl and News Commentary for both language pairs plus the UN and the Gigaword corpus for French- English. Moreover, the authors use the same subsets of News Commentary alone to run the separate experiments in order to compare with the semi-supervised scenario.
The Comparable NMT was trained using the same proposed model except it does not use monolingual corpora, and consequently, it was trained without denoising and back-translation. The proposed model under a supervised setting does much worse than the state of the NMT in row 10, which suggests that adding the additional constraints to enable unsupervised learning also limits the potential performance. To improve these results, the authors also suggest using larger models, longer training times, and incorporating several well-known NMT techniques.
Qualitative Analysis
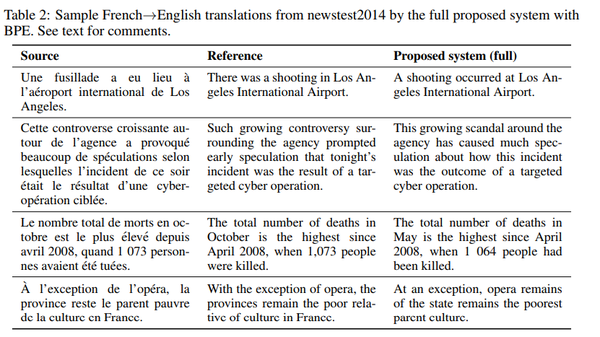
Table 2 shows 4 examples of French to English translations, which shows that the high-quality translations are produced by the proposed system, and this system adequately models non-trivial translation relations. Example 1 and 2 show that the model is able to not only go beyond a literal word-by-word substitution but also model structural differences in the languages (ex.e, it correctly translates "l’aeroport international de Los Angeles" as "Los Angeles International Airport", and it is capable of producing high-quality translations of long and more complex sentences. However, in Example 3 and 4, the system failed to translate the months and numbers correctly and having difficulty with comprehending odd sentence structures, which means that the proposed system has limitations. Especially, the authors point that the proposed model has difficulties to preserve some concrete details from source sentences. Results also show, the proposed model's translation quality often lags behind that of a standard supervised NMT system and also there are also some cases where there are both fluency and adequacy problems that severely hinders understanding the original message from the proposed translation, suggesting that there is still room for improvement and possible future work.
Conclusions and Future Work
The paper presented an unsupervised model to perform translations with monolingual corpora by using an attention-based encoder-decoder system and training using denoise and back-translation.
Although experimental results show that the proposed model is effective as an unsupervised approach, there is significant room for improvement when using the model in a supervised way, combining the proposed method with a small parallel corpus, suggesting the model is limited by the architectural modifications. Some ideas for future improvement include:
- Instead of using fixed cross-lingual word embeddings at the beginning which forces the encoder to learn a common representation for both languages, progressively update the weight of the embeddings as training progresses.
- Decouple the shared encoder into 2 independent encoders at some point during training
- Progressively reduce the noise level
- Incorporate character level information into the model, which might help address some of the adequacy issues observed in our manual analysis
- Use other noise/denoising techniques, and analyze their effect in relation to the typological divergences of different language pairs.
Critique
While the idea is interesting and the results are impressive for an unsupervised approach, much of the model had actually already been proposed by other papers that are referenced. The paper doesn't add a lot of new ideas but only builds on existing techniques and combines them in a different way to achieve good experimental results. The paper is not a significant algorithmic contribution.
As pointed out, in order to critically analyze the effect of the algorithm, we need to formulate the algorithm in terms of mathematics.
The results showed that the proposed system performed far worse than the state of the art when used in a supervised setting, which is concerning and shows that the techniques used creates a limitation and a ceiling for performance.
Additionally, there was no rigorous hyperparameter exploration/optimization for the model. As a result, it is difficult to conclude whether the performance limit observed in the constrained supervised model is the absolute limit, or whether this could be overcome in both supervised/unsupervised models with the right constraints to achieve more competitive results.
The best results shown are between two very closely related languages(English and French), and does much worse for English - German, even though English and German are also closely related (but less so than English and French) which suggests that the model may not be successful at translating between distant language pairs. More testing would be interesting to see.
The results comparison could have shown how the semi-supervised version of the model scores compared to other semi-supervised approaches as touched on in the other works section.
As the authors didnot try practises like, coverage penalty , these approaches might improve their results.
Their qualitative analysis just checks whether their proposed unsupervised NMT generates a sensible translation. It is limited and it needs further detailed analysis regarding the characteristics and properties of translation which is generated by unsupervised NMT.
One interesting research direction might explore the performance of the proposed approach over different semantic contexts. In other words, as a parallel work, a quantitative comparison could be made between the translation of texts from areas such as novels, news, technical reports, among others. The reason is people speaking different languages might use similar phrases for technical writing but very different for expressing emotions or feelings.
- (As pointed out by an anonymous reviewer [1])Future work is vague: “we would like to detect and mitigate the specific causes…” “We also think that a better handling of rare words…” That’s great, but how will you do these things? Do you have specific reasons to think this, or ideas on how to approach them? Otherwise, this is just hand-waving.
References
- [Mikolov, 2013] Tomas Mikolov, Ilya Sutskever, Kai Chen, Greg S Corrado, and Jeff Dean. "Distributed representations of words and phrases and their compositionality."
- [Artetxe, 2017] Mikel Artetxe, Gorka Labaka, Eneko Agirre, "Learning bilingual word embeddings with (almost) no bilingual data".
- [Gouws,2016] Stephan Gouws, Yoshua Bengio, Greg Corrado, "BilBOWA: Fast Bilingual Distributed Representations without Word Alignments."
- [He, 2016] Di He, Yingce Xia, Tao Qin, Liwei Wang, Nenghai Yu, Tieyan Liu, and Wei-Ying Ma. "Dual learning for machine translation."
- [Sennrich,2016] Rico Sennrich and Barry Haddow and Alexandra Birch, "Neural Machine Translation of Rare Words with Subword Units."
- [Ravi & Knight, 2011] Sujith Ravi and Kevin Knight, "Deciphering foreign language."
- [Dou & Knight, 2012] Qing Dou and Kevin Knight, "Large scale decipherment for out-of-domain machine translation."
- [Johnson et al. 2017] Melvin Johnson,et al, "Google’s multilingual neural machine translation system: Enabling zero-shot translation."
- [Zhang et al. 2017] Meng Zhang, Yang Liu, Huanbo Luan, and Maosong Sun. "Adversarial training for unsupervised bilingual lexicon induction"
- [ Koehn & Knowles, 2017] Philipp Koehn and Rebecca Knowles. Six challenges for neural machine translation.
- [Chen et al., 2017] Yun Chen, Yang Liu, Yong Cheng, and Victor O.K. Li. A teacher-student framework for zero-resource neural machine translation.